1. 前言¶
1.1. 版权声明¶
以下条款适用于所有访问燧原产品和服务相关文档的用户或阅读者(以下统称“您”),本文档包括本文档提供的信息属于上海燧原科技股份有限公司和/或其子公司(以下统称“燧原”)或其许可方所有,且燧原保留不经通知随时对本文档信息或对本文档所述任何产品和服务做出修改的权利。本文档所含信息和本文档所引用燧原其他信息均“按原样”提供。燧原不担保信息、文本、图案、链接或本文档内所含其他项目的准确性或完整性。燧原不对本文档所述产品的可销售性、所有权、不侵犯知识产权、准确性、完整性、稳定性或特定用途适用性做任何暗示担保、保证。燧原可不经通知随时对本文档或本文档所述产品或服务做出更改,但不承诺因此更新本文档。
在任何情况下,燧原不对因使用或未使用本文档而导致的任何损害(包括但不限于利润损失、业务中断和信息损失等损害)承担任何责任。除非另行书面同意,燧原不对本文档承担任何责任,不论该责任因任何原因而产生或基于任何侵权理论。
本文档所列的规格参数、性能数据和等级基于特定芯片或计算机系统或组件测试所得。经该等测试,本文档所示结果反映了燧原产品在上述测试环境中的性能表现。测试系统配置及软硬件版本、环境变量等的任何变化都会影响产品或服务的实际性能,如产品或服务的实际效果与本文档描述存在差异的,均属正常现象。燧原不担保测试本文档中每种产品或服务的所有参数的准确性和稳定性。您自行承担对本文档中产品或服务是否适合并适用于您计划的应用进行评估以及进行必要测试的责任。您的使用环境、系统配置、产品设计等特性可能会影响燧原产品或服务的质量和可靠性并导致超出本文档范围的额外或不同的情况和/或要求,燧原对此不做任何担保或承担任何责任。
燧原®、Enflame ®和本文档中显示的其他所有商标、标志是上海燧原科技股份有限公司或其许可方申请和/或注册的商标。本文档并未明示或暗示地授予您任何专利、版权、商标、集成电路布图设计、商业秘密或任何其他燧原或其许可方知识产权的权利或许可。
本文档为燧原或其许可方版权所有并受全世界版权法律和条约条款的保护。未经燧原或其许可方的事先书面许可,任何人不可以任何方式复制、修改、出版、上传、发布、传输或分发本文档。为免疑义,除了允许您按照本文档要求使用本文档相关信息外,燧原或其许可方不授予其他任何明示或暗示的权利或许可。
本文档可能保留有与第三方网站或网址的链接,访问这些链接将由您自己作出决定,燧原并不保证这些链接上所提供的任何信息、数据、观点、图片、陈述或建议的准确性、完整性、充分性和可靠性。燧原提供这些链接仅仅在于提供方便,并不表示燧原对这些信息的认可和推荐,也不是用于宣传或广告目的。
您同意在使用本文档及其内容时,遵守国家法律法规、社会公共道德。您不得利用本文档及其内容从事制作、查阅、复制和传播任何违法、侵犯他人权益等扰乱社会秩序、破坏社会稳定的行为,亦不得利用本文档及其内容从事任何危害或试图危害计算机系统及网络安全的活动。
您同意,与您访问或使用本文档相关的所有事项,应根据中华人民共和国法律解释、理解和管辖。您同意,中国上海市有管辖权的法院具有相关的管辖权。
燧原对本文档享有最终解释权。
1.2. 专有名词解释¶
术语 |
定义 |
---|---|
GCU |
General Computing Unit 燧原科技通用计算单元 |
gcu300 |
燧原科技面向数据中心的第三代人工智能推理加速卡 |
SIP |
Scalable Intelligent Processor,芯片计算核心 |
Cluster |
SIP的集群分组 |
H2H |
Host to Host,数据从主存中加载,GCU运行完保存回主存 |
D2D |
Device to Device,数据放在GCU中直接运行,不写回主存 |
engine |
TopsInference在GCU上的可执行对象 |
1.3. 手册简介¶
本手册的主要目的是介绍TopsInference软件栈基础API的调用方法,并提供大量参考用例,读者可以通过本手册快速上手TopsInference推理栈。
版本更新¶
文档版本 |
文档日期 |
文档说明 |
---|---|---|
V1.0 |
2022-01-17 |
初版发布 |
V2.0 |
2022-11-18 |
修改结构,增加动态形状,混合精度,runV2等新特性 |
V2.1 |
2023-03-29 |
增加编译选项,完善使用说明 |
V2.2 |
2023-06-02 |
增加Cluster模式说明及性能评估说明 |
V2.3 |
2023-06-27 |
增加INT8推理,refit,以及用户自定义算子说明 |
V2.4 |
2023-08-18 |
更新完善编译选项说明 |
V2.5 |
2023-10-18 |
更新标量操作方法,完善使用说明 |
V3.1 |
2024-06-19 |
增加I/O数据格式支持,增加IO关于HBM用量控制策略 |
v1.0 2022.01.17
初版发布,介绍TopsInference的基本使用方法。
v2.0 2022.11.18
增加了混合精度和runV2等接口,增加完善了代码示例和安装调试方法;解决了动态形状推理,异步操作等问题。
v2.1 2023.03.29
增加编译配置选项,完善使用说明,添加常见问题解答。
v2.2 2023.06.02
增加Cluster模式说明,及性能评估说明。
v2.3 2023.06.27
增加INT8推理,refit,以及用户自定义算子说明,在H2H模式下,run/run_with_batch/runV2的output最终获取类型由list[list]调整为list[numpy.array]。
v2.4 2023.08.18
更新完善编译选项说明。
v2.5 2023.10.18
更新标量操作方法;支持无卡编译;支持loop,if,LSTM,RNN等动态控制流算子;完善refit功能,支持weight自动预处理,用户可用onnx层面的weights进行refit。
v3.1 2024-06-19
增加I/O数据格式,适用于模型输入输出不包含N维度信息或者N维度不在index=0位置; python新增api set_io_dimension_info,c++新增api setIODimensionInfo
增加IO关于HBM用量控制策略,适用于模型调优或者模型HBM有严格要求;python新增api max_workspace_size,c++新增api setMaxWorkspaceSize,getMaxWorkspaceSize
TopsInference是什么?¶
TopsInference是燧原科技提供给用户在GCU硬件平台上的推理加速引擎。 TopsInference通过读取onnx格式模型,将深度学习框架(TensorFlow, PyTorch, Caffe等)训练好的模型进行模型结构解析,优化,重组达到推理加速目的。 通过TopsInference,可以将深度学习框架训练好的模型,在GCU硬件产品中进行推理加速。
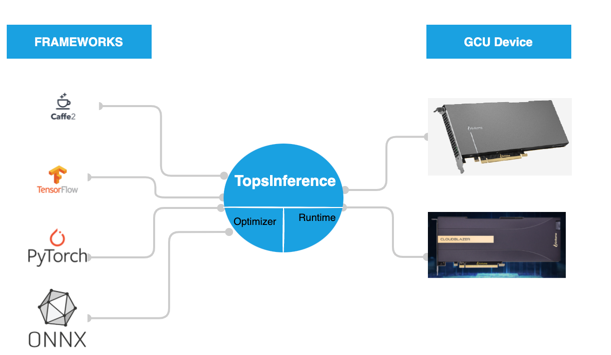
图 1.3.1 TopsInference整体示例图¶
主要功能¶
TopsInference的主要功能有:
支持 ONNX 模型图的解析
支持推理执行期间获取推理模型图输入输出形状和数据类型
支持推理模型图 FP32 到 FP16 和 INT8 的自动量化,在保证结果精度同时提高推理运算速度
支持推理模型图编译融合优化
支持动态形状模型的推理
支持同步异步,多batch,多任务,D2D等复杂场景使用
支持权重更新
支持用户自定义算子
TopsInference相比于竞品优势有:
简单易用: TopsInference 提供了 Python 和 C++ 高度封装的API接口,用户可以快速的构建起推理服务,无需关注底层和硬件细节。
丰富的模型支持: 支持市场上大部分模型,并持续扩充中。
执行流程¶
TopsInference的执行流程非常简单,如图所示。TopsInference可以将ONNX模型通过解析器Parser进行解析成Network,之后通过优化器Optimizer生成TopsInference可执行对象engine,使用engine对模型进行推理。
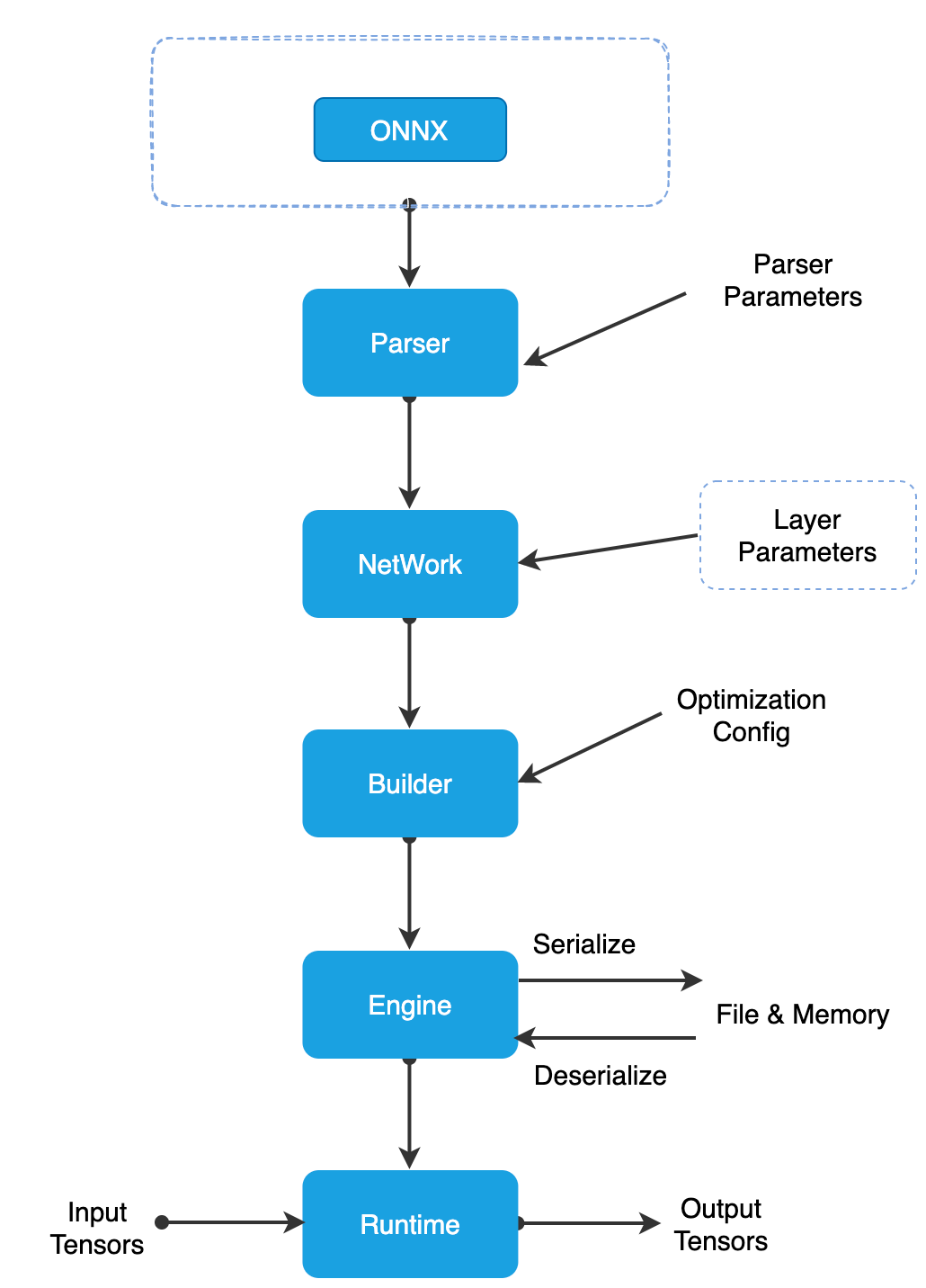
图 1.3.2 TopsInference执行流程图¶
2. 安装使用说明¶
TopsInference的安装过程请参考《TopsRider软件栈安装手册》,完成TopsRider即完成了TopsInference的安装。
Attention
TopsInference依赖于tops-sdk和topsruntime包,在安装TopsInference之前一定要确保安装好正确版本的tops-sdk和topsruntime包,否则TopsInference无法正常运行。
TopsInference的Python包依赖于TopsInference C++库,请在安装完C++包之后再安装Python包。
查看Python的TopsInference版本号命令为:
import TopsInference
print(TopsInference.__version__)
TopsInference依赖numpy>=1.18.5。
查看C++的TopsInference版本号命令为:
readelf -a ./libTopsInference.so | grep SONAME
3. 快速入门¶
TopsInference的推理流程简单易用,快速上手使用TopsInference进行模型推理示例如下:
3.1. Python模型推理示例¶
Python模型推理的核心步骤为创建解析器parser读取模型,创建优化器optimizer生成engine,最后执行推理。engine也可以先保存到磁盘,运行时直接加载即可推理。
import TopsInference
# 创建GCU分配设备的作用域
with TopsInference.device(0, 0):
# 读取模型
onnx_parser = TopsInference.create_parser(TopsInference.ONNX_MODEL)
network = onnx_parser.read("/path/to/your/model/")
# 生成engine
optimizer = TopsInference.create_optimizer()
engine = optimizer.build(network)
# 可以先将engine保存到磁盘,使用时直接加载即可
# engine.save_executable("/path/to/engine")
# engine = TopsInference.load("/path/to/engine")
# 执行推理
outputs = []
future = engine.runV2([img])
outputs = future.get()
Python使用TopsInference只需要加载 import TopsInference。
3.2. C++模型推理示例¶
C++和Python一样,首先创建解析器parser读取模型,之后创建优化器optimizer生成engine,最后执行推理。engine也可以先保存到磁盘,运行时直接加载即可推理。
和Python代码的区别为:topsInference_init是初始化topsInference,在进程开始时调用一次,topsInference_finish代表topsInference结束使用;需要配置输入输出的ITensor。用户要保证handler,parser,network,optimizer的生命周期。
#include <stdio.h>
#include <iostream>
#include <vector>
#include "TopsInference/TopsInferRuntime.h"
void TEST() {
// 初始化TopsInference
TopsInference::topsInference_init();
// 创建GCU分配设备的作用域
uint32_t cluster_id[] = {0};
auto handler = TopsInference::set_device(0, cluster_id);
// 读取模型并生成engine
TopsInference::IParser* parser = TopsInference::create_parser(TopsInference::TIF_ONNX);
TopsInference::INetwork* network = nullptr;
network = parser->readModel("/path/to/your/model/");
TopsInference::IOptimizer* optimizer = TopsInference::create_optimizer();
TopsInference::IEngine* engine = optimizer->build(network);
// 可以先将engine保存到磁盘,使用时直接加载即可
// engine->saveExecutable("/path/to/engine");
// engine->loadExecutable("/path/to/engine");
std::vector<std::vector<float>> outs;
std::vector<std::vector<float>> inputs_guad;
...
// 设置输入ITensor
float* inputs[] = {inputs_guad[t].data()};
std::vector<TopsInference::TensorPtr_t> input_tensor_list;
int32_t input_num = engine->getInputNum();
for (size_t i = 0; i < input_num; i++) {
TopsInference::TensorPtr_t sub_input = TopsInference::create_tensor();
// 需要设置输入ITensor的数据
sub_input->setOpaque(reinterpret_cast<void*>(inputs[i]));
// 需要设置输入ITensor的DeviceType
sub_input->setDeviceType(TopsInference::DataDeviceType::HOST);
TopsInference::Dims input_shape = engine->getInputShape(i);
input_shape.dimension[0] = batch_size;
// 需要使用设置输入ITensor的dims
sub_input->setDims(input_shape);
input_tensor_list.emplace_back((TopsInference::TensorPtr_t)sub_input);
}
// 设置输出ITensor
std::vector<TopsInference::TensorPtr_t> output_tensor_list;
int32_t output_num = engine->getOutputNum();
for (size_t i = 0; i < output_num; ++i) {
TopsInference::Dims max_shape = engine->getMaxOutputShape(i);
int64_t element_size = 1;
max_shape.dimension[0] = batch_size;
for (size_t j = 0; j < max_shape.nbDims; ++j) {
element_size *= max_shape.dimension[j];
}
std::vector<float> out(element_size);
outs[t] = out;
TopsInference::TensorPtr_t sub_output = TopsInference::create_tensor();
// 需要设置输出ITensor的数据
sub_output->setOpaque(reinterpret_cast<void*>(out.data()));
// 需要使用maxoutputshape设置输出ITensor的dims,设置为最大输出形状
sub_output->setDims(max_shape);
// 需要设置输出ITensor的DeviceType
sub_output->setDeviceType(TopsInference::DataDeviceType::HOST);
output_tensor_list.emplace_back((TopsInference::TensorPtr_t)sub_output);
}
// 调用runV2,执行推理
engine->runV2(input_tensor_list.data(), output_tensor_list.data());
// 获取输出outs
...
// 释放资源
for (size_t i = 0; i < input_num; ++i) {
TopsInference::destroy_tensor(input_tensor_list[i]);
}
for (size_t i = 0; i < output_num; ++i) {
TopsInference::destroy_tensor(output_tensor_list[i]);
}
output_tensor_list.clear();
input_tensor_list.clear();
inputs_guad.clear();
std::vector<std::vector<float>>().swap(inputs_guad);
outs.clear();
std::vector<std::vector<float>>().swap(outs);
TopsInference::release_parser(parser);
TopsInference::release_network(network);
TopsInference::release_optimizer(optimizer);
TopsInference::release_engine(engine);
TopsInference::release_device(handler);
TopsInference::topsInference_finish();
}
C++使用TopsInference只需要引用 #include “TopsInference/TopsInferRuntime.h” 。
TopsInference各组件的细节及进阶应用方法请查阅下一章 用户使用说明。
4. 用户使用说明¶
本章讲解TopsInference的详细使用方法,涵盖了不同应用场景的调度配置方法,前四节是使用TopsInference的基本步骤讲解,后面是进阶教程和示例。
如需要更为详尽的API参考信息,Python参见《TopsInference Python API参考手册》,C++参见《TopsInference C++ API参考手册》。
4.1. 关于set_device接口¶
基本功能¶
set_device是用来绑定硬件资源,TopsInference只有绑定了GCU资源才可以进行模型推理。
Python示例:
# 创建GCU分配设备的作用域,并声明生成engine所使用的板卡和Cluster
# 第一个参数为板卡ID,第二个参数为Cluster ID
with TopsInference.device(0, 0) as device:
C++示例:
uint32_t cluster_id[] = {0};
// 第一个参数为板卡ID,第二个参数为Cluster ID
auto handler = TopsInference::set_device(0, cluster_id);
C++的接口为:
handler_t set_device(uint32_t card_id,
const uint32_t *cluster_ids,
uint32_t cluster_ids_size=1,
IErrorManager *error_manager=nullptr);
card_id: 设备卡的id;cluster_ids: 通过设置id序列来选取卡内的Cluster;cluster_ids_size: 用来描述cluster_ids的元素个数。
通过TopsInference.device()方法来创建一个TopsInference Device句柄,并在句柄中声明卡id和cluster_id。使用gcu300时,一张卡中包含的Cluster个数与resource_mode编译选项有关(参见 resource_mode )。默认配置下(resource_mode=”1c12s”),有2个Cluster,cluster_id可以为一个0~1的列表,或一个大于等于0且小于等于1的整型数。
单进程中若没有通过TopsInference.release_device()的方法释放资源时,不能重复通过TopsInference.set_device()来分配推理资源。
不同情况下的作用域¶
i: 多线程下,每个线程都设置set_device(0, [1], 1),则每个线程独占一个Cluster,如下图:
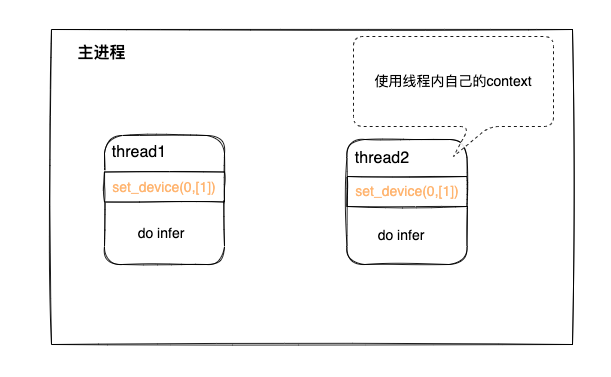
图 4.1.1 TopsInference作用域示例图1¶
ii: 只在进程中设置set_device(0, [0], 1),线程间不设置set_device,那么线程间共享这个资源,如下图:
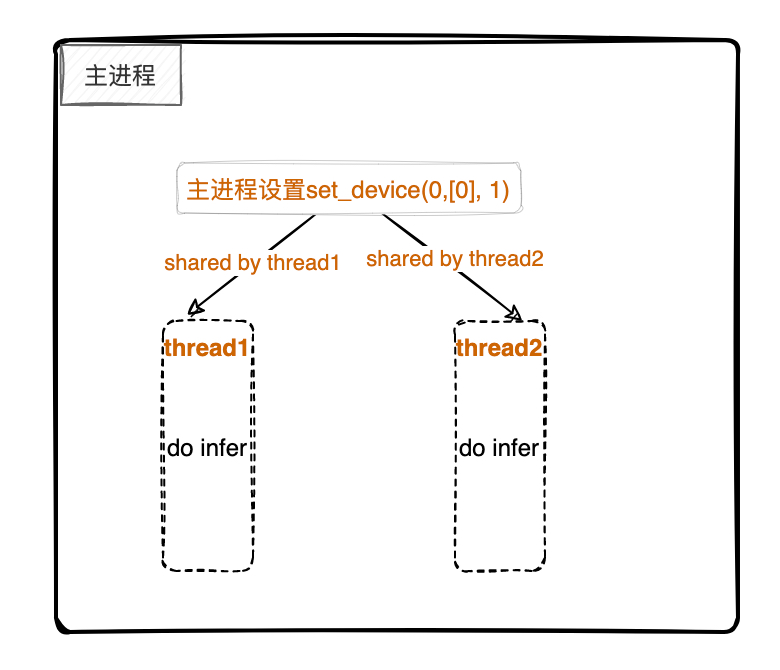
图 4.1.2 TopsInference作用域示例图2¶
iii:进程中设置了set_device(0, [0], 1) 但是每个线程都设置set_device(0, [0], 1),则每个线程依旧使用线程内Cluster,如下图:
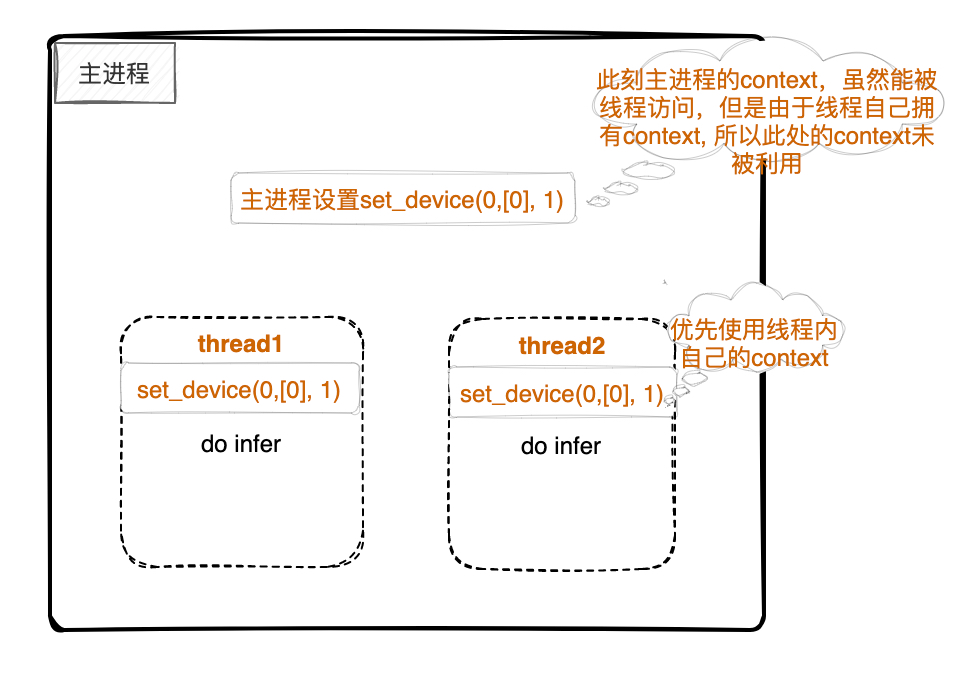
图 4.1.3 TopsInference作用域示例图3¶
iv:只在进程中设置set_device(xxx, 0),有的线程设置了set_device,那么设置set_device的线程独占分配的资源,没设置的共享进程中的资源。
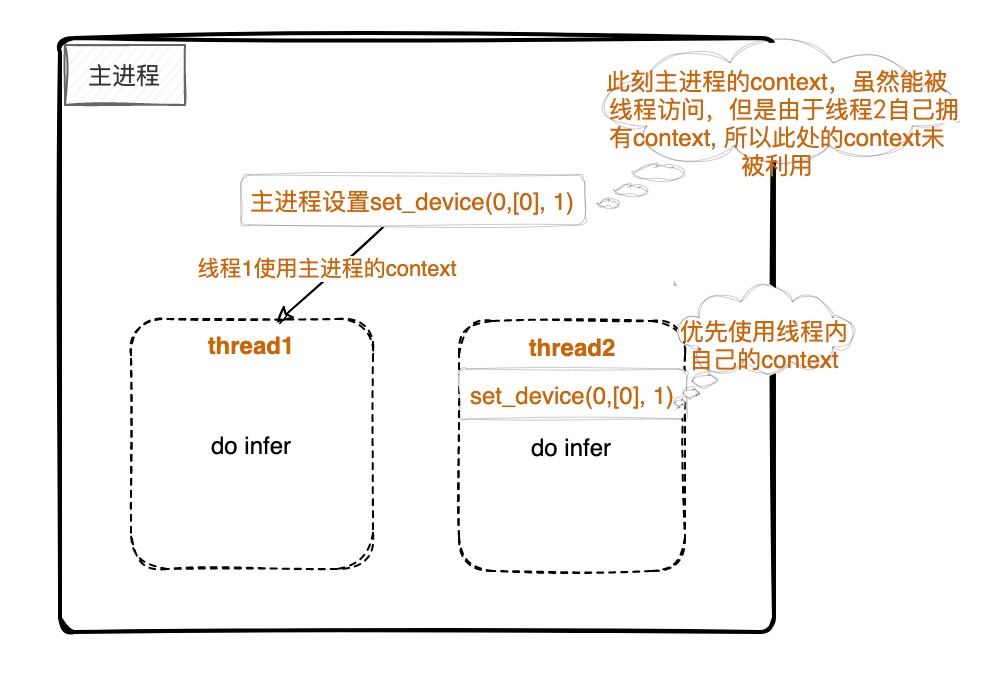
图 4.1.4 TopsInference作用域示例图4¶
Attention
同一线程下,只允许一次set_device,当然你可以将set_device的handle释放之后,再次set_device。
def test_model_multi_thread_with_onecontxt(self):
# 开启单Cluster运行
handler = TopsInference.set_device(0, 0)
thread_managers = []
thread_nums = 6
for th_id in range(thread_nums):
thread_managers.append(Thread(target=inference))
thread_managers[th_id].start()
for th_id in range(thread_nums):
thread_managers[th_id].join()
TopsInference.release_device(handler)
关于set_device(0, [-1], 1)的含义:
Python环境下,set_device(0, -1)表示的是资源占满的含义(注意,只有在gcu300卡存在该模式)
set_device(0, -1) 等价于 set_device(0, [0, 1])
C++环境下,set_device(0, [-1], 1)表示的是资源占满的含义(注意,只有在gcu300卡存在该模式)
set_device(0, [-1], 1) 等价于 set_device(0, [0, 1], 2)
延长绑定Cluster¶
当我们在set_device后,是真的已经绑定了Cluster了吗 ?
答案是否定的,比如set_device(0, [0, 1], 2)之后,TopsInference并未立刻去绑定和独占两个Cluster,而是推迟到第一个engine创建时; 这时TopsInference认为可能马上需要用到Cluster了,才开始依据set_device的相关信息去绑定并独占相应数量的Cluster。
4.2. 读取模型¶
TopsInference支持ONNX模型格式,若使用其他模型格式,可以通过开源工具转换为ONNX格式后再使用;
例如:TensorFlow PB格式,通过tf2onnx工具可以将其转换为ONNX格式。
Attention
推荐使用ONNX Opset 13
读取模型方式¶
从文件中读取¶
TopsInference需要通过创建解析器parser对模型进行读取:对应ONNX模型,对于Python需要设置parser类型为TopsInference.ONNX_MODEL,对于C++需要设置parser类型为TopsInference::TIF_ONNX。TopsInference支持的算子参见 《ONNX算子支持列表》 。
在读取模型前,可以对解析器parser配置模型的input/output name,input shape,input/output dtype,该操作会覆盖ONNX文件自己的设定,output name配置后可对模型进行子图截取;如果未进行设置,则使用ONNX模型自身定义的参数。
ONNX模型的读取支持图和权重参数保存在一个文件中,也支持分开保存,权重参数统一或独立保存成文件,这些文件要放在和ONNX同一目录之下。
下面展示了Python和C++两种方式读取ONNX模型。
Python读取示例:
# 创建ONNX解析器
onnx_parser = TopsInference.create_parser(TopsInference.ONNX_MODEL)
onnx_parser.set_input_names(["761"])
onnx_parser.set_input_shapes([[399, 256]])
onnx_parser.set_input_dtypes([TopsInference.TIF_FLOAT32])
onnx_parser.set_output_names(["779"])
# 读取ONNX模型
network = onnx_parser.read("/path/to/your/model/")
Python接口中:
set_input_names是设置输入层名称;
set_input_shapes是设置输入层的形状,输入形状数值需为大于等于0的整数(支持形状维度值为0),如果为动态形状维度,需要设置为-1;
set_input_dtypes是设置输入层的数据类型;
set_output_names是设置输出层名称;
set_output_dtypes是设置输出层的数据类型。
上述set_input_names,set_input_shapes,set_input_dtypes接口的参数映射关系需要保持一致。
set_input_shapes中的参数配置方法如下:
set_input_shapes([[1, 2, 3, 4]])
set_input_shapes([[1, 2, 3, 4], [5, 6, 7, 8]])
# 如果输入包含标量scalar输入,也就是不包含shape,使用None表示
set_input_shapes([[1, 2, 3, 4], None])
set_input_names和set_output_names配置方法如下:
set_input_names(['a'])
set_input_names(['a', 'b'])
set_input_dtypes和set_output_dtypes配置方法如下:
set_input_dtypes([TopsInference.TIF_FLOAT32])
set_input_dtypes([TopsInference.TIF_FLOAT32, TopsInference.TIF_FLOAT32])
C++读取示例:
// 创建ONNX解释器
TopsInference::IParser* parser = TopsInference::create_parser(TopsInference::TIF_ONNX);
parser->setInputNames("input");
parser->setInputShapes("1,224,224,3");
parser->setOutputNames("resnet_v1_50/predictions/Reshape_1");
// 读取ONNX模型
TopsInference::INetwork* network = nullptr;
network = parser->readModel(onnx_model_file.c_str());
C++中setInputNames是设置输入层名称,setOutputNames是设置输出层名称,setInputDtypes是设置输入各层的数据类型,setOutputDtypes是设置输出各层的数据类型,setInputShapes是设置输入各层的形状,配置中仅可以使用字符串作为输入,多个输入之间用“,”及“:”分隔。
当前TopsInference支持的数据类型有:
TIF_BOOL
TIF_INDEX
TIF_INT8
TIF_INT16
TIF_INT32
TIF_INT64
TIF_UINT8
TIF_UINT16
TIF_UINT32
TIF_UINT64
TIF_FP16
TIF_FP32
TIF_BF16
TIF_TUPLE
在Python和C++中,读取模型得到的network,可以通过network.dump()获得模型在TopsInference的中间表示,为有特殊使用需求用户开放。
从内存中读取¶
从内存中读取ONNX模型,对于Python要求parser类型为TopsInference.ONNX_MODEL,对于C++要求输入类型为onnx::ModelProto,parser类型为TopsInference::TIF_ONNX。
下面展示了Python和C++两种方式读取。
Python读取示例:
# 加载ONNX文件
onnx_model = onnx.load("/path/to/your/model/")
# 序列化
model_data = onnx_model.SerializeToString()
# 创建ONNX解析器
onnx_parser = TopsInference.create_parser(TopsInference.ONNX_MODEL)
# 读取ONNX模型
network = onnx_parser.read_from_str(model_data, len(model_data))
C++读取示例:
// 读取文件
onnx::ModelProto graphdef;
std::ifstream in(onnx_model_file, std::ios::in | std::ios_base::binary);
graphdef.ParseFromIstream(&in);
std::string graph_data;
graphdef.SerializeToString(&graph_data);
// 创建parser
TopsInference::IParser* parser = TopsInference::create_parser(TopsInference::TIF_ONNX);
TopsInference::INetwork* network = nullptr;
// 注意protobuf序列化之后存在'\0'行为,对于'\0',
// string复制存在安全隐患,一定要按照此处这种方式书写
network = parser->readModelFromStr(graph_data.c_str(), graph_data.length());
读取含有标量的模型¶
TopsInference在加载模型时,如果模型中输入(input)中存在标量scalar类型,前端语言是python时,在Parser中的set_input_shapes方法中,该处的形状设置为None。
Python 生成示例:
# 创建解析器
onnx_parser = TopsInference.create_parser(TopsInference.ONNX_MODEL)
onnx_parser.set_input_names(["133", "360"])
# 指定输入,设定形状,如下
onnx_parser.set_input_shapes([[1,224,224,3], None]) # 第二个输入为标量
onnx_parser.set_input_dtypes([TopsInference.TIF_FLOAT32, TopsInference.TIF_FLOAT32])
# 读取模型
network = onnx_parser.read("/path/to/your/model/")
如果前端语言是c++,那么在Parser中的set_input_shapes方法中,该处的形状设置为”_”即可:
C++ 生成示例:
// 创建解析器
TopsInference::IParser* parser = TopsInference::create_parser(model_type);
// 指定输入的名称
parser->setInputNames("133,360");
// 为输入指定shape,shape之间用冒号“:”分割,shape内以“,”隔开,如"1,224,224,3"
// 第二个输入为标量,那么直接设定为“_”
std::string input_shape = std::to_string(BATCH_NUM) + ",224,224,3:_";
parser->setInputShapes(input_shape.c_str());
parser->setOutputNames("output@0");
TopsInference::INetwork* network = nullptr;
network = parser->readModel("/path/to/your/model/");
读取动态形状模型¶
TopsInference支持具有动态形状模型的解析,在读取初始化过程中指定模型的动态输入维度为-1。
我们可以通过使用解析器Parser中的set_input_shapes方法来设置动态形状(ONNX模型中对应动态维度已设置为-1可以不用设置),同时在之后生成engine时必须调用set_max_shape_range和set_min_shape_range,设置好模型的max-shape和min-shape。
# 创建解析器
onnx_parser = TopsInference.create_parser(TopsInference.ONNX_MODEL)
# 为动态形状模型指定输入,设定形状,动态形状的维度设置为-1
onnx_parser.set_input_names(["133", "360"])
onnx_parser.set_input_shapes([[1, -1, -1, 64], [1, -1, -1, 128]])
onnx_parser.set_input_dtypes([TopsInference.TIF_FLOAT32, TopsInference.TIF_FLOAT32])
# 读取模型
network = onnx_parser.read("/path/to/your/model/")
4.3. 生成engine¶
以上一节读取到的模型作为输入,本章展示TopsInference如何生成推理所要使用的engine。生成engine是指创建优化器optimizer并使用优化器将读取进来的模型进行优化,生成TopsInference可执行对象engine。
engine是TopsInference的可执行对象,包含TopsInference优化后的指令权重值,之后的生成、导入、导出和推理,都是使用的这个engine对象。
生成engine方式¶
下面展示了Python和C++两种方式生成engine。
Python生成示例:
# 创建优化器
optimizer = TopsInference.create_optimizer()
# 根据读取到的ONNX模型,使用优化器生成推理engine
engine = optimizer.build(network)
C++生成示例:
// 创建优化器
TopsInference::IOptimizer* optimizer = TopsInference::create_optimizer();
// 根据读取到的ONNX模型,使用优化器生成推理engine
TopsInference::IEngine* engine = optimizer->build(network);
在编译生成engine时,可以配置推理精度,该操作为可选项。set_build_flag可以配置模型FP32,FP16,FP32与FP16混精或FP32与INT8混精,同时也可以配置每一单独层的精度,详细配置方法参考本手册 混合精度推理。
Python中engine提供了多个接口,其中 get_input_num()、get_output_num() 获取输入输出数量; get_max_input_shape(index)、 get_min_input_shape(index) 获取动态形状下最大最小输入形状信息;get_max_output_shape(index)获取动态形状下最大输出形状; get_input_shape(index)、get_output_shape(index)获取输入输出的形状信息,如果为动态形状,则获取最大形状;get_input_dtype(index)、get_output_dtype(index)获取输入输出的类型信息; get_input_name(index)、get_output_name(index)获取输入输出的层名称;get_device_memory_size()预估推理时一个engine所会占用的最大GCU内存,多cluster同一个engine下实际占用会更大,但不一定和使用的cluster数量成比例关系。
C++中engine提供了getInputNum()、getOutputNum()获取输入输出数量;getMaxInputShape(index)、getMinInputShape(index)获取动态形状下最大最小输入形状信息;getMaxOutputShape(index)获取动态形状下最大输出形状; getInputShape(index)、getOutputShape(index)获取输入输出的形状信息,如果为动态形状,则获取最大形状;getInputDtype(index)、getOutputDtype(index)获取输入输出的类型信息; getInputName(index)、getOutputName(index)获取输入输出的层名称;getDeviceMemorySize()预估推理时一个engine所会占用的最大GCU内存,多cluster同一个engine下实际占用会更大,但不一定和使用的cluster数量成比例关系。
TopsInference默认已支持loop、if、LSTM、RNN等动态控制流算子,这是在开启Host compile模式下生效,环境变量ENABLE_HOST_COMPILE需要配置为True(当前默认为True,用户无需配置)。
编译动态形状模型¶
TopsInference为支持动态形状模型,在生成engine时必须调用set_max_shape_range和set_min_shape_range,设置好模型输入的最大和最小形状,同时注意在上一节 读取模型 时要设置输入形状对应的动态维度为-1。当输入为多个,其中一些输入为静态时,静态输入的最小形状和最大形状也必须设置,并且要和设置的输出形状保持一致。
Attention
使用set_max_shape_range和set_min_shape_range时,参数输入为json格式,且根节点为key是“main”的字典,其value应为每个输入的形状为一个元素的列表。真实运行中的形状和最大最小形状的关系必须满足:最小形状≤真实形状≤最大形状,否则会报参数配置错误,最小形状的数值必须大于等于0。
比如形状为[N, C, H, W],H和W为动态维度,那么要满足:
[N, C, H-min, W-min] ≤ [N, C, H-real, W-real] ≤ [N, C, H-max, W-max]
用户在使用关键字为“main”的字典配置过模型的输入最大最小形状后,也可以配置模型中节点的最大最小形状,这时,就需要将关键字写为ONNX中节点的名称,如果用户对TopsInference足够了解,也可以使用network.dump()后,将关键字设置为中间表示里的节点名称。
Python生成示例:
# 创建优化器
optimizer = TopsInference.create_optimizer()
# 配置输入最大形状max-shape
max_shape_dim_setting = []
max_shape_dim = {}
max_shape_dim["main"] = [[1,1024,1024,64], [1,512,512,128]]
max_shape_dim_setting.append(max_shape_dim)
optimizer.set_max_shape_range(max_shape_dim_setting)
# 配置输入最小形状min-shape
min_shape_dim_setting = []
min_shape_dim = {}
min_shape_dim["main"] = [[1,16,16,64], [1,8,8,128]]
min_shape_dim_setting.append(min_shape_dim)
optimizer.set_min_shape_range(min_shape_dim_setting)
# 根据读取到的ONNX模型,使用优化器生成推理engine
engine = optimizer.build(network)
Python设置最大形状和最小形状的接口为set_max_shape_range和set_min_shape_range。
C++生成示例:
// 创建优化器
TopsInference::IOptimizer* optimizer = TopsInference::create_optimizer();
TopsInference::IOptimizerConfig* optimizer_config = optimizer->getConfig();
// 配置输入最大形状max-shape
Json::Value max_shape_range_setting;
Json::Value op_max_val;
op_max_val["main"].append("1,1024,1024,64");
op_max_val["main"].append("1,512,512,128");
max_shape_range_setting.append(op_max_val);
std::string max_setting_str = max_shape_range_setting.toStyledString();
optimizer_config->setMaxShapeRange(max_setting_str.c_str());
// 配置输入最小形状min-shape
Json::Value min_shape_range_setting;
Json::Value op_min_val;
op_min_val["main"].append("1,16,16,64");
op_min_val["main"].append("1,8,8,128");
min_shape_range_setting.append(op_min_val);
std::string min_setting_str = min_shape_range_setting.toStyledString();
optimizer_config->setMinShapeRange(min_setting_str.c_str());
// 根据读取到的ONNX模型,使用优化器生成推理engine
TopsInference::IEngine* engine = optimizer->build(network);
C++对应的接口分别为setMaxShapeRange和setMinShapeRange,参数输入为json string,且根节点为key是“main”的字典,其value应为每个输入的形状为一个元素的列表。
根节点的key是“main”表示对整个模型输入shape所做的限制,也可对单个层限制最大和最小形状,这时就要添加一个key为层名称的字典。key需要配置为如:“resnet/Transformer/ conv2d/Conv2D”,“expand”等。
无卡编译¶
TopsInference支持无卡编译,可以在没有燧原硬件卡的条件下编译engine。环境中无需安装驱动,但是用户需要保证runtime、sdk和TopsInference安装成功。
编译时和有卡编译的区别有三点:
需要配置环境变量 export ENFLAME_TIF_ENABLE_OFFLINE_OPTIMIZE=True。
不需要set_device。
需要在compile_options设置想要编译的卡,arch中选择gcu300,用户仅可以指定一个arch,不可以多选。
Python 使用无卡编译的示例:
import os
# 1.无卡编译需要配置环境变量
os.environ["ENFLAME_TIF_ENABLE_OFFLINE_OPTIMIZE"] = "True"
import TopsInference
# 2.无卡编译不需要set_device
onnx_parser = TopsInference.create_parser(TopsInference.ONNX_MODEL)
network = onnx_parser.read("/path/to/your/onnx")
compile_options = {}
# 3.无卡编译必须选择目标卡的arch
compile_options['arch'] = 'gcu300'
optimizer = TopsInference.create_optimizer()
optimizer.set_compile_options(compile_options)
engine = optimizer.build(network)
engine.save_executable("/path/to/your/bin")
C++ 使用无卡编译的示例:
// 1.无卡编译需要设置环境变量
// export ENFLAME_TIF_ENABLE_OFFLINE_OPTIMIZE=True
#include <stdio.h>
#include <iostream>
#include <vector>
#include "TopsInference/TopsInferRuntime.h"
void TEST() {
TopsInference::topsInference_init();
// 2.无卡编译无需set_device
// uint32_t cluster_id[] = {0};
// auto handler = TopsInference::set_device(0, cluster_id);
TopsInference::IParser* parser = TopsInference::create_parser(TopsInference::TIF_ONNX);
TopsInference::INetwork* network = nullptr;
network = parser->readModel("/path/to/your/model/");
TopsInference::IOptimizer* optimizer = TopsInference::create_optimizer();
// 3.无卡编译必须选择目标卡的arch
auto config = optimizer->getConfig();
const char* compiler_options = "{\"arch\": \"gcu300\"}";
config->setCompileOptions(compiler_options);
TopsInference::IEngine* engine = optimizer->build(network);
engine->saveExecutable("/path/to/engine");
TopsInference::release_parser(parser);
TopsInference::release_network(network);
TopsInference::release_optimizer(optimizer);
TopsInference::release_engine(engine);
TopsInference::topsInference_finish();
}
无卡编译时不支持混精中INT8量化。
配置编译选项¶
TopsInference提供了功能丰富的高阶可配置编译选项,如配置禁用pass,配置融合模式,开启自动混精调试等,用户需在生成engine前进行配置。
调用set_compile_options接口,传入complier_options用于可选参数设置,complier_options由key-value键值对组成的字典表示。不支持通过环境变量等其他方式对TopsInference的编译选项进行配置。
Python 配置编译选项的示例:
optimizer = TopsInference.create_optimizer()
compile_options = {'fusion.use_nodename_match': True,
'pass.excludes': "xxx1,xxx2",
'statics.config_path': 'xxx3'}
optimizer.set_compile_options(compile_options)
c++ 配置编译选项的示例:
TopsInference::IOptimizer* optimizer = TopsInference::create_optimizer();
TopsInference::IOptimizerConfig *optimizer_config = optimizer->getConfig();
Json::Value compile_options;
compile_options["fusion.use_nodename_match"] = true;
compile_options["pass.excludes"] = "xxx1,xxx2";
compile_options["statics.config_path"] = "xxx3";
const std::string options = compile_options.toStyledString();
optimizer_config->setCompileOptions(options.c_str());
C++可以使用Json格式的字符串对compile_options进行配置,用户需引用Json库,如不使用Json库,可以直接使用const char * 来配置。
const char *compile_options = "{\"resource_mode\": \"1c12s\"}";
optimizer_config->setCompileOptions(compile_options);
以下为所有可以配置keys的编译选项及说明:
pass.excludes¶
使用该键可以配置需要禁用的pass优化,类型为string,可以配置的选项见 优化配置项 。
Python中,值为多个的时候,类型可以为string、list、set和tuple,配置方法如下:
实现方式 |
代码示例 |
---|---|
string |
|
list |
|
set |
|
tuple |
|
C++的配置方法如下:
Json::Value compile_options;
compile_options["pass.excludes"] = "xxx1,xxx2";
const std::string options = compile_options.toStyledString();
pass.includes¶
使用该键可以配置需要使能部分默认关闭的pass,某些pass在只有在特定的模型中是正向优化,因此我们默认关闭,特定模型通过此选项开启,类型为string,可以配置的选项见 优化配置项 。
Python中,值为多个的时候,类型可以为string、list、set和tuple,配置方法如下:
实现方式 |
代码示例 |
---|---|
string |
|
list |
|
set |
|
tuple |
|
C++的配置方法如下:
Json::Value compile_options;
compile_options["pass.includes"] = "xxx1,xxx2";
const std::string options = compile_options.toStyledString();
enable_op_statistics¶
使用该键可以配置算子结果统计开关,类型为bool型,默认为false。
Python的配置方法如下:
compile_options = {'enable_op_statistics': True}
C++的配置方法如下:
Json::Value compile_options;
compile_options["enable_op_statistics"] = true;
const std::string options = compile_options.toStyledString();
op_statistics.config_path¶
使用该键可以配置算子结果统计数据文件路径,类型为目录的string。
Python的配置方法如下:
compile_options = {'enable_op_statistics': True,
'op_statistics.config_path': '/home/xxx.proto'}
C++的配置方法如下:
Json::Value compile_options;
compile_options["enable_op_statistics"] = true;
compile_options["op_statistics.config_path"] = "/home/xxx.proto";
const std::string options = compile_options.toStyledString();
enable_auto_fine_tune¶
使用该键可以配置是否开启自动混精调试模式,配合topsideas混精调试工具使用,类型为bool型,默认为false。
Python的配置方法如下:
compile_options = {'enable_auto_fine_tune': True}
C++的配置方法如下:
Json::Value compile_options;
compile_options["enable_auto_fine_tune"] = true;
const std::string options = compile_options.toStyledString();
precision.fp32_ops¶
使用该键可以配置指定id的算子在混精计算中以FP32精度运算,类型为int或int的list或string。
配置算子的id是由topsideas混精调试工具计算得出的整型结果,请配合topsideas工具一起使用。
Python的配置方法如下:
compile_options = {'precision.fp32_ops': 3}
# list
compile_options = {'precision.fp32_ops': [1, 2, 3]}
C++的配置方法如下:
Json::Value compile_options;
compile_options["precision.fp32_ops"] = 3;
# string
compile_options["precision.fp32_ops"] = "1,2,3";
const std::string options = compile_options.toStyledString();
enable_gcu_only¶
使用该键可以配置是否仅使用GCU进行计算,类型为bool型,默认为false,表示算子可以在GCU/CPU上混用,true表示禁用CPU核心,仅可以在GCU上运算。
Python的配置方法如下:
compile_options = {'enable_gcu_only': True}
C++的配置方法如下:
Json::Value compile_options;
compile_options["enable_gcu_only"] = true;
const std::string options = compile_options.toStyledString();
gcu_only.check_excludes¶
当enable_gcu_only为true时,使用该键可以配置当找不到GCU算子时,可以选择在CPU中计算的算子,类型为string。
Python中,值为多个的时候,类型可以为string、list、set和tuple,配置方法如下:
实现方式 |
代码示例 |
---|---|
string |
|
list |
|
set |
|
tuple |
|
C++的配置方法如下:
Json::Value compile_options;
compile_options["enable_gcu_only"] = true;
compile_options["gcu_only.check_excludes"] = "xxx1,xxx2";
const std::string options = compile_options.toStyledString();
enable_fusion¶
使用该键可以配置是否开启算子融合,类型为bool型,默认为true,表示开启。
2.3.0版本后弃用,默认开启,可使用’fusion.excludes’: [“all_fusion”]替代 ‘enable_fusion’: False。
Python的配置方法如下:
compile_options = {'enable_fusion': False}
C++的配置方法如下:
Json::Value compile_options;
compile_options["enable_fusion"] = false;
const std::string options = compile_options.toStyledString();
fusion.includes¶
使用该键可以配置指定算子进行融合,类型为string。可以配置的选项见 优化配置项 。
Python中,值为多个的时候,类型可以为string、list、set和tuple,配置方法如下:
实现方式 |
代码示例 |
---|---|
string |
|
list |
|
set |
|
tuple |
|
C++的配置方法如下:
Json::Value compile_options;
compile_options["fusion.includes"] = "xxx1, xxx2";
const std::string options = compile_options.toStyledString();
fusion.excludes¶
使用该键可以配置禁用指定算子融合,类型为string。可以配置的选项见 优化配置项 。
如果想禁用所有算子融合,需要配置为’fusion.excludes’: [“all_fusion”]。
Python中,值为多个的时候,类型可以为string、list、set和tuple,配置方法如下:
实现方式 |
代码示例 |
---|---|
string |
|
list |
|
set |
|
tuple |
|
C++的配置方法如下:
Json::Value compile_options;
compile_options["fusion.excludes"] = "xxx1,xxx2";
const std::string options = compile_options.toStyledString();
Attention
TopsInference中,默认所有fusion全部开启;配置fusion.includes时,只有指定fusion才生效;配置fusion.excludes时,只有指定fusion被禁用;如果同时配置fusion.includes和fusion.excludes,仅fusion.includes配置生效,fusion.excludes配置无效。
fusion.use_nodename_match¶
使用该键可以配置是否使用节点名称进行匹配,类型为bool型,默认为true。
Python的配置方法如下:
compile_options = {'fusion.use_nodename_match': False}
C++的配置方法如下:
Json::Value compile_options;
compile_options["fusion.use_nodename_match"] = false;
const std::string options = compile_options.toStyledString();
resource_mode¶
使用该键可以配置Cluster计算资源数量,仅可以在gcu300卡上配置,类型为string,包含一种选项:
1个Cluster对应12个SIP计算资源: “1c12s”
默认使用“1c12s”。
Python的配置方法如下:
compile_options = {'resource_mode': '1c12s'}
C++的配置方法如下:
Json::Value compile_options;
compile_options["resource_mode"] = "1c12s";
const std::string options = compile_options.toStyledString();
max_dim_size¶
使用该键可以配置输出张量单个维度的最大尺寸,用于动态形状模型编译,默认为 32×1024×1024,类型为int或int的list。
Python的配置方法如下:
compile_options = {'max_dim_size': 20000}
C++的配置方法如下:
Json::Value compile_options;
compile_options["max_dim_size"] = 1000;
const std::string options = compile_options.toStyledString();
loop_shape_inference¶
使用该键可以配置启动循环形状推理,直到形状不再变化或循环次数达到指定值。此选项主要针对一些复杂动态模型,需要多次形状推理才能得出最优形状。默认为0,类型为int。
compile_options = {'loop_shape_inference': 1}
C++的配置方法如下:
Json::Value compile_options;
compile_options["loop_shape_inference"] = 2;
const std::string options = compile_options.toStyledString();
attention_engine¶
使用该键可以配置对AIGC大模型提供极致融合优化,提供更高的性能表现;此选项主要针对Transformer类网络的attention结构进行优化,并覆盖其他相关衍生结构。默认为true,类型为bool型。
Python的配置方法如下:
compile_options = {'attention_engine': False}
C++的配置方法如下:
Json::Value compile_options;
compile_options["attention_engine"] = false;
const std::string options = compile_options.toStyledString();
disable_weight_preprocess¶
使用该键可以关闭对weight的预处理操作,一张卡上多个模型weight可以复用。默认为false,类型为bool型。
开启该选项后(True),同一张卡中多个模型可以复用weight,但是性能有可能会有下降,请权衡使用。
Python的配置方法如下:
compile_options = {'disable_weight_preprocess': True}
C++的配置方法如下:
Json::Value compile_options;
compile_options["disable_weight_preprocess"] = true;
const std::string options = compile_options.toStyledString();
unsafe_math_optimizations¶
使用该键可以配置一些非安全的数学优化,简化网络,默认不启用优化。当前支持enable_float_mul_0_simplify,从网络层简化乘0的操作,融合为constant 0。
Python中,值为多个的时候,类型可以为string、list、set和tuple,配置方法如下:
实现方式 |
代码示例 |
---|---|
string |
|
list |
|
set |
|
tuple |
|
Python的配置方法如下:
compile_options = {'unsafe_math_optimizations': 'enable_float_mul_0_simplify'}
C++的配置方法如下:
Json::Value compile_options;
compile_options["unsafe_math_optimizations"] = "enable_float_mul_0_simplify";
const std::string options = compile_options.toStyledString();
enable_constant_reuse¶
使用该键可以使能weight复用操作,一张卡上多个模型weight可以复用。默认为false,类型为bool型。
开启该选项后(True),同一张卡中多个模型可以复用weight,disable_weight_preprocess会配置为True,关闭所有broadcast的折叠,但是性能有可能会有下降,请权衡使用。
Python的配置方法如下:
compile_options = {'enable_constant_reuse': True}
C++的配置方法如下:
Json::Value compile_options;
compile_options["enable_constant_reuse"] = true;
const std::string options = compile_options.toStyledString();
arch¶
使用该键可以在无卡编译场景下指定硬件卡类型,可以指定“gcu200”、“gcu210”或“gcu300”,仅可以选择一种,类型为string型。
需配合环境变量ENFLAME_TIF_ENABLE_OFFLINE_OPTIMIZE使用,参考 无卡编译 。
Python的配置方法如下:
compile_options = {'arch': 'gcu300'}
C++的配置方法如下:
Json::Value compile_options;
compile_options["arch"] = 'gcu300';
const std::string options = compile_options.toStyledString();
4.4. 推理¶
在读取模型并生成engine之后,本节讲解如何进行推理。
加载engine¶
生成engine后我们已经将ONNX文件转换成了可在GCU上运行的可执行对象,可以直接执行推理,就不需要加载engine这一步骤;也可以先保存到文件或内存后再加载执行。
Python保存至文件使用save_executable接口,加载engine使用load接口,示例:
# 保存engine
engine.save_executable("/path/to/engine")
# 加载engine
engine = TopsInference.load("/path/to/engine")
Python从内存中加载engine使用load_exec_from_buffer接口,示例:
# 加载engine
engine = TopsInference.load_exec_from_buffer(blob)
C++保存至文件使用saveExecutable接口,加载engine使用loadExecutable接口,示例:
// 保存engine
engine->saveExecutable("/path/to/engine");
// 加载engine
engine->loadExecutable("/path/to/engine");
C++从内存中加载engine使用loadExecFromBuffer接口,示例:
TopsInference::IEngine* engine = TopsInference::create_engine();
uint64_t binary_size = 0;
std::vector<char> binary_data;
std::ifstream in_file(EXEC_PATH,
std::ios::in | std::ios::binary | std::ios::ate);
if (in_file.is_open()) {
binary_size = in_file.tellg();
in_file.seekg(0, std::ios::beg);
binary_data.reserve(binary_size);
in_file.read(binary_data.data(), binary_size);
in_file.close();
}
engine->loadExecFromBuffer(binary_data.data(), binary_size);
执行推理¶
一个完整的TopsInference模型推理流程可以被划分成以下四个步骤:
加载或生成engine
读取数据
为输入数据分配片上缓存空间
为输出数据分配片上缓存空间
将数据从主机拷贝至设备上指定的缓存空间中(host to device)
使用engine执行推理
获得推理结果
将推理结果从设备上指定的缓存空间中拷贝至主机上(device to host)
释放片上缓存
TopsInference在推理时提供了三种接口,其中runV2接口功能最为全面,推荐用户使用,run_with_batch接口适用于多batch的场景,run接口使用较为简便。
关于runV3接口¶
runV3接口,是runV2的一个升级版本,目的在于能够和runtime3.0 C API进行互相调用,从这个接口开始,我们会逐渐去除TopsInference的topsInferStream_t类型,开始统一使用rt3.0的TopsStream_t。 这次我们先发布了c++版本的接口,如下:
TIFStatus runV3(IN TensorPtr_t* inputs, INOUT TensorPtr_t* outputs,
topsStream_t stream = nullptr,
IFuture* future = nullptr);
和runV2的唯一不同,在于我们使用了rt3.0的topsStream_t。
关于runV2接口¶
我们提供的runV2接口覆盖更为全面的应用场景,包含静态形状和动态形状,同步和异步模式,多Batch,H2H以及D2D的场景。
Python接口中input_list为输入的list;关键字参数可以加入对应的output_list和py_stream,output_list为输出的list,py_stream默认为空,为同步模式,可以配置stream,实现异步功能;返回py_future。
def runV2(self, input_list, **kwargs):
"""
:param input_list: tensor or list
:param kwargs: key include: output_list, py_stream
if output_list is None, will allocate output_list.
:return: py_future
"""
在H2H模式下,output_list最终获取的是list[numpy.array]格式。
接口参数,如下所示:
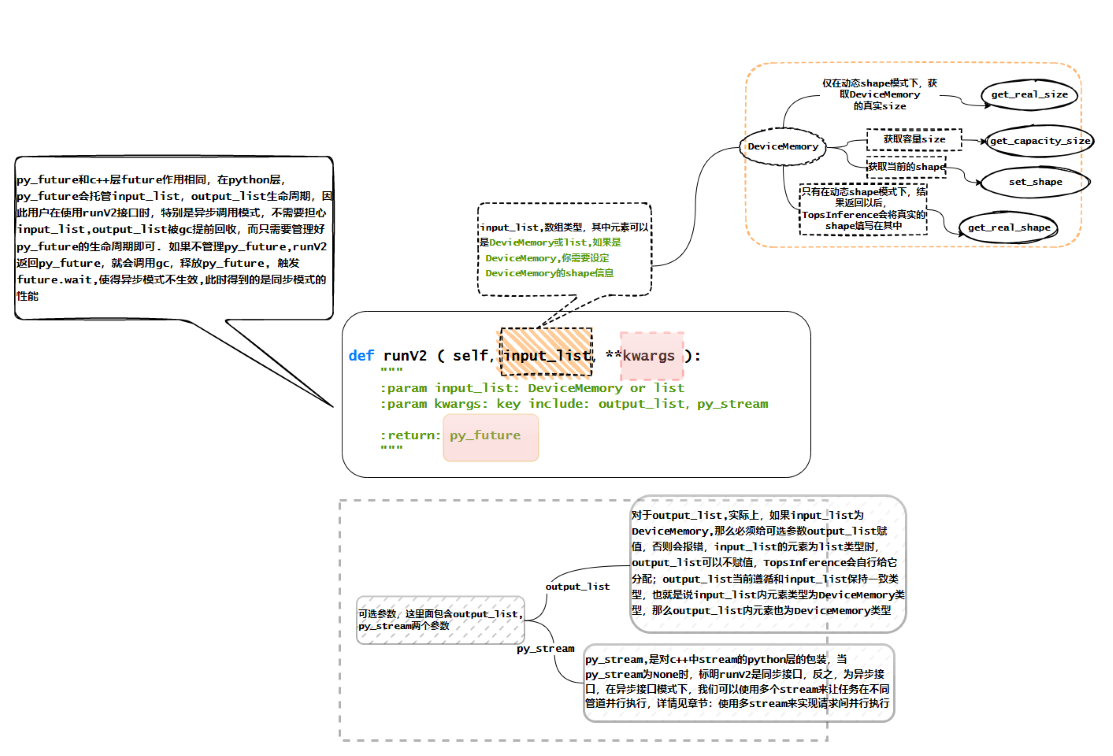
图 4.4.1 TopsInference接口参数示例图1¶
ITensor作为TopsInference数据的载体,包含数据和形状等信息。 每个输入输出都是一个独立的ITensor(一个模型可能会包含多个输入或输出),用户要对ITensor中原始数据Opaque,维度信息Dims和设备类型DeviceType三部分信息进行配置。
C++接口中inputs为所有输入的ITensor;outputs为所有输出的ITensor;stream默认值为空,代表同步模式;future默认值为空。
TIFStatus runV2(IN TensorPtr_t* inputs, INOUT TensorPtr_t* outputs,
topsInferStream_t stream = nullptr,
IFuture* future = nullptr);
接口参数,如下如所示:
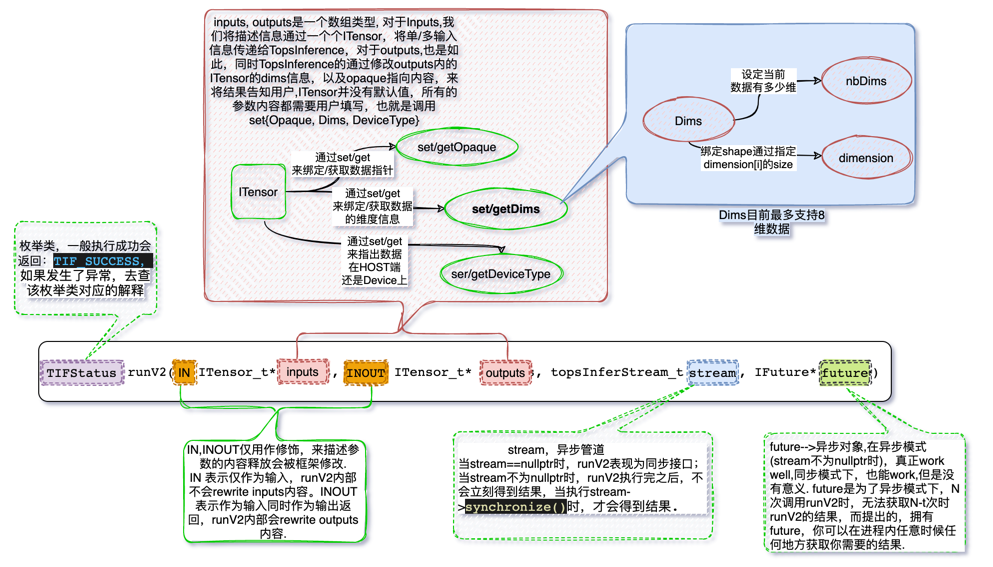
图 4.4.2 TopsInference接口参数示例图2¶
Python简单的推理过程如下:
# 生成随机输入,d0,d1,d2,d3分别对应模型input shape
input_data = np.random.randn(d0, d1, d2, d3).astype(np.float32, order='C')
output_data = []
with TopsInference.device(0, 0):
# 加载engine
engine = TopsInference.load("/path/to/engine")
# 读取数据,执行推理并返回结果
future = engine.runV2([input_data])
output_data = future.get()
至此一个简单的推理过程便完成了。
关于run_with_batch接口¶
run_with_batch对静态形状模型的多batch运行进行了优化,但是该接口不支持动态形状。
Python接口中,sample_nums为请求样本数量,input_list为输入数据,可以是tensor或者list,kwargs包括output_list、buffer_type和py_stream。
def run_with_batch(self, sample_nums, input_list, **kwargs):
"""
:param sample_nums: the number of sample
:param input_list: tensor or list
:param kwargs: key include: output_list,buffer_type,py_stream.
if output_list is None, will allocate output_list.
:return: py_future
"""
output_list = kwargs.get('output_list', [])
buffer_type = kwargs.get('buffer_type', TIF_ENGINE_RSC_IN_HOST_OUT_HOST)
py_stream = kwargs.get('py_stream', None) # default sync mode
....
Python层的run_with_batch各个参数的解释:
sample_num: 请求的样本数量,对应NCHW的第N维,和生成engine时的N不一定相等,以指定数量N多次处理,拼接为sample_num的结果,不整除时填充为0。
input_list: 可以为DeviceMemory或者Host数据。
output_list: 当使用的是IN_HOST_OUT_HOST模式时,output_list可以为空,此时,系统会自动帮你分配output_list数组,其他模式,需要用户自己来分配数据对象,无论buffer_type是哪种模式,当用户自己分配数组对象时,系统会复用这个数组(避免了分配数组空间操作,性能会更快),在H2H模式下,最终获取的结果是list[numpy.array]。
buffer_type: 使用数据模式。
py_stream: 使用stream,意味着使用异步模式。
Python层的run_with_batch的return: run_with_batch返回的结果是一个PyFuture对象。
class PyFuture(object):
def get(self):
...
def wait(self):
...
def status(self):
C++接口,其中batch_size为请求样本数量,inputs为输入数据,outputs为输出结果,buf_type为执行模式(H2H,D2D可选),stream默认为空,若为空,同步模式,future默认为空。
bool runWithBatch(std::size_t batch_size, void** inputs,
void** outputs, BufferType buf_type,
topsInferStream_t stream = nullptr, IFuture* future = nullptr) = 0;
关于batch_size(sample_num)参数的一些解释: batch_size通俗的说,就是这次请求的样本数量,这里需要和我们的构图期间的set_shape(N,C,H,W)的N区别开来,两者不是同一个概念。 构图时的set_shape(N,C,H,W)中N是每次GCU处理的数量,执行时无论提交了多少样本数量,每次在GCU里进行构图时以指定数量N处理,然后进行汇总为batch_size数量的结果。如果样本数量比每次处理的少,自动填充为0。 batch_size不需要强行等于N,可以小于也大于,当然也可以不整除。
Attention
TopsInference所有推理接口仅支持batch_size位于形状信息的第一个维度,如果有多个输入,每个输入的第一维都必须是batch_size。若无batch_size,请使用runV2接口进行模型推理。
可以通过PyFuture对象的get获取结果数据,wait 来等待执行完成, status查询任务释放执行完毕。 使用Python层的run_with_batch,你不必去管理input_list和output_list的生命周期,系统会自动管理,推荐使用返回结果PyFuture来获取output数据,在H2H模式下,output的格式为list[numpy.array]。举例如下:
# output_list 可以为空
stream = streams[c_ind]
c_ind = (c_ind + 1) % cluster_num
py_future = engine.run_with_batch(batch_size, np.array([intputs]), output_list=None,
buffer_type=TopsInference.TIF_ENGINE_RSC_IN_HOST_OUT_HOST,
py_stream=stream)
outputs_list.append(py_future)
# output_list 不为空
py_future = engine.run_with_batch(batch_size, np.array([intputs]), output_list=[],
buffer_type=TopsInference.TIF_ENGINE_RSC_IN_HOST_OUT_HOST,
py_stream=stream)
outputs_list.append(py_future)
# 同步模式 py_stream=None
py_future = engine.run_with_batch(batch_size, np.array([intputs]),
buffer_type=TopsInference.TIF_ENGINE_RSC_IN_HOST_OUT_HOST)
outputs_list.append(py_future)
# 同步模式 py_stream=None
py_future = engine.run_with_batch(batch_size, np.array([intputs]), output_list=[],
buffer_type=TopsInference.TIF_ENGINE_RSC_IN_HOST_OUT_HOST)
outputs_list.append(py_future)
Attention
run_with_batch适用于inputs中的样本数和outputs结果数,为1:1对应关系,例如: 输入为(N,C,H,W)中的N和输出中的(N,)中的N相等
关于run接口¶
TopsInference提供了最基本的run接口,使用engine进行推理的Python核心语法如下:
engine.run(input_tensor_list, output_tensor_list, resource_type, stream = None)
可以看到输入输出均为list形式,使用resource_type对输入输出的缓存类型进行区分,stream则是用于异步推理模式,这两个概念可以参考本手册 异步推理。
在H2H模式下,输出output_tensor_list最终获取的为list[numpy.array]格式。
使用engine进行推理的C++核心语法如下:
bool TopsInferenceEngineImpl::run(void **inputs, void **outputs,
BufferType buf_type,
topsInferStream_t stream);
Python简单的推理过程如下:
# 生成随机输入,d0,d1,d2,d3分别对应模型input shape
input_data = np.random.randn(d0, d1, d2, d3).astype(np.float32, order='C')
output_data = []
with TopsInference.device(0, 0):
# 加载engine
engine = TopsInference.load("/path/to/engine")
# 读取数据,执行推理并返回结果
engine.run([input_data], output_data, TopsInference.TIF_ENGINE_RSC_IN_HOST_OUT_HOST)
4.5. 性能调优¶
本节针对一些较为进阶的使用场景进行介绍,更加高效的使用硬件资源,运行复杂模型,包括如何使用TopsInference进行异步推理,多Cluster资源运行以及动态batch运行。
H2H及D2D配置¶
在推理时,必然存在一个数据由主机搬运到设备上的过程,这一过程需要申请设备上的缓存空间。因此根据设备缓存空间的申请方式不同,TopsInference划分了两种缓存处理方式,分别为:
TopsInference.TIF_ENGINE_RSC_IN_HOST_OUT_HOST:即H2H模式,输入输出的缓存空间在主机上,该模式不需要用户手动指定设备缓存空间,从而使得代码结构更加简单。
TopsInference.TIF_ENGINE_RSC_IN_DEVICE_OUT_DEVICE:即D2D模式,输出输出的缓存空间在设备(即GCU)上,该模式需要用户根据模型的输入输出大小指定设备缓存空间,这样可以在多个相关任务推理时避免缓存的重复拷贝,提高了推理效率。
用户可以跟据自己所使用的场景去选择,默认都为H2H模式。
当使用D2D模式时,需要将输入和输出数据配置为对应的DEVICE数据,并将DeviceType或buffer_type配置为D2D模式。
异步推理¶
同步方法表明调用一旦开始,调用者必须等待方法执行完成,才能继续执行后续方法。异步方法表明,方法一旦开始,立即返回,调用者无需等待其中方法执行完成,就可以继续执行后续方法。 快速入门 这一章节就是一个经典的同步模式的推理案例,因此我们在这不再赘述同步模式的推理应用。
异步推理基本方法¶
在异步推理时,我们需要引入一个新的概念——流(stream)。
所谓stream,指的是在GCU上按顺序执行的一系列操作(即输入数据搬运、数据推理、推理结果搬运等),我们可以将一个stream视为一个推理任务。同一个stream内的操作,TopsInference确保是按照加入stream的顺序进行执行的。不同stream之间,TopsInference不保证执行的顺序,和多线程类似。
TopsInference提供的runV2、run_with_batch和run都有提供异步调用模式,run接口目前仅提供了IN_DEVICE_OUT_DEVICE模式的异步, 而runV2和run_with_batch提供了IN_HOST_OUT_HOST和IN_DEVICE_OUT_DEVICE两种模式,所有情况都支持异步,本节主要以run_with_batch为例,讲述TopsInference异步编程。
在异步模式下,我们需要手动构建流:
stream = TopsInference.create_stream()
TopsInference支持同时创建不超过1000个stream,用户在使用多stream时需要及时释放不需要的资源。
如果需要使用D2D的方式,在数据搬运上有着较大区别:
# 数据搬运
TopsInference.mem_h2d_copy_async(src, dst, size, stream) # host to deivce
TopsInference.mem_d2h_copy_async(src, dst, size, stream) # device to host
由于上述两个数据拷贝是异步操作,因此为了正确取得结果,我们应该在每次推理任务结束之后同步流中的所有操作:
stream.synchronize()
Python接口如何使用run_with_batch的异步模式:
# 创建GCU分配设备的作用域
handle = TopsInference.set_device(0, 0)
# 创建GCU stream
stream = TopsInference.create_stream()
py_future = engine.run_with_batch(batch_size,
np.array([intput[0],intput[1],intput[2]]),
output_list=None,
buffer_type=TopsInference.TIF_ENGINE_RSC_IN_HOST_OUT_HOST,
py_stream=stream)
# 获取结果
results = py_future.get()
# 释放分配设备
TopsInference.release_device(handle)
C++接口如何使用runWithBatch的异步模式:
// 设备卡号
constexpr int32_t card_id = 0;
// 使用virtual core logic id
constexpr uint32_t cluster_ids[] = {0};
// 初始化TopsInference
TopsInference::topsInference_init();
// 创建GCU分配设备的作用域
auto* handler = TopsInference::set_device(card_id, cluster_ids, /*cluster_ids_size*/ 1, nullptr);
// 创建stream
TopsInference::topsInferStream_t stream;
TopsInference::create_stream(&stream);
std::vector<std::vector<float>> outs;
std::vector<IFuture*> futures;
...
for (size_t t = 0; t < steps; t++) {
float* inputs[] = {inputs_guard[t].data()};
handle->inputs_buffer = reinterpret_cast<void**>(inputs);
float* outputs[] = {outs[t].data()};
futures.emplace_back(TopsInference::create_future());
engine->runWithBatch(batch_list[t], handle->inputs_buffer,
reinterpret_cast<void**>(outputs),
TopsInference::BufferType::TIF_ENGINE_RSC_IN_HOST_OUT_HOST,
stream, futures[t]);
}
...
for (size_t t = 0; t < steps; t++) {
if (futures[t].wait()) {
uint32_t output_idx = argsortMax(outs[t]);
}
}
// 释放资源
TopsInference::destroy_stream(stream);
TopsInference::release_device(handler);
Attention
为保障性能最优,建议在构建好engine之后,再创建stream。
同时,使用future也可以达到异步的效果,在什么情况下,我们需要使用到future这个选项?
当我们在调用runWithBatch之后,不需要立刻获取output数据时(或者说异步情况下),我们可以通过这个future来保持对这次请求的output数据的状态的跟踪。
future和stream有什么区别?
首先需要说明的是,future是配合stream一起使用的,当然在没有stream的同步情况下,你依旧可以使用future,不过此时future的功能是多余的。 future在stream的情况下,可以避免调用TopsInference::synchronize_stream(stream)方法来获得output,因为此时如果stream堆积了超过1次请求的数据,而此时我们需要获取第一次请求的数据时,需要等到stream内的任务都执行完才能获取到,这会使得效率降低,并且很有可能会影响到stream的任务继续下发,而使用future可以很好的避免这一点。
# 创建GCU stream
stream = TopsInference.create_stream()
py_future1 = engine.runV2([img1], py_stream=stream)
py_future2 = engine.runV2([img2], output_list=outputs2, py_stream=stream)
py_future3 = engine.runV2([img3], py_stream=stream)
py_future4 = engine.runV2([img4], py_stream=stream)
# 获取第一运行结果,此时第2、3、4次任务并未同步运行完,只同步第一次的推理
results1 = py_future1.get()
# 同步所有任务,全部推理完才可以拿到结果outputs2
stream.synchronize()
results1 = outputs2
设置合理共享Cluster数¶
上文有提到,在同一个分配设备的作用域下(线程域或者进程域),不同的engine之间可以共享分配设备的作用域,如下图所示。
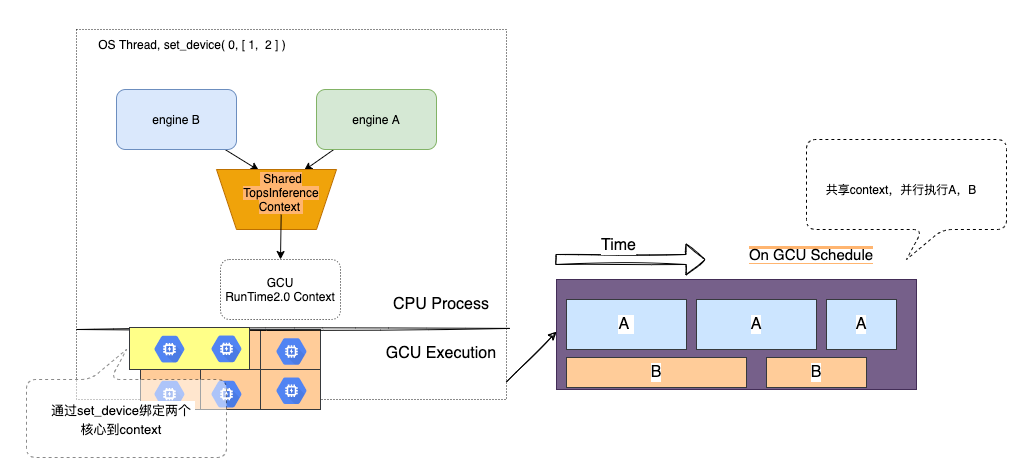
图 4.5.1 TopsInference共享设备作用域示例图1¶
此处我们讨论的是在单个engine上,当我们将多个Cluster绑定到分配设备的作用域上时(如set_device(0, [0, 1], 2),将两个Cluster绑定到分配设备的作用域上),如果使用 异步推理基本方法 的方式 调用run_with_batch接口下发的样本数sample_num, 不足以占满所有Cluster时,GCU的利用率就不会高,Python举例如下: 下面的例子,我们编译构图期使用的compiler_bs=4,但是两次请求的sample_num分别是: req1{sample_num=4},req2{sample_num=4}:
# 创建GCU分配设备的作用域,并绑定两个Cluster
handle = TopsInference.set_device(0, [0, 1])
# 创建GCU stream
stream = TopsInference.create_stream()
batch_size = 4
A1 = engine.run_with_batch(batch_size,
np.array([intput]),
output_list=None,
buffer_type=TopsInference.TIF_ENGINE_RSC_IN_HOST_OUT_HOST,
py_stream=stream)
A2 = engine.run_with_batch(batch_size,
np.array([intput]),
output_list=None,
buffer_type=TopsInference.TIF_ENGINE_RSC_IN_HOST_OUT_HOST,
py_stream=stream)
A3 = engine.run_with_batch(batch_size,
np.array([intput]),
output_list=None,
buffer_type=TopsInference.TIF_ENGINE_RSC_IN_HOST_OUT_HOST,
py_stream=stream)
# 获取结果
results.append(A1.get())
results.append(A2.get())
results.append(A3.get())
# 同步一下stream,确保stream上所有任务均执行完
stream.synchronize()
# 释放作用域
TopsInference.release_device(handle)
上述代码,执行如下图所示:
上图所示,每一个时刻,在同一个Stream上只有一个任务(请求)在占用分配设备的作用域内的Cluster,但是由于一个任务的sample_num=4, 在一个Cluster上执行就能满足需求,此时就存在一个Cluster浪费的情况,相对于作用域中的资源利用率就只有50%, 那么要想不浪费Cluster,我们需要更大的sample_num 至少大于 (作用域分配设备数-1)*compiler_bs=4,Python举例如下:
# 创建GCU分配设备的作用域, 并绑定两个Cluster
handle = TopsInference.set_device(0, [0, 1])
# 创建GCU stream
stream = TopsInference.create_stream()
# sample_num > (作用域分配设备数-1)*compiler_bs
batch_size = 7
A1 = engine.run_with_batch(sample_nums=batch_size,
input_list=np.array([intput[0],intput[1],intput[2]]),
output_list=None,
buffer_type=TopsInference.TIF_ENGINE_RSC_IN_HOST_OUT_HOST,
py_stream=stream)
A2 = engine.run_with_batch(sample_nums=batch_size,
input_list=np.array([intput[0],intput[1],intput[2]]),
output_list=None,
buffer_type=TopsInference.TIF_ENGINE_RSC_IN_HOST_OUT_HOST,
py_stream=stream)
A3 = engine.run_with_batch(sample_nums=batch_size,
input_list=np.array([intput[0],intput[1],intput[2]]),
output_list=None,
buffer_type=TopsInference.TIF_ENGINE_RSC_IN_HOST_OUT_HOST,
py_stream=stream)
# 获取结果
results.append(A1.get())
results.append(A2.get())
results.append(A3.get())
# 同步一下stream,确保stream上所有任务均执行完
stream.synchronize()
# 释放作用域
TopsInference.release_device(handle)
上述代码,执行如下图所示:
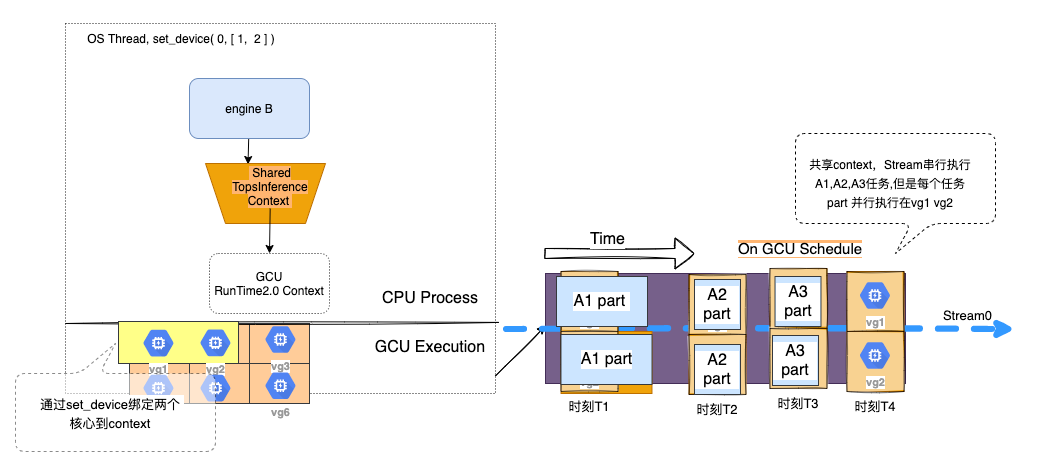
图 4.5.2 TopsInference共享设备作用域示例图3¶
如上图,大的sample_num > (作用域分配设备数-1)*compiler_bs场景下,一个Cluster已经跑不下这么多数据,那么TopsInference在使用run_with_batch会自动帮你分配给下一个Cluster,A1任务被拆分成两个part,分别同时执行在Cluster1, Cluster2上。 通过这种方式,一个任务(请求)便能并行执行在两个Cluster上,这需要用户自行分配数据。使用runV2时想要最大化使用Cluster硬件资源,需要用户自行分配数据,参考下一节 多Stream并行。
多Stream并行¶
在上一节,我们介绍了使用大sample_num可以达到请求内并行执行,来提升分配设备的作用域绑定资源内的利用率。 这节我们打算提供更便捷的提升分配设备作用域资源利用率的方式—多stream模式或者称为和Cluster数量相等的stream模式,Python示例:
# 创建GCU分配设备的作用域, 并绑定两个Cluster
handle = TopsInference.set_device(0, [0, 1])
# 创建GCU stream A 和 B
stream_A = TopsInference.create_stream()
stream_B = TopsInference.create_stream()
batch_size = 4
future_A = engine.run_with_batch(sample_nums=batch_size,
input_list=np.array([intput[0],intput[1],intput[2]]),
output_list=None,
buffer_type=TopsInference.TIF_ENGINE_RSC_IN_HOST_OUT_HOST,
py_stream=stream_A)
future_B = engine.run_with_batch(sample_nums=batch_size,
input_list=np.array([intput[0],intput[1],intput[2]]),
output_list=None,
buffer_type=TopsInference.TIF_ENGINE_RSC_IN_HOST_OUT_HOST,
py_stream=stream_B)
# 获取结果
results.append(future_A.get())
results.append(future_B.get())
# 同步一下stream,确保stream上所有任务均执行完
stream.synchronize()
# 释放作用域
TopsInference.release_device(handle)
上述代码,执行如下图所示:

图 4.5.3 TopsInference多stream并行示例图¶
当有两个stream时,stream间共享分配设备的作用域并行执行各自的任务,而stream内串行有序执行自有任务。 这种模式不需要用户分配数据,便可以在同一时刻,实现请求间分配设备的作用域绑定的Cluster资源共享。
动态batch推理¶
动态batch推理是指用户可以使用run_with_batch接口,显示指定任意大小的样本数batch_size, 该方法会通过enqueue操作自动将输入沿着第0轴(即batch方向)数据拆分或者合并成N个部分,这N部分数据存入到队列并同时被一个拥有M个Cluster的资源池中进行并发推理,并且推理完成后自动拼接结果。
对于密集请求,动态的batch_size, run_with_batch能够帮助你自动请求合并,例如:req0{batch_size=3,}, req1{batch_size=5,},在适当的时间内(在从入队列到出队列之前的过程中,会自动合并),帮你变成两个batch_size=4的请求, 可以节约一次run时间,当然这是针对静态构图的bs=4时而言。
在使用runV2推理动态形状模型时,同时想使用多batch,可以结合多stream用法,创建多个异步runV2进行推理,能够有效利用硬件资源。注意,runV2使用多batch时也要保证第一维的batch size在最大形状和最小形状之间。
计算图优化¶
TopsInference支持丰富的编译时计算图优化手段。通过对计算图进行优化,可以达到以下目标:
提高计算速度:通过减少计算量、优化计算顺序、并行计算等方式,减少计算图的执行时间,提高计算速度。这对于需要快速处理大规模数据或实时响应的应用非常重要。
减少资源占用:通过减少内存占用、优化数据传输、合并操作等方式,降低计算图对硬件资源(如内存、带宽)的需求,减少资源占用。这有助于提高系统的整体效率和可扩展性。
提高能效:通过优化计算图的执行方式,减少不必要的计算和数据传输,以及最大程度地利用硬件并行性和缓存等特性,提高能效。这对于减少资源消耗非常重要。
支持特定硬件:通过针对特定硬件平台进行优化,利用硬件的特性和优化技术,充分发挥硬件的潜力,提高计算图在特定硬件上的执行效率和性能。
支持特定应用场景:针对特定的应用场景和需求,进行计算图优化,以满足应用的实时性、精度、资源限制等方面的需求。
优化分类¶
当前主要支持如下2个方面的优化:
1.设备无关优化
完成与硬件无关、算法级别的优化,包括常量折叠、冗余分支消除等:
线性代数简化:除了利用矩阵乘法结合律之外,还可以利用分配律、转置性质等线性代数相关性质进行表达式简化。例如,将矩阵乘法转换为矩阵-向量乘法或矩阵-矩阵乘法,以减少计算量和内存访问次数。
常量折叠:识别计算图中可以在编译期进行提前计算的常量表达式,例如常数相加、乘法等,将其替换为计算结果。这样可以减少运行时阶段的计算量,提高执行效率。
形状推断(Shape Inference):通过在编译期进行形状推导,可以确定计算图中各个操作的输出形状,并提前分配内存空间。这样可以避免在运行时动态分配内存的开销,提高执行效率。
量化:对于精度要求不高的张量,可以使用低精度数据表示,例如使用8位整数或浮点数代替32位浮点数。这样可以减少设备内存的占用,降低对DMA(直接内存访问)带宽的影响,并加快计算速度。
图剪枝:识别和移除计算图中不必要的计算分支或无用的节点。这样可以减少计算量和内存占用,并简化计算图的结构。
内存重用:识别计算图中可以共享和重用的中间结果,减少重复计算和数据传输。
2.设备相关优化
完成硬件相关的优化,包括物理布局转换、前后融合等:
Layout转换:根据硬件的要求,转换张量在内存中的布局,使其更适合硬件内核的访问方式。例如,将张量的存储顺序从行主序(row-major)转换为列主序(column-major),或者进行数据重排,以最大程度地利用硬件的数据缓存和访问模式,提高访问效率。
图算融合(fusion):将计算图中可以融合的多个算子组合成单个内核执行。这样可以减少内存访问和内核启动的开销,提高计算效率。例如,将连续的卷积、批归一化和激活函数操作合并为一个内核执行。
内核选择:根据算子的实际支持情况和硬件特性,选择性能最优的内核。不同的硬件平台可能支持不同的内核实现,例如CPU、GPU或专用的加速器。选择最适合目标硬件的内核可以提高计算效率和性能。
这些设备无关和设备相关的优化技术可以相互结合,根据具体的计算图、硬件平台和性能需求进行选择和调整,以达到最佳的计算图执行效率和性能。
优化配置项¶
用户想要调整TopsInference提供的优化编译选项,可以参考 配置编译选项 进行配置。
3.1版本gcu300支持的fusion pattern list如下,默认全部开启,用户可以指定部分开启或部分关闭:
ConvBiasActivation
ConvBiasQuantActivation
DotgBiasActivation
ReduceMean
Argmax
TopKSelect
SwishFusion
GluFusion
EncoderMaskFusion
BroadcastSelectSoftmaxBroadcastSelectFusion
MaskPadSliceFusion
FcTopK
ConvBiasTranspose
Generalffn
Generalmsa
ConvModuleGeneral
ResidualLNConvModuleGeneral
TransposeConvBias
ReduceSign
ElementwiseAddReluFusion
AfeStraight
AfeAggregate
AfeGeneral
AfeTupleOutput
AfeSingleNode
TlfFmhaMulSoftmax
AfeFmhcaMulSoftmax
AfeFmhaMulSoftmax
TlfTransConvElem
TlfElemTrans
TlfGegluDotLayernorm
Ocr2MHSA
Ocr2MHSABni
Reduce_H
Reduce_W
AddQuantActivation
ExpandOutputShape
PadConvBiasOutput
TensorLoopFusionWrap
TensorLoopFusion
DafeGeneral
TransposeBNIDotOutput
Ocr2InputHWShape
ConvBiasActivationSplitFusion
inter_dot_gemm
DynamicAttention
DotFusion
ConvFusion
SwiGLU
topsopGatherLastTokenLogits
topsopRearrangeAfterAllGather
topsvllmFusedAddRmsNorm
GeneralSoftmaxFusion
gcu300可支持禁止的优化pass如下:
ConstantFolding
CustomFusionPass
BroadcastFoldingPass
内存优化¶
在H2H模式推理时,TopsInference在GCU侧会自动复用输入和输出的内存,分配输入和输出中较大尺寸的设备内存,减少GCU设备的内存占用,此优化不需要用户进行操作。
用户在使用D2D模式GCU内存资源紧张时,也可以使用同一片内存同时作为网络的输入和输出。
用户在C++中使用H2H模式时,可以使用Runtime的接口topsHostMalloc分配pin memory来防止内存分配到swap空间,导致数据拷贝影响推理时间。
C++配置示例:
void* ptr = nullptr;
// D2H和H2D的时间会更短
ret = topsHostMalloc(&ptr, maxSize * getTypeSize(dataType));
4.6. 混合精度推理¶
TopsInference已支持多种不同精度的模型推理,包含FP32、FP16、FP16与FP32混精推理,INT8与FP32混精推理。通过配置精度,以极小的精度损失换取数倍的性能提升,精度方案由用户根据具体应用场景自行选配。
设置模型精度¶
在很多情况下,为了得到更高推理性能,我们可以通过降低模型精度的方法来实现这一目标。在TopsInference推理栈中,获得低精度engine的方法十分简单,只需要使用优化器Optimizer提供的set_build_flag来指定需要的数据精度即可。
目前TopsInference推理栈支持4种精度模式:
- ONNX原始默认精度模式:
Python为TopsInference.KDEFAULT
C++为TopsInference::BuildFlag::TIF_KTYPE_DEFAULT
- INT8与FP32的混合精度:
Python为TopsInference.KINT8_FP32_MIX
C++为TopsInference::BuildFlag::TIF_KTYPE_INT8_MIX_FP32
- FP16与FP32的混合精度:
Python为TopsInference.KFP16_MIX
C++为TopsInference::BuildFlag::TIF_KTYPE_MIX_FP16
- FP16精度:
Python为TopsInference.KFP16
C++为TopsInference::BuildFlag::TIF_KTYPE_FLOAT16
TopsInference仅支持原始ONNX为FP32精度进行混合精度量化,不支持已经量化为FP16/INT8再使用TopsInference的混精推理,如ONNX已进行了量化或混精处理,请使用默认KDEFAULT模式。
将模型精度配置为FP16和FP32混合精度,具体代码样例如下:
Python配置示例:
optimizer = TopsInference.create_optimizer()
# 指定模型推理为FP16和FP32混合精度
optimizer.set_build_flag(TopsInference.KFP16_MIX)
C++配置示例:
TopsInference::IOptimizer* optimizer = TopsInference::create_optimizer();
TopsInference::IOptimizerConfig* optimizer_config = optimizer->getConfig();
// 指定模型推理为FP16和FP32混合精度
optimizer_config->setBuildFlag(TopsInference::BuildFlag::TIF_KTYPE_MIX_FP16);
针对单个/某类型layer设置精度¶
设置layer精度的操作仅可在FP16与FP32的混合精度模式、INT8与FP32的混合精度模式下生效。
FP16与FP32的混合精度模式中,设置layer精度支持如下两种配置:
1)FP32精度:TopsInference.TIF_FP32;
2)F16精度:TopsInference.TIF_FP16;
FP16与FP32的混合精度模式下,对精度敏感的少数类型的layer使用FP32计算,其他layer默认使用FP16精度计算。
但是可通过layer name或layer type来设置某一个layer或某种类型的layer的计算精度为FP32,这样做的主要目的是降低该layer对最终精度的影响。
Layer Type |
Which Layer |
---|---|
TIF_DECONVOLUTION |
Deconvolution layer. |
TIF_CONVOLUTION |
Convolution layer. |
TIF_UNARY |
UnaryOp operation layer. |
TIF_TRANSCENDENTAL |
Transcendental layer. |
TIF_ELEMENTWISE |
Elementwise operation layer. |
TIF_SELECT |
Select layer. |
TIF_POOLING |
Pooling layer. |
TIF_BATCHNORM |
Batch normalization layer. |
TIF_CONVERT |
Convert layer for converting between different data precision. |
TIF_CONCAT |
Concat layer. |
TIF_CONSTANT |
Constant layer. |
TIF_SHUFFLE |
Shuffle layer. |
TIF_ACTIVATION |
Activation layer. |
TIF_ORDER |
Layer for sorting by a certain rule. |
TIF_RNN |
Rnn layer. |
TIF_GATHER |
Gather layer. |
TIF_MATMUL |
Matmul layer. |
TIF_COMPARE |
Compare layer. |
TIF_CONDITION |
Condition layer. |
TIF_NMS |
Non max suppression layer. |
TIF_PAD |
Padding layer. |
TIF_RANDOM |
Random generator layer. |
TIF_REDUCE |
Reduce layer. |
TIF_RESHAPE |
Reshape layer. |
TIF_RESIZE |
Resize layer. |
TIF_ROIALIGN |
ROI align layer. |
TIF_SCATTER |
Scatter layer. |
TIF_SLICE |
Slice layer. |
TIF_TOPK |
TopK layer. |
TIF_TRANSPOSE |
Transpose layer. |
TIF_MVN |
Mean-variance normalization layer. |
TIF_SOFTMAX |
Softmax layer. |
TIF_LOG_SOFTMAX |
Log Softmax layer. |
TIF_SAMPLE |
Sample layer. |
TIF_DEQUANTIZE |
Dequantize layer. |
TIF_CUMSUM |
Cumsum layer. |
TIF_DFT |
DTF(discrete Fourier transform) layer. |
TIF_DET |
DET layer. |
TIF_EINSUM |
einsum layer. |
TIF_LOOP |
loop layer. |
TIF_MELWEIGHT |
mel weight matrix layer. |
TIF_OPTIONAL |
optinal serise op layer. |
TIF_QUANTIZE |
Quantize layer. |
TIF_SEQUENCE |
sequence serise op layer. |
TIF_STFT |
STFT(Short-time Fourier Transform) layer. |
TIF_TFIDF |
TFIDF layer. |
TIF_TRILU |
TRILU(triangular) layer. |
TIF_STRINGNORM |
STRINGNORM layer. |
TIF_EXPAND |
Expand layer. |
TIF_CUSTOM |
Customcall layer. |
TIF_NORMLIZE |
Normlization layer. |
Python设置layer精度示例:
onnx_parser = TopsInference.create_parser(TopsInference.ONNX_MODEL)
network = onnx_parser.read("/path/to/your/model/")
layers_num = network.get_layer_num()
# 通过算子名称设置精度
layers_name = ['resnetv17_stage1_relu1_fwd', 'resnetv17_pool1_fwd']
# 通过算子类型设置精度
layers_type = [TopsInference.TIF_CONVOLUTION, TopsInference.TIF_ELEMENTWISE]
for index in range(layers_num):
layer = network.get_layer_by_index(index)
layer_name = layer.get_name()
layer_type = layer.get_type()
if (layer_name in layers_name) or (layer_type in layers_type):
layer.set_precision(TopsInference.TIF_FP32)
C++设置layer精度示例:
TopsInference::INetwork* network = nullptr;
network = parser->readModel("/path/to/your/model/");
// 逐层单层设置精度
int32_t layers_num = network->getLayerNum();
for (size_t index = 0; index < layers_num; index++) {
auto layer = network->getLayer(index);
auto layer_type = layer->getType();
if (TopsInference::LayerType::TIF_CONVOLUTION == layer_type) {
layer->setPrecision(TopsInference::DataType::TIF_FP16);
}
}
INT8与FP32的混合精度模式中,设置layer精度支持如下两种配置:
1)FP32精度:TopsInference.TIF_FP32;
2)INT8精度:TopsInference.TIF_INT8;
INT8与FP32的混合精度模式下,以下ONNX OP类型的layer默认使用INT8精度计算:
Conv
Add
Sub
Mul
Concatenate
Relu
AveragePool
GlobalAveragePool
MaxPool
Gemm
INT8量化校准器配置¶
INT8与FP32的混合精度模式中,是使用的训练后量化(Post-training quantization,PTQ)方式,必须要配置对应的量化校准器, 基于原始模型和用户配置的输入样本数据计算得到数据的分布范围,选择不同的量化粒度。
无卡编译时不能使用INT8量化功能。
当前支持4种校准器算法,分别为:
KL_ENTROPY 对应校准器类为:IInt8EntropyCalibrator。
MAX_MIN 对应校准器类为:IInt8MaxMinCalibrator。
MAX_MIN_EMA 对应校准器类为:IInt8MaxMinEMACalibrator。
PERCENTILE 对应校准器类为:IInt8PercentCalibrator。
以KL_ENTROPY算法举例,用户需要去继承IInt8EntropyCalibrator类,其中必须实现的是getBatchSize和getbatch函数,getBatchSize是获取每次校准的batch size,getbatch是获取样本数据。
Important
校准器类中的getBatchSize()返回的值会影响engine推理数据的batch size。
举例: getBatchSize()返回值为2,parser在解析onnx模型的时候,如果调用set_input_shape(),指定N为8,那最终engine推理的时候,输入batch size为2*8=16。
getBatchSize()返回值为4,parser在解析onnx模型的时候,如果未调用set_input_shape(),则采用onnx模型默认的batch size(假设默认值为1),那最终engine推理的时候,输入batch size为4*1=4。
Python实现IInt8EntropyCalibrator类示例代码如下:
class MyCalibrator(tif.IInt8EntropyCalibrator):
def __init__(self, batch_size, data_path, cache_file="onnx_resnet50.calibrator"):
super().__init__()
self.max_batch_size = 500
self.cache_file = cache_file
self.batch_size = batch_size
self.current_index = 0
self.num_inputs = 0
self.data = []
with codecs.open(data_path, mode="r", encoding="utf8") as fr:
for filename in fr:
self.data.append("../../" + filename.strip())
self.num_inputs = min(len(self.data), self.max_batch_size)
logger.info("total img_num: {}\m".format(self.num_inputs))
def get_batch_size(self):
return self.batch_size
def get_batch(self, names):
if self.current_index + self.batch_size > self.num_inputs:
logger.info("Calibrating index {:} batch size {:} exceed max input limit {:} sentences".format(
self.current_index, self.batch_size, self.num_inputs))
# need to be reset to 0 for next call
self.current_index = 0
return [] # return [] or None
current_batch = int(self.current_index / self.batch_size)
if current_batch % 10 == 0:
logger.info("Calibrating batch {:}, containing {:} sentences".format(
current_batch, self.batch_size))
# get a batch
features = []
for i in range(self.batch_size):
logger.info("call getBatch img_index/total_imgs: {:}/{:}, cur batch: {:} , maxBatches: {:}, model batch_size: {:}".format(
self.current_index+i+1, self.num_inputs, current_batch+1, int(self.num_inputs/self.batch_size), self.batch_size))
file_path = self.data[self.current_index + i]
# get feature
logger.info("load img: {}".format(file_path))
if (self.current_index+i) % 5 == 0:
logger.info("load img :{} +++++++++++++++ percent: {:}%".format(
file_path, float((i+self.current_index+1)*100.)/self.num_inputs))
features.append(preprocess_cv(file_path))
self.current_index += self.batch_size
return [np.concatenate(features, axis=0)]
def read_calibration_cache(self):
# If there is a cache, use it instead of calibrating again. Otherwise, implicitly return None.
# if os.path.exists(self.cache_file):
# with open(self.cache_file, "rb") as f:
# return f.read()
return None
def write_calibration_cache(self, cache):
# with open(self.cache_file, "wb") as f:
# f.write(cache)
# f.flush()
# os.fsync(f)
pass
write_calibration_cache()是将校准结果进行保存,read_calibration_cache()可以读取之前存储的校准结果,避免重复执行校准,节省时间,这两个为用户可选方法。
C++实现IInt8EntropyCalibrator类示例代码如下:
class Int8EntropyCalibrator : public TopsInference::IInt8EntropyCalibrator {
public:
explicit Int8EntropyCalibrator(const DataFlowParams& data_params);
virtual ~Int8EntropyCalibrator();
int getBatchSize() const noexcept override { return data_params_.batchSize; }
bool getBatch(TopsInference::TensorPtr_t bindings[], const char* names[],
int num) noexcept override;
const void* readCalibrationCache(int64_t& length) override;
bool writeCalibrationCache(const void* ptr, int64_t length) override;
private:
friend InferModel;
std::size_t batch_index_;
DataFlowParams data_params_;
std::size_t input_size_;
std::vector<std::string> img_paths_;
std::vector<float> h_inputs_;
std::vector<char> calibrationCache;
};
Int8EntropyCalibrator::Int8EntropyCalibrator(const DataFlowParams& data_params)
: data_params_(data_params) {
batch_index_ = data_params_.imageIndex +
(data_params_.batchSize - 1) / data_params_.batchSize;
int inputChannel = INPUT_C;
int inputH = INPUT_H;
int inputW = INPUT_W;
this->input_size_ = data_params_.batchSize * inputChannel * inputH * inputW;
std::fstream f(data_params.imgListFilePath, std::ios_base::in);
if (f.is_open()) {
std::string temp;
while (std::getline(f, temp)) img_paths_.push_back(temp);
}
h_inputs_.resize(this->input_size_);
}
Int8EntropyCalibrator::~Int8EntropyCalibrator() {}
bool Int8EntropyCalibrator::getBatch(TopsInference::TensorPtr_t bindings[],
const char* names[],
int nbBindings) noexcept {
if (batch_index_ > data_params_.maxBatches ||
data_params_.imageIndex + data_params_.batchSize >
static_cast<int>(img_paths_.size())) {
return false;
}
float* h_inputs_block = h_inputs_.data();
std::size_t img_ind_st = data_params_.imageIndex;
std::size_t img_ind_ed = data_params_.imageIndex + data_params_.batchSize;
for (size_t j = img_ind_st; j < img_ind_ed; ++j) {
if (j % 5 == 0 || j == img_ind_ed) {
std::cout << "load img percent: " << img_paths_[j]
<< " +++++++++++++++: " << (j + 1) * 100. / img_paths_.size()
<< "%" << std::endl;
}
cv::Mat img = cv::imread(img_paths_[j]);
std::vector<float> inputData = prepareImage(img);
if (inputData.size() != input_size_) {
std::cerr << "input shape error" << std::endl;
return false;
}
assert(inputData.size() == input_size_);
std::memcpy(h_inputs_block, inputData.data(),
static_cast<int>(inputData.size()) * sizeof(float));
h_inputs_block += inputData.size();
}
data_params_.imageIndex += data_params_.batchSize;
assert(nbBindings == 1);
TopsInference::TensorPtr_t sub_input = bindings[0];
sub_input->setOpaque(reinterpret_cast<void*>(h_inputs_.data()));
sub_input->setDeviceType(TopsInference::DataDeviceType::HOST);
TopsInference::Dims input_shape;
input_shape.nbDims = 4;
input_shape.dimension[0] = data_params_.batchSize; // N
input_shape.dimension[1] = INPUT_C; // C
input_shape.dimension[2] = INPUT_H; // H
input_shape.dimension[3] = INPUT_W; // W
sub_input->setDims(input_shape);
batch_index_++;
return true;
}
const void* Int8EntropyCalibrator::readCalibrationCache(int64_t& length) {
return nullptr;
}
bool Int8EntropyCalibrator::writeCalibrationCache(const void* cache,
int64_t length) {
return true;
}
writeCalibrationCache()是将校准结果进行保存,readCalibrationCache()可以读取之前存储的校准结果,避免重复执行校准,节省时间,这两个为用户可选方法。
使用INT8量化校准器进行配置,在生成engine时,模型由FP32量化为INT8,流程如下图:
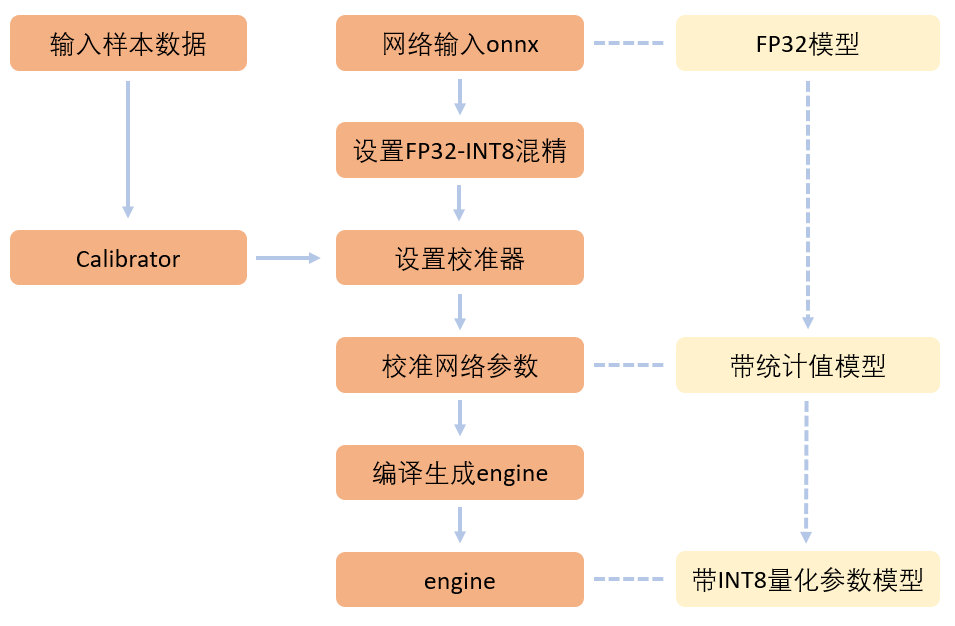
图 4.6.1 TopsInference INT8量化校准配置流程图¶
INT8量化混精模型推理¶
INT8与FP32的混合精度模式中,首先在优化器optimizer的config中配置好BuildFlag,选择INT8与FP32的混合精度模式,实现TopsInference提供的Calibrator类,配置对应的校准样本数据,并将Calibrator配置到optimizer中。 之后流程和其他方式没有区别,build生成engine后,推理即可。
Note
INT8校准过程在/tmp/gcu_calibration目录下中会生成大量中间缓存参数文件,请勿删除(校准量化结束后可以手动删除)。
Python进行混合量化模型推理代码如下,MyCalibrator见上前面 INT8量化校准器配置 Python示例代码:
onnx_parser = TopsInference.create_parser(TopsInference.ONNX_MODEL)
network = onnx_parser.read("/path/to/your/model/")
optimizer = TopsInference.create_optimizer()
optimizer.set_build_flag(TopsInference.BuildFlag.KINT8_FP32_MIX)
# 使用样本数据校准INT8量化网络
calibrator = MyCalibrator(batch_size=1, data_path="/path/to/your/data/")
optimizer.set_int8_calibrator(calibrator)
engine = optimizer.build(network)
future = engine.runV2(inputs)
C++进行混合量化模型推理代码如下,Int8EntropyCalibrator见上前面 INT8量化校准器配置 C++示例代码:
auto* parser =
TopsInference::create_parser(TopsInference::ParserType::TIF_ONNX);
auto* network = parser->readModel("/path/to/your/model/");
TopsInference::release_parser(parser);
TopsInference::IOptimizer* optimizer = TopsInference::create_optimizer();
auto config = optimizer->getConfig();
config->setBuildFlag(TopsInference::BuildFlag::TIF_KTYPE_INT8_MIX_FP32);
Int8EntropyCalibrator calibrator;
config->setInt8Calibrator(&calibrator);
TopsInference::IEngine* engine = optimizer->build(network);
// prepare input and output tensor
...
engine->runV2(input_tensor_list.data(), output_tensor_list.data());
4.7. 动态形状支持¶
runV2能够有效支持动态形状的应用场景,在生成engine时中配置好最大和最小形状后,任何满足范围的输入维度参数都可以在运行时配置。
运行动态形状时,需要配置每个输入的ITensor对应形状信息,同时将所有输出ITensor的形状配置为最大形状,运行结束后获取实际的形状信息。
Attention
动态形状模型中的最大输入形状会影响模型的推理速度以及GCU内存的占用,用户在设置时要考量实际输入范围,不要设置过大的最大输入形状。
Python动态形状推理¶
Python在使用动态形状时,主要分为五个步骤:
a.读取模型生成engine,读取模型时对应输入维度设置为-1,在engine的生成过程中要使用set_max_shape_range和set_min_shape_range配置最大和最小形状信息。
b.配置好对应的输入list。
c.配置好对应的输出,H2H模式时可以不进行配置,当为D2D模式时输出形状需要配置为最大形状。
d.调用runV2接口进行推理计算。
e.同步获取输出数据,并释放相关资源,当为D2D模式时需要现获取真实输出形状。
调用Python中runV2接口进行动态形状推理例子:
# 读取模型并生成engine
onnx_parser = TopsInference.create_parser(TopsInference.ONNX_MODEL)
onnx_parser.set_input_names(["587", "814"])
onnx_parser.set_input_shapes([[1, -1, -1, 256], [1, -1, -1, 512]])
network = onnx_parser.read("/path/to/your/model/")
optimizer = TopsInference.create_optimizer()
# 设置输入最大形状max-shape
max_shape_dim_setting = []
max_shape_dim = {}
max_shape_dim["main"] = [[1,256,256,256], [1,128,128,512]]
max_shape_dim_setting.append(max_shape_dim)
optimizer.set_max_shape_range(max_shape_dim_setting)
# 设置输入最小形状min-shape
min_shape_dim_setting = []
min_shape_dim = {}
min_shape_dim["main"] = ["1,4,4,256", "1,2,2,512"]
min_shape_dim_setting.append(min_shape_dim)
optimizer.set_min_shape_range(min_shape_dim_setting)
engine = optimizer.build(network)
# 设置输入
input0 = np.random.randint(0, 100, (1, 256, 256, 256)).astype(np.float32)
input1 = np.random.randint(0, 100, (1, 128, 128, 512)).astype(np.float32)
# 设置输出
outputs = []
# 调用runV2
stream = TopsInference.create_stream()
future = engine.runV2([input0, input1], py_stream=stream)
# 获取输出
outputs = future.get()
Python侧在H2H时输入可以仅配置input list,输出运行完自动获取真实的形状和数据。
D2D模式例子,读取模型并生成engine和上述相同,形状尺寸信息需要由用户来进行配置:
# 读取模型并生成engine
...
# 设置输入
input0 = np.random.randint(0, 100, (1, 256, 256, 256)).astype(np.float32)
input1 = np.random.randint(0, 100, (1, 128, 128, 512)).astype(np.float32)
stream = TopsInference.create_stream()
input_num = engine.get_input_num()
inputs = [input0, input1]
p_inputs = []
for ind in range(input_num):
# 尺寸需要乘float的4字节
input_size = np.size(np.array(inputs[ind])) * 4
p_inputs.append(TopsInference.mem_alloc(input_size))
# 将输入数据配置到GCU上
p_inputs[ind].set_shape(np.array(inputs[ind]).shape)
TopsInference.mem_h2d_copy_async(inputs[ind], p_inputs[ind], input_size, stream)
# 设置输出,在运行前将输出配置为max,运行完以真实的形状来获取
p_outputs = []
output_num = engine.get_output_num()
for ind in range(output_num):
# 尺寸需要乘float的4字节
output_size = 4
sub_output_shape = engine.get_max_output_shape(ind)
for d in sub_output_shape:
if d == -1:
p_outputs.append(None)
output_size *= d
p_outputs.append(TopsInference.mem_alloc(output_size))
p_outputs[ind].set_shape(sub_output_shape)
# 调用runV2
future = engine.runV2(p_inputs, output_list=p_outputs, py_stream=stream)
# 获取输出
outputs = future.get()
host_outputs = []
for ind in range(output_num):
# 获取推理完真实的输出尺寸和形状
real_buf_size = outputs[ind].get_real_size()
out_shape = outputs[ind].get_real_shape()
# out_shape[0] = 1是根据实际的batch_size配置
# 如果第一维不表示batch_size,可删除
out_shape[0] = 1
host_outputs.append(np.zeros(out_shape, dtype=np.float32))
TopsInference.mem_d2h_copy_async(p_outputs[ind], host_outputs[ind], real_buf_size, stream)
stream.synchronize()
在Python中,用户可以使用get_input_shape(index)获取ONNX模型中第index个输入设置的形状,动态形状中会包含-1。 使用get_max_input_shape(index)可以获取第index个输入设置的最大形状,同样,get_min_input_shape(index)可以获取第index个输入设置的最小形状。 当模型为静态形状模型时,get_max_input_shape和get_min_input_shape返回结果和ONNX模型中输入形状相同。 使用get_max_output_shape(index)获取第index个输出的最大形状。
C++动态形状推理¶
C++在使用动态形状时,主要分为五个步骤:
a.读取模型并生成engine,对应输入维度设置为-1,在engine的生成过程中要配置最大和最小形状信息。
b.配置好对应的输入ITensor信息,注意要配置所有输入的ITensor信息。
c.配置好对应的输出ITensor信息,配置所有输出ITensor的信息,形状设置成最大形状。
d.调用runV2接口进行推理计算。
e.同步获取真实输出形状信息和输出数据,并释放相关资源。
调用C++中runV2接口进行动态形状推理例子:
// 读取模型并生成engine
TopsInference::IParser* parser = TopsInference::create_parser(TopsInference::TIF_ONNX);
parser->setInputNames("[587, 814]");
TopsInference::INetwork* network = nullptr;
network = parser->readModel("/path/to/your/model/");
TopsInference::IOptimizer* optimizer = TopsInference::create_optimizer();
// 设置输入最大形状max-shape
Json::Value max_shape_range_setting;
Json::Value op_max_val;
op_max_val["main"].append("1,256,256,256");
op_max_val["main"].append("1,128,128,512");
max_shape_range_setting.append(op_max_val);
std::string max_setting_str = max_shape_range_setting.toStyledString();
// 设置输入最小形状min-shape
Json::Value min_shape_range_setting;
Json::Value op_min_val;
op_min_val["main"].append("1,4,4,256");
op_min_val["main"].append("1,2,2,512");
min_shape_range_setting.append(op_min_val);
std::string min_setting_str = min_shape_range_setting.toStyledString();
TopsInference::IOptimizerConfig* optimizer_config = optimizer->getConfig();
optimizer_config->setMaxShapeRange(max_setting_str.c_str());
optimizer_config->setMinShapeRange(min_setting_str.c_str());
TopsInference::IEngine* engine = optimizer->build(network);
// 设置输入ITensor 注意类型要和配置的input dtype匹配
std::vector<std::vector<float>> input_data(4);
input_data[0].resize(128 * 128 * 256);
input_data[1].resize(128 * 128 * 512);
...
std::vector<TopsInference::TensorPtr_t> input_tensor_list;
int32_t input_num = engine->getInputNum();
for (size_t i = 0; i < input_num; i++) {
TopsInference::TensorPtr_t sub_input = TopsInference::create_tensor();
// 需要设置输入ITensor的数据
sub_input->setOpaque(reinterpret_cast<void*>(input_data[i].data()));
// 需要使用设置输入ITensor的DeviceType
sub_input->setDeviceType(TopsInference::DataDeviceType::HOST);
TopsInference::Dims input_shape = engine->getInputShape(i);
input_shape.dimension[0] = 1;
input_shape.dimension[1] = 128;
input_shape.dimension[2] = 128;
input_shape.dimension[3] = 256 * pow(2, i);
// 需要使用设置输入ITensor的dims
sub_input->setDims(input_shape);
input_tensor_list.emplace_back((TopsInference::TensorPtr_t)sub_input);
}
// 设置输出ITensor 注意类型要和配置的output dtype匹配,多种类型可以不使用vector
std::vector<std::vector<float>> outs;
std::vector<TopsInference::TensorPtr_t> output_tensor_list;
int32_t output_num = engine->getOutputNum();
outs.resize(output_num);
for (size_t i = 0; i < output_num; ++i) {
TopsInference::TensorPtr_t sub_output = TopsInference::create_tensor();
TopsInference::Dims max_shape = engine->getMaxOutputShape(i);
int64_t out_element_size = 1;
// max_shape.dimension[0]是根据实际的batch_size配置
// 如果第一维不表示batch_size,可删除
max_shape.dimension[0] = 1;
for (size_t j = 0; j < max_shape.nbDims; ++j) {
out_element_size *= max_shape.dimension[j];
}
outs[i].resize(out_element_size);
// 需要设置输出ITensor的数据
sub_output->setOpaque(reinterpret_cast<void*>(outs[i].data()));
// 需要使用maxoutputshape设置输出ITensor的dims,设置为最大输出形状
sub_output->setDims(max_shape);
// 需要设置输出ITensor的DeviceType
sub_output->setDeviceType(TopsInference::DataDeviceType::HOST);
output_tensor_list.emplace_back((TopsInference::TensorPtr_t)sub_output);
}
TopsInference::topsInferStream_t stream;
TopsInference::create_stream(&stream);
// 调用runV2
engine->runV2(input_tensor_list.data(), output_tensor_list.data(), stream);
// 获取输出
TopsInference::synchronize_stream(stream);
for (size_t i = 0; i < output_num; ++i) {
int64_t real_element_num = 1;
// 需要使用真实的输出形状来获取结果数据
TopsInference::Dims ouput_shape = output_tensor_list[i]->getDims();
for (size_t index = 0; index < ouput_shape.nbDims; ++index) {
real_element_num *= ouput_shape.dimension[index];
}
outs[i].resize(real_element_num);
}
// 释放资源
for (size_t i = 0; i < input_num; ++i) {
TopsInference::destroy_tensor(input_tensor_list[i]);
}
for (size_t i = 0; i < output_num; ++i) {
outs[i].clear();
TopsInference::destroy_tensor(output_tensor_list[i]);
}
input_tensor_list.clear();
output_tensor_list.clear();
TopsInference::destroy_stream(stream);
outs.clear();
std::vector<std::vector<float>>().swap(outs);
TopsInference::release_parser(parser);
TopsInference::release_network(network);
TopsInference::release_optimizer(optimizer);
TopsInference::release_engine(engine);
在C++中,用户可以使用getInputShape(index)获取ONNX模型中第index个输入设置的形状,动态形状中会包含-1。 使用getMaxInputShape(index)可以获取第index个输入设置的最大形状,同样,getMinInputShape(index)可以获取第index个输入设置的最小形状。 当模型为静态形状模型时,getMaxInputShape和getMinInputShape返回结果和ONNX模型中输入形状相同。 使用getMaxOutputShape(index)获取第index个输出的最大形状。
4.8. refit engine权重更新¶
为支持一些需要频繁更新权重的推理场景,如自对弈应用,TopsInference支持refit功能,节省了用户重新生成engine的编译过程,仅需更新权重。
开启refit功能¶
如果想要使用refit的功能,在生成engine之前,优化器optimizer需要配置为REFIT模式,这样生成的engine才可以支持refit。
Python代码如下:
optimizer.set_build_flag(TopsInference.KREFIT)
engine = optimizer.build(network)
C++代码如下:
TopsInference::IOptimizerConfig* optimizer_config = optimizer->getConfig();
optimizer_config->setBuildFlag(TopsInference::BuildFlag::TIF_REFIT);
TopsInference::IEngine* engine = optimizer->build(network);
如果想要使用refit自动预处理功能,优化器optimizer需要配置为REFIT模式,并使能自动预处理功能。设置了自动预处理功能,不需要用户提前对weight做预处理,直接用onnx的name和weight进行refit即可。使能自动预处理之后,会禁用某些pass,可能会带来性能下降。
python配置语句为:
optimizer.enable_refit_preprocess()
C++配置语句为:
optimizer_config->setRefitPreprocess(true)
如果使用refit功能同时想要启用混精功能,请使用“或(|)”逻辑去实现,可以将KREFIT和任意精度配合使用。
Python配置语句为:
optimizer.set_build_flag(TopsInference.KREFIT | TopsInference.KFP16_MIX)
C++配置语句为:
optimizer_config->setBuildFlag(TopsInference::BuildFlag::TIF_REFIT |
TopsInference::BuildFlag::TIF_KTYPE_MIX_FP16);
refit权重更新¶
用户使用refit功能,需要创建refitter,使用set_named_weights将要更新的weight绑定层的名称设置到refit中,最后使用refit_engine就可以把新的权重更新到engine中,此时再运行engine推理就会使用新的权重。
Python代码如下:
refitter = TopsInference.create_refitter(engine)
# 获取engine所有可以refit的层名称
all_weights = refitter.get_all_weights()
print("all_weights:{}".format(all_weights))
# 查看当前engine是否支持weight自动预处理
is_support_preprocess = refitter.is_support_preprocess()
print("is_support_preprocess:{}".format(is_support_preprocess))
# 使用numpy隐式构造权重
weight_data = np.zeros((390, 400), dtype=np.float32)
# 1.如果没有使能自动预处理:layer名称是hlir last pass的名称,weights是经过
# hlir pass处理之后的weights,具体经过的哪些预处理需要分析hlir dump文件
# 或者咨询开发人员
# 2.如果使能了自动预处理:layer名称是onnx layer名字,weights是onnx的weights
refitter.set_named_weights("layer_name", weight_data)
# 使用TopsInference.Weights构造权重
# weights = TopsInference.Weights(weight_data)
# print(weights.dtype)
# print(weights.nbytes)
# print(weights.size)
# 1.如果没有使能自动预处理:layer名称是hlir last pass的名称,weights是经过
# hlir pass处理之后的weights,具体经过的哪些预处理需要分析hlir dump文件
# 或者咨询开发人员
# 2.如果使能了自动预处理:layer名称是onnx layer名字,weights是onnx的weights
# refitter.set_named_weights("layer_name", weights)
# 可以使用get_named_weights去检查权重是否配置成功
# weights_check = TopsInference.Weights(TopsInference.TIF_FP32)
# layer名称是onnx layer的名称
# refitter.get_named_weights("layer_name", weights_check)
# 检查是否还有遗漏未更新的权重
assert(refitter.get_missing_weights() == [])
# refit后engine更新权重
refitter.refit_engine()
get_all_weights是为了获取所有可以refit的层名称;is_support_preprocess可以获取当前engine是否支持weight自动预处理,用户可以根据返回值确定是否需要做预处理;权重可以使用numpy数据或者实现TopsInference的weight; set_named_weights是将特定名称层的权重配置进去;可以使用get_named_weights去检查权重是否配置成功;get_missing_weights检查是否有遗漏的层没有更新权重;refit_engine之后,权重就在engine更新了。
Attention
1.如果没有使能自动预处理,set_named_weights、get_named_weights配置层名称和weights时,需要使用hlir last pass的名称和优化后的weights,因为通过TopsInference优化,图会被重构,TopsInference的层和onnx的层并不是一一对应。sdk版本升级可能会导致constant优化和层名称发生变化;如果用户重新编译engine,则需要适配新的constant优化,重新set_named_weights并refit_engine;如果不重新编译engine,则不需要重新refit。2.如果使能了自动预处理,配置层名称和weights跟onnx保持一致,TopsInference会自动进行预处理,不过使能自动预处理之后由于禁用了一些pass,可能会导致模型推理性能下降。
在C++代码如下:
TopsInference::IRefitter* refit = TopsInference::create_refitter(engine);
// 获取engine所有可以refit的层名称
int32_t const size{refit->getAllWeights(0, nullptr)};
std::vector<char const*> names(size);
auto size_ret = refit->getAllWeights(size, names.data());
// 查看当前engine是否支持自动预处理
auto is_support_preprocess = refit->isSupportPreprocess()
// 使用TopsInference::Weights构造权重
TopsInference::Weights weights;
float* newBiasLocal = new float[8];
for (size_t i = 0; i < 8; i++) {
newBiasLocal[i] = 0.0001 * i;
}
weights.count = 8;
weights.values = newBiasLocal;
weights.type = TopsInference::DataType::TIF_FP32;
auto ret = refit->setNamedWeights("layer_name", weights);
// 可以使用getNamedWeights去检查权重是否配置成功
TopsInference::Weights get_weights{TopsInference::DataType::TIF_UINT8, 0,
nullptr};
refit->getNamedWeights(
"fwffm_pbp_mlp/dense_14/MatMul/ReadVariableOp/_20__cf__20:0",
get_weights);
for (size_t i = 0; i < 8; i++) {
ASSERT_EQ(
*(reinterpret_cast<float*>(const_cast<void*>(get_weights.values)) + i),
newBiasLocal[i]);
}
// 检查是否有遗漏的权重没有配置
assert(refit->getMissingWeights(0, nullptr) == 0);
// refit后engine更新权重
refit->refitEngine();
getAllWeights是为了获取所有可以refit的层名称,需要调用两遍,第一遍参数使用0和空指针,获取可以配置的数量,第二遍获取所有的名称;isSupportPreprocess可以获取当前engine是否支持weight自动预处理,用户可以根据返回值确定是否需要自己做预处理; 权重配置和Python不同,C++必须使用TopsInference::Weights结构体去构造Weigtht;setNamedWeights是将特定名称层的权重配置进去;可以使用getNamedWeights去检查权重是否配置成功;使用getMissingWeights检查是否有遗漏的层没有更新权重; refitEngine之后,权重就在engine更新了。
4.9. 自定义算子实现¶
用户使用TopsInference时,想要使用未支持或自定义的的算子,可以选择自定义算子开发的方法。TopsInference支持 AOT(Ahead Of Time,运行前编译)和 JIT(Just In Time,运行时编译)两种用户自定义算子的实现。
本节建议对GCU和TopsInference已有深入了解,熟悉掌握C++编程的用户使用。
自定义AOT算子¶
AOT类型的自定义算子采用运行前编译编译方式,用户基于算子实现接口,手写算子实现函数对应的源码文件,并提前将源码文件编译为动态链接库,然后在生成engine时调用动态链接库中的函数,在推理前生成GCU上执行的算子代码。
自定义AOT算子当前仅支持静态形状算子,如果想要自定义动态形状算子请使用 自定义JIT算子 。
如果用户想要实现一个自定义加法,ONNX结构图如下:
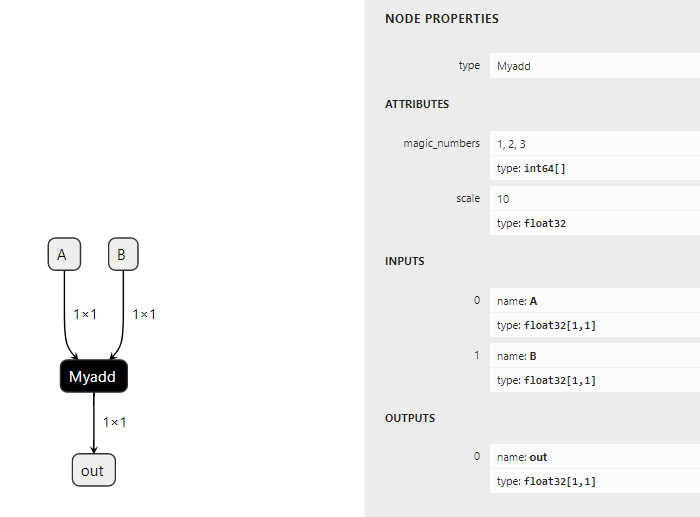
图 4.9.1 ONNX自定义加法算子结构示例图¶
自定义算子实现¶
算子实现的kernel需要用C/C++实现:
extern "C" void my_add_kernel(float* dev_input0, TensorShape* s0,
float* dev_input1, TensorShape* s1,
float* dev_output, TensorShape* o, float scale) {
auto size =
std::accumulate(s0->dims, s0->dims + 2, 1, std::multiplies<int64_t>());
size *= sizeof(float);
float* input0_host = new float[1];
float* input1_host = new float[1];
float* out_host = new float[1];
// 使用runtime的host和device直接的拷贝接口
topsMemcpyDtoH(input0_host, dev_input0, size);
topsMemcpyDtoH(input1_host, dev_input1, size);
for (auto i = 0; i < 1; i++) {
out_host[i] = input0_host[i] + scale * input1_host[i];
}
topsMemcpyHtoD(dev_output, out_host, size);
}
用户新建继承hlir::HlirOpKernel的MyAddAotOP类,其中要去实现getPriority、dynamicOpSelect、staticOpSelect、compile方法。
class MyAddAotOp : public hlir::HlirOpKernel {
public:
using hlir::HlirOpKernel::HlirOpKernel;
int getPriority(const hlir::HlirOpKernelDesc& kernel_description) const;
void dynamicOpSelect(const hlir::HlirOpKernelContext& context) const;
void staticOpSelect(const hlir::HlirOpKernelContext& context) const;
void compile(const hlir::HlirOpKernelContext& context) const override;
private:
void handle_fusion(const hlir::HlirOpKernelContext& context) const;
};
Factor类型使用说明¶
Factor的类型包括ArrayType和StructType。定义如下:
/**
* @brief Helper to create an array type.
* @param element_type Data type of array element, which must be in {BoolType,
* IntType, UIntType, FloatType, BFloatType, StructType}.
* @param shape Number of elements on each dimension.
* @return A type of array.
*/
FACTOR_EXPORT Type ArrayType(const Type &element_type,
const std::vector<int64_t> &shape);
/**
* @brief Helper to create a struct type.
* @param tag Structure tag.
* @param members Member definition including member type and member name.
* member type must be in {BoolType, IntType, UIntType,
* FloatType, BFloatType, ArrayType, StructType}.
* @return A type of struct.
*/
FACTOR_EXPORT Type StructType(const char *tag,
const std::vector<std::pair<Type, const char*>> &members);
基于上述接口,下面给出一些定义的范例,注释部分给出了C语言的等价定义。
/// Sample of struct type
/* struct Point {
float x;
float y;
}; */
auto point_type = StructType("Point", {{FloatType(32), "x"},
{FloatType(32), "y"}});
/* struct Point* */
auto ptr_type = PtrType(point_type);
/* struct Mixed {
bool a;
char b;
unsigned char c;
short d;
unsigned short e;
int f;
unsigned int g;
float h;
int i[10];
struct Point j;
}; */
auto mixed_type = StructType("Mixed", {{BoolType(), "a"},
{IntType(8), "b"},
{UIntType(8), "c"},
{IntType(16), "d"},
{UIntType(16), "e"},
{IntType(32), "f"},
{UIntType(32), "g"},
{FloatType(32), "h"},
{ArrayType(IntType(32), {10}), "i"},
{point_type, "j"}});
/// Sample of array type
// int[10]
auto s32_1d_array = ArrayType(IntType(32), {10});
// unsigned int[10]
auto u32_1d_array = ArrayType(UIntType(32), {10});
// float [][3][4][5];
auto f32_4d_array = ArrayType(FloatType(32), {2, 3, 4, 5});
// f16's raw data
// unsigned short[10];
auto f16_1d_array = ArrayType(FloatType(16), {10});
// bf16's raw data
// unsigned short[10];
auto bf16_1d_array = ArrayType(BFloatType(16), {10});
// struct Point points[10];
auto struct_1d_array = ArrayType(point_type, {10});
// struct Mixed mixed[3][4];
auto mixed_2d_array = ArrayType(mixed_type, {3, 4});
ArrayType的元素数据类型,支持factor所有的scalar类型以及StructType类型。ArrayType中的数组维度没有限制,大于等于1即可。
StructType的成员数据类型,支持factor所有的scalar类型,ArrayType类型以及StructType类型。
ArrayType和StructType都不支持64位的整型与浮点,ArrayType和StructType都不支持PtrType,ArrayType的元素不能是ArrayType。
自定义算子形状推理¶
用户还必须提供C++的形状推理函数:
using Range = hlir::TensorShape::Range;
using Shape = hlir::TensorShape;
using Param = hlir::HlirOpParam;
using ParamVal = hlir::HlirOpParamVal;
void MyShapeInfer(const std::vector<Shape> inputs, const Param& params,
std::vector<Shape>& outputs) {
auto input0 = inputs[0];
auto input1 = inputs[1];
auto output = Shape(input0);
outputs.clear();
outputs.emplace_back(output);
}
自定义算子注册¶
用户需要通过REGISTER_HLIR_OP和REGISTER_HLIR_KERNEL注册AOT算子信息,包括算子的inputs、outputs、参数信息、算子的optype,以及算子实现的函数名称等信息。
构造AOT算子信息:
REGISTER_HLIR_OP(MyAdd)
.addInputs(2)
.addOutputs(1)
.addParam("scale", ParamVal(1.f))
.setShapeInfer(MyShapeInfer);
REGISTER_HLIR_KERNEL(hlir::Name("custom_call")
.Unique("MyAdd")
.Device(hlir::GCU_DEVICE)
.TypeConstraint({::dtu::op::DataType::F32,
::dtu::op::DataType::F16}),
MyAddAotOp);
注册算子是针对ONNX的构建过程,其中addInputs表示输入的数量,addOutputs表示输出的数量,addParam表示算子参数,setShapeInfer设置上述用户自定义的推理形状程序,定义好函数传入函数名称即可。
加载自定义算子¶
用户将以上的C++代码生成为动态链接库so传给TopsInference就可以完成自定义算子的kernel绑定。
C++在编译时链接so文件即可,如下:
target_link_libraries(app library1 <library2> ... custom)
将以上代码编译生成.so文件,在使用前加载自定义算子库即可。
TopsInference在Python侧加载自定义算子方法如下:
build_path = "/path/to/your/build/so"
TopsInference.load_hlir_ops("my_add", build_path)
至此自定义算子就已经注册好,开启环境变量 “export ENABLE_HOST_COMPILE=true” , “export LD_LIBRARY_PATH=$LD_LIBRARY_PATH:/path/to/your/build/” 用户可以正常生成engine并推理。
自定义JIT算子¶
自定义JIT算子在模型推理时才会生成GCU的算子代码,支持静态和动态形状算子,但是需要用户自行开发的代码也更多。和AOT算子一样,用户需注册给TopsInference相关的算子信息,包括输入,输出,属性等相关信息。
除此之外,用户还需提供按照Factor(Factor是燧原提供的专门用于数据流编程的领域特定语言)的构图方式实现虚基类搭建图,因此用户必须掌握Factor编写技能。
自定义算子实现¶
用户需要继承hlir::HlirOpKernel类去定义自己的算子类,其中要完善getPriority、compile方法。
保证Compile最终调用的是自定义算子实现,如下代码中最终调用的是DoradoSparseFieldsConcatV2Impl。
class GcuSparseFieldsConcatV2Op : public hlir::HlirOpKernel {
public:
using hlir::HlirOpKernel::HlirOpKernel;
int getPriority(const hlir::HlirOpKernelDesc& kernel_description) const override {
if (!hlir::isHlirCustomOp(kernel_description.op)) {
return -1;
}
auto op_and_param =
hlir::getOpDefAndParam(kernel_description.op);
if (!op_and_param.first.isOpType("my_sparse_fields_concat_v2")) {
return -1;
}
auto in_out_shapes =
hlir::getHlirOpInOutShapes(kernel_description.op);
auto input_shapes = in_out_shapes.first;
auto output_shapes = in_out_shapes.second;
auto feature_weight_shape = input_shapes[0].Dimensions();
auto feature_field_shape = input_shapes[1].Dimensions();
auto feature_index_shape = input_shapes[2].Dimensions();
auto output1_shape = output_shapes[0].Dimensions();
auto output2_shape = output_shapes[1].Dimensions();
if (feature_weight_shape.size() != 3 || feature_field_shape.size() != 2 ||
feature_index_shape.size() != 1) {
return -1;
}
auto feature_weight_dtype = input_shapes[0].GetElementType();
auto feature_field_dtype = input_shapes[1].GetElementType();
auto feature_index_dtype = input_shapes[2].GetElementType();
auto output1_dtype = output_shapes[0].GetElementType();
auto output2_dtype = output_shapes[1].GetElementType();
if (feature_weight_dtype != hlir::HlirDataType::F32) {
return -1;
}
if (feature_field_dtype != hlir::HlirDataType::S32) {
return -1;
}
if (feature_index_dtype != hlir::HlirDataType::S32) {
return -1;
}
if (output1_dtype != feature_weight_dtype ||
output2_dtype != feature_weight_dtype) {
return -1;
}
return true;
}
void compile(const hlir::HlirOpKernelContext& context) const override {
DoradoSparseFieldsConcatV2Impl(context);
}
};
用户需要自己实现Factor中对数据流的定义,代码为:
void DoradoSparseFieldsConcatV2Impl(
const hlir::HlirOpKernelContext& context) {
// ...
}
自定义算子形状推理¶
用户需要使用C++自定义形状推理程序。
以下是自定义的形状推理程序示例:
using Range = hlir::TensorShape::Range;
using Shape = hlir::TensorShape;
using HlirOpParam = hlir::HlirOpParam;
using namespace hlir;
void myShapeInfer(const std::vector<Shape> inputs, const HlirOpParam& params,
std::vector<Shape>& outputs) {
auto input_shape = inputs[0];
auto field_shape = inputs[1];
auto index_shape = inputs[2];
auto output_dtype = input_shape.GetElementType();
auto part1_fields = params["part1_fields"].as<std::vector<int64_t>>();
auto part2_fields = params["part2_fields"].as<std::vector<int64_t>>();
int32_t part1_len = part1_fields.size();
int32_t part2_len = part2_fields.size();
EFCHECK_EQ(input_shape.ndim(), 3);
std::vector<int64_t> input_dimensions = input_shape.Dimensions();
Range input_range = input_shape.GetDimensionsRange();
std::vector<int64_t> output1_dimensions(2);
Shape::Range output1_range;
output1_dimensions.at(0) = kUnknownDim;
output1_range.first.emplace_back(1);
output1_range.second.emplace_back(2048);
std::vector<int64_t> output2_dimensions(2);
Shape::Range output2_range;
output2_dimensions.at(0) = kUnknownDim;
output2_range.first.emplace_back(1);
output2_range.second.emplace_back(2048);
if (input_dimensions.at(1) != kUnknownDim &&
input_dimensions.at(2) != kUnknownDim) {
int32_t embedding_size = input_dimensions.at(1) * input_dimensions.at(2);
output1_dimensions.at(1) = part1_len * embedding_size;
output1_range.first.emplace_back(output1_dimensions.at(1));
output1_range.second.emplace_back(output1_dimensions.at(1));
output2_dimensions.at(1) = part2_len * embedding_size;
output2_range.first.emplace_back(output2_dimensions.at(1));
output2_range.second.emplace_back(output2_dimensions.at(1));
} else {
output1_dimensions.at(1) = kUnknownDim;
output1_range.first.emplace_back(1);
output1_range.second.emplace_back(kMaxDim);
output2_dimensions.at(1) = kUnknownDim;
output2_range.first.emplace_back(1);
output2_range.second.emplace_back(kMaxDim);
}
auto out1_shape = Shape(output_dtype, output1_dimensions,
output1_range.second, output1_range.first);
outputs.at(0) = out1_shape;
auto out2_shape = Shape(output_dtype, output2_dimensions,
output2_range.second, output2_range.first);
outputs.at(1) = out2_shape;
}
自定义算子注册¶
完成上述工作后要注册算子,以下是注册算子的示例:
int64_t field_num = 3;
std::vector<int64_t> part1{};
std::vector<int64_t> part2{1, 2, 3};
REGISTER_HLIR_OP(my_sparse_fields_concat_v2)
.addInputs(3)
.addOutputs(2)
.addParam("fw_field_num", hlir::HlirOpParamVal(field_num))
.addParam("part1_fields", hlir::HlirOpParamVal(part1))
.addParam("part2_fields", hlir::HlirOpParamVal(part2))
.setShapeInfer(myShapeInfer);
REGISTER_HLIR_KERNEL(hlir::Name("custom_call")
.Unique("my_sparse_fields_concat_v2")
.Device(hlir::GCU_DEVICE)
.TypeConstraint({hlir::HlirDataType::F32,
hlir::HlirDataType::S32,
hlir::HlirDataType::F16}),
GcuSparseFieldsConcatV2Op);
注册算子是针对ONNX的构建过程,其中addInputs表示输入的数量,addOutputs表示输出的数量,addParam表示算子参数,setShapeInfer设置上述用户自定义的推理形状程序,定义好函数,传入函数名称即可。
REGISTER_HLIR_KERNEL是对GCU算子的注册工作,其中Name为固定的“custom_call”,Unique中设置和REGISTER_HLIR_OP相同的名称,Device如果为GCU设备请设置为hlir::GCU_DEVICE,第二个参数为继承hlir::HlirOpKernel的类,也即算子的实现。
和AOT算子一样,用户可以按照C++工程自行编译成so文件。之后按照正常的TopsInference构建推理流程就会自动包含用户自定义的JIT算子。
用户将以上的C++代码生成为动态链接库so传给TopsInference就可以完成自定义算子的kernel绑定。
C++在编译时链接so文件即可,如下:
target_link_libraries(app library1 <library2> ... custom)
将以上代码编译生成.so文件,在使用前加载自定义算子库即可。
TopsInference在Python侧加载自定义算子方法如下:
build_path = "/path/to/your/build/so"
TopsInference.load_hlir_ops("my_add", build_path)
自定义算子支持所有的推理接口,如run、runV2等,示例代码见 用户自定义JIT算子 。
4.10. I/O 数据格式¶
在模型中,数据格式存在多种,1D,2D,3D,4D,5D等维度不同,拥有的数据格式不同,比如4D维度中,图像处理的数据格式有NCHW,NHWC,CHWN。 传统的run接口兼容了这种格式,但是runV2需要用户来感知,python中配合使用engine.set_io_dimension_info,c++中配合使用 engine->setIODimensionInfo来配置BatchSize 也就是N在维度中的哪个位置。比如CHWN中N所在的位置3,NCHW中N的位置在0。 对于不包含N也就是BatchSize信息的输入输出,就指定为-1。 另外参数也需要做一些声明,inputs/outputs均是一个一维数组,并且你需要保证它们的顺序和onnx/engine中inputs/outputs设定的顺序一致。
哪些接口支持¶
I/O数据格式支持,目前支持runV2,runV3接口。在这些场景使用在inputs/outputs 出现标量或者不包含维度N信息时,或者N不在维度0位置。
对于run,你可以不用设置。
配置I/O N位置的优势¶
当I/O中仅存在N在0位置,同时也存在标量时,或者不含维度信息的输入时。 显示配置I/O N位置可以进行多个cluster自动拆分,并行进行。通过传入大的Batch Inputs数据到runV2/runV3.
当I/O中存在N不在0位置时,使用runV2/runV3不会进行多个cluster自动拆分,需要用户通过多个stream异步下发,实现多个cluster并行处理
I/O 数据格式示例¶
我们以某个模型五个输入(包含标量),一个输出来举例(BatchSize=4):
输入如下:
名称 |
数据类型 |
形状 |
说明 |
---|---|---|---|
img |
float32 |
[4,224,224,3] |
向量 4D NHWC模式 |
scalar_var |
int32 |
[] |
标量,0-D不包含维度信息 |
input3 |
int64 |
[12, 4] |
向量 2D N维度不在0位置 |
input4 |
float32 |
[28,36] |
向量,2D N维度不存在 |
input5 |
float32 |
[12] |
向量,1D,不包含维度信息 |
输出如下:
名称 |
数据类型 |
形状 |
说明 |
---|---|---|---|
out1 |
float32 |
[4,224,224,3] |
向量 4D NHWC模式 |
使用python进行实现,如下:
...
engine = optimizer.build(network)
#配置IO的N在维度中的位置
engine.set_io_dimension_info(np.array([0,-1,1,-1,-1], dtype=np.int32), np.array([0], dtype=np.int32))
#设置inputs
scalar_var=3
inputs = [
img,
np.array([scalar_var], dtype=np.int64),
np.random.randint(0, 100, size=(12, 4), dtype=np.int64),
np.random.randint(0, 100, size=(28, 36), dtype=np.float),
np.random.rand(12).astype(np.float32)
]
future = engine.runV2(inputs)
gcu_outputs_v2 = future.get()
使用c++进行实现,如下:
...
std::unique_ptr<Resnet50Test> res50ptr(new Resnet50Test());
constexpr uint32_t cluster_id[] = {0, 1, 2, 3, 4, 5};
auto* handler = TopsInference::set_device(0, cluster_id,
/*cluster_num*/ 6, nullptr);
EFCHECK(handler != nullptr) << "[Error] set device failed!";
TopsInference::IErrorManager* error_manager =
TopsInference::create_error_manager();
ASSERT_TRUE(error_manager != nullptr)
<< "[Error] create error_manager failed!";
auto sg_err_manager = sg::make_scope_guard(
[=]() { TopsInference::release_error_manager(error_manager); });
auto h = std::make_unique<tops_h>();
res50ptr->prepareResEngine(h.get(), error_manager);
// test get...
type = h.get()->engine_ptr->getOutputDataType(0);
// setIODimensionInfo 需要填入全部的inputs的N所在位置,outputs同理
std::vector<int32_t> inputNIndices{0,-1,1,-1,-1},outputNIndices{0};
h.get()->engine_ptr->setIODimensionInfo(inputNIndices.data(),outputNIndices.data());
EXPECT_EQ(type, TopsInference::DataType::TIF_FP32);
TopsInference::Dims input_dim = h.get()->engine_ptr->getInputShape(0);
EXPECT_EQ(input_dim.nbDims, 4);
TopsInference::Dims output_dim = h.get()->engine_ptr->getOutputShape(0);
EXPECT_EQ(output_dim.nbDims, 4);
std::string input_str = h.get()->engine_ptr->getInputName(0);
std::string output_str = h.get()->engine_ptr->getOutputName(0);
std::cout << "input name:" << input_str << std::endl;
std::cout << "output name:" << output_str << std::endl;
// test runV2
TopsInference::topsInferStream_t stream_0;
if (!TopsInference::create_stream(&stream_0)) {
std::cout << "[Error] create stream_0 failed!";
}
EFDLOG(TOPS, TIF) << "start runV2 test!!";
constexpr int test_times = 10;
for (int32_t step = 0; step < test_times; step++) {
res50ptr->inferenceRunV2(h.get());
}
TopsInference::destroy_stream(stream_0);
TopsInference::release_device(handler);
4.11. I/O 最大HBM缓存用量¶
通过设定每个cluster上,I/O最大HBM缓存用量,传入,来限定TopsInference内部异步执行状态。默认情况下,每个cluster上 workspacesize为(3倍inputs byte size,2倍outputs byte size )之和,单位为byte。
支持场景¶
仅支持静态shape。
运行期,限定每个cluster上最大HBM用量上限(input,output buffer数),当work space size为0时,每个cluster上会仅使用一对input,output.
不同workspacesize策略走向¶
workspacesize设定的是每个cluster上,inputs,outputs会使用缓存的大小。 不同的workspacesize会有不同的性能,但是会引发HBM耗尽而出现OOM,用户需要调试获得HBM用量和性能之间的平衡, 下面展示了workspacesize是如何影响cluster上的执行流:
当workspacesize为0时,当你下发多笔任务,通过执行多次runV2,那么TopsInference执行流程 H2D->RUN->D2H,轮询执行N次,如下图所示:

图 4.11.1 TopsInference不同workspacesize策略执行流程示例图1¶
默认情况下,workspacesize=3*(total inputs byte size) + 2*(total outputs byte size), 当你下发多笔任务,通过执行多次runV2,那么TopsInference执行流程,如下所示:
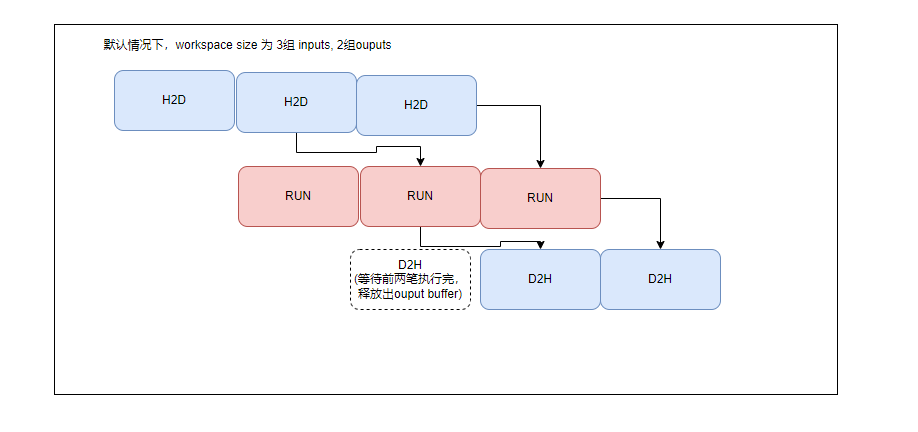
图 4.11.2 TopsInference不同workspacesize策略执行流程示例图2¶
代码示例¶
使用 python进行实现,如下:
def write_run_infer(card_id, cluster_id, img, assertEqual):
with TopsInference.device(card_id, cluster_id):
...
engine = optimizer.build(network)
engine.max_workspace_size=100*1024*1024 #100M
cluster_num = len(cluster_id)
streams = [TopsInference.create_stream() for _ in range(cluster_num)]
outputs_list = []
sum = 0
total_time = 0.
st = time.time()
c_ind = 0
for iind in range(1):
batch_size = random.randint(1, 17)
sum = sum + batch_size
inputs = np.concatenate((img,) * batch_size, axis=0).astype(np.float32)
stream = streams[c_ind]
c_ind = (c_ind + 1) % cluster_num
py_future = engine.run_with_batch(sample_nums=batch_size, input_list=np.array([inputs]), output_list=None,
buffer_type=TopsInference.TIF_ENGINE_RSC_IN_HOST_OUT_HOST,
py_stream=stream)
outputs_list.append(py_future)
ed = time.time()
total_time = total_time + (ed - st)
print(
"total image:{} ,speed: {} fps, latency: {} ms".format(sum, sum / total_time, ((total_time * 1000.) / sum)))
for iind in range(len(outputs_list)):
print("before wait, output is ready? {}".format(outputs_list[iind].status()))
outputs_list[iind].wait()
print("after wait, output is ready? {}".format(outputs_list[iind].status()))
ret = utils.decode_result(outputs_list[iind].get()[0])
assertEqual(ret[0][0][1], 'beagle')
使用c++进行实现,如下:
...
// test get...
TopsInference::DataType type = h.get()->engine_ptr->getInputDataType(0);
EXPECT_EQ(type, TopsInference::DataType::TIF_FP32);
type = h.get()->engine_ptr->getOutputDataType(0);
h.get()->engine_ptr->setMaxWorkspaceSize(100*1024*1024); //使用100MB
EXPECT_EQ(type, TopsInference::DataType::TIF_FP32);
TopsInference::Dims input_dim = h.get()->engine_ptr->getInputShape(0);
EXPECT_EQ(input_dim.nbDims, 4);
TopsInference::Dims output_dim = h.get()->engine_ptr->getOutputShape(0);
EXPECT_EQ(output_dim.nbDims, 2);
std::string input_str = h.get()->engine_ptr->getInputName(0);
std::string output_str = h.get()->engine_ptr->getOutputName(0);
std::cout << "input name:" << input_str << std::endl;
std::cout << "output name:" << output_str << std::endl;
// test runV2
TopsInference::topsInferStream_t stream_0;
if (!TopsInference::create_stream(&stream_0)) {
std::cout << "[Error] create stream_0 failed!";
}
EFDLOG(TOPS, TIF) << "start runV2 test!!";
constexpr int test_times = 10;
for (int32_t step = 0; step < test_times; step++) {
res50ptr->inferenceRunV2(h.get());
}
TopsInference::destroy_stream(stream_0);
TopsInference::release_device(handler);
}
4.12. 代码示例¶
本节为用户提供了TopsInference多种不同场景的demo,方便用户学习开发。安装完TopsInference之后,C++示例在/usr/local/gcu/sample/目录下,Python示例在/usr/local/lib/python3.8/site-packages/TopsInference/TopsInference_pysample_codes.tar压缩包中。
多batch模型推理示例¶
Python模型run_with_batch推理示例:
with TopsInference.device(card_id, cluster_id):
# 读取模型
onnx_parser = TopsInference.create_parser(TopsInference.ONNX_MODEL)
onnx_parser.set_input_names(['data'])
onnx_parser.set_input_shapes([[1, 3, 224, 224]])
onnx_parser.set_output_names(['resnetv17_dense0_fwd'])
onnx_parser.set_input_dtypes(TopsInference.TIF_FLOAT32)
network = onnx_parser.read("/path/to/your/model/")
# 生成engine
optimizer = TopsInference.create_optimizer()
optimizer.set_build_flag(TopsInference.KFP16_MIX)
engine = optimizer.build(network)
cluster_num = len(cluster_id)
streams = [TopsInference.create_stream() for _ in range(cluster_num)]
outputs_list = []
c_ind = 0
batch_size = random.randint(1, 17)
intputs = np.concatenate((img,) * batch_size, axis=0).astype(np.float32)
# output_list为空
stream = streams[c_ind]
c_ind = (c_ind + 1) % cluster_num
py_future = engine.run_with_batch(batch_size,
np.array([intputs]),
output_list=None,
buffer_type=TopsInference.TIF_ENGINE_RSC_IN_HOST_OUT_HOST,
py_stream=stream)
outputs_list.append(py_future)
# output_list不为空
py_future = engine.run_with_batch(batch_size,
np.array([intputs]),
output_list=[],
buffer_type=TopsInference.TIF_ENGINE_RSC_IN_HOST_OUT_HOST,
py_stream=stream)
outputs_list.append(py_future)
# 同步模式 py_stream=None
py_future = engine.run_with_batch(batch_size,
np.array([intputs]),
buffer_type=TopsInference.TIF_ENGINE_RSC_IN_HOST_OUT_HOST)
outputs_list.append(py_future)
# 同步模式 py_stream=None
py_future = engine.run_with_batch(batch_size,
np.array([intputs]),
output_list=[],
buffer_type=TopsInference.TIF_ENGINE_RSC_IN_HOST_OUT_HOST)
outputs_list.append(py_future)
C++模型runWithBatch推理示例:
// 读取模型
TopsInference::IParser* parser = TopsInference::create_parser(model_type);
parser->setInputNames("input");
std::string input_shape = std::to_string(BATCH_NUM) + ",224,224,3";
parser->setInputShapes(input_shape.c_str());
parser->setOutputNames("resnet_v1_50/predictions/Reshape_1");
TopsInference::INetwork* network = nullptr;
network = parser->readModel("/path/to/your/model/");
// 生成engine
TopsInference::IOptimizer* optimizer = TopsInference::create_optimizer();
TopsInference::IEngine* engine = optimizer->build(network);
std::vector<std::vector<float>> outs;
std::vector<std::vector<float>> inputs_guad;
outs.resize(steps);
inputs_guad.resize(steps);
auto storage_data = loadAndProcessImage("/path/to/your/image/");
int32_t total_img_nums = 0;
std::vector<int32_t> batch_list;
for (size_t t = 0; t < steps; t++) {
int32_t random_batch = 6;
total_img_nums += random_batch;
batch_list.emplace_back(random_batch);
for (int32_t i = 0; i < random_batch; i++) {
inputs_guad[t].insert(inputs_guad[t].end(),
storage_data.begin(), storage_data.end());
}
outs[t].resize(random_batch * 1000);
}
std::chrono::steady_clock::time_point now = std::chrono::steady_clock::now();
for (size_t t = 0; t < steps; t++) {
float* inputs[] = {inputs_guad[t].data()};
void** inputs_buffer = reinterpret_cast<void**>(inputs);
float* outputs[] = {outs[t].data()};
engine->runWithBatch(batch_list[t], inputs_buffer,
reinterpret_cast<void**>(outputs),
TopsInference::BufferType::TIF_ENGINE_RSC_IN_HOST_OUT_HOST,
stream);
}
TopsInference::synchronize_stream(stream);
// 执行推理
for (size_t t = 0; t < 1; t++) {
for (size_t batch_i = 0; batch_i < outs[t].size() / 1000; ++batch_i) {
auto* base_ptr = outs[t].data();
std::vector<float> output_vec(base_ptr + 1000 * batch_i,
base_ptr + 1000 * (batch_i + 1));
uint32_t output_idx = argsortMax(output_vec);
std::string result_name = getOutputName(output_idx);
}
}
// 释放资源
inputs_guad.clear();
outs.clear();
std::vector<std::vector<float>>().swap(outs);
std::vector<std::vector<float>>().swap(inputs_guad);
TopsInference::release_parser(parser);
TopsInference::release_network(network);
TopsInference::release_optimizer(optimizer);
TopsInference::release_engine(engine);
动态形状模型推理示例¶
TopsInference调用动态形状模型要使用runV2接口,在读取模型时设置input的形状动态维度为-1,在生成engine时要配置动态形状模型输入的最大和最小形状。
Python动态形状模型推理示例:
with TopsInference.device(0, 0):
# 读取模型
onnx_parser = TopsInference.create_parser(TopsInference.ONNX_MODEL)
onnx_parser.set_input_names(["133", "360", "587", "814"])
onnx_parser.set_input_shapes([[1, -1, -1, 64], [1, -1, -1, 128],
[1, -1, -1, 256], [1, -1, -1, 512]])
onnx_parser.set_input_dtypes([
TopsInference.TIF_FLOAT32, TopsInference.TIF_FLOAT32,
TopsInference.TIF_FLOAT32, TopsInference.TIF_FLOAT32
])
network = onnx_parser.read("/path/to/your/model/")
# 生成engine
optimizer = TopsInference.create_optimizer()
# 配置动态形状模型输入的最大和最小形状
max_shape_dim_setting = []
max_shape_dim = {}
max_shape_dim["main"] = [[1,512,512,64], [1,512,512,128],
[1,256,256,256], [1,128,128,512]]
max_shape_dim_setting.append(max_shape_dim)
optimizer.set_max_shape_range(max_shape_dim_setting)
min_shape_dim_setting = []
min_shape_dim = {}
min_shape_dim["main"] = [[1,16,16,64], [1,8,8,128],
[1,4,4,256], [1,2,2,512]]
min_shape_dim_setting.append(min_shape_dim)
optimizer.set_min_shape_range(min_shape_dim_setting)
engine = optimizer.build(network)
input0 = np.random.randint(0, 100, (1, 256, 256, 64)).astype(np.float32)
input1 = np.random.randint(0, 100, (1, 128, 128, 128)).astype(np.float32)
input2 = np.random.randint(0, 100, (1, 64, 64, 256)).astype(np.float32)
input3 = np.random.randint(0, 100, (1, 32, 32, 512)).astype(np.float32)
outputs = []
stream = TopsInference.create_stream()
# 执行推理
future = engine.runV2([input0, input1, input2, input3], py_stream=stream)
outputs = future.get()
C++动态形状模型推理示例:
struct EngineDeleter {
void operator()(TopsInference::IEngine* e) {
TopsInference::release_engine(e);
}
};
using EnginePtr = std::unique_ptr<TopsInference::IEngine, EngineDeleter>;
TEST(RunV2DynamicTest, dynamic) {
// 初始化TopsInference
TopsInference::topsInference_init();
uint32_t cluster_id[] = {0};
auto handler = TopsInference::set_device(0, cluster_id);
// 读取模型
TopsInference::IParser* parser = TopsInference::create_parser(TopsInference::TIF_ONNX);
parser->setInputNames("[133, 360, 587, 814]");
TopsInference::INetwork* network = nullptr;
network = parser->readModel("/path/to/your/model/");
// 生成engine
TopsInference::IOptimizer* optimizer = TopsInference::create_optimizer();
// 配置动态形状模型输入的最大和最小形状
Json::Value max_shape_range_setting;
Json::Value op_max_val1;
op_max_val1["main"].append("1,1024,1024,64");
op_max_val1["main"].append("1,512,512,128");
op_max_val1["main"].append("1,256,256,256");
op_max_val1["main"].append("1,128,128,512");
max_shape_range_setting.append(op_max_val1);
Json::Value min_shape_range_setting;
Json::Value op_min_val1;
op_min_val1["main"].append("1,16,16,64");
op_min_val1["main"].append("1,8,8,128");
op_min_val1["main"].append("1,4,4,256");
op_min_val1["main"].append("1,2,2,512");
min_shape_range_setting.append(op_min_val1);
std::string max_setting_str = max_shape_range_setting.toStyledString();
std::string min_setting_str = min_shape_range_setting.toStyledString();
TopsInference::IOptimizerConfig* optimizer_config =
optimizer->getConfig();
optimizer_config->setMaxShapeRange(max_setting_str.c_str());
optimizer_config->setMinShapeRange(min_setting_str.c_str());
TopsInference::IEngine* engine = optimizer->build(network);
// 注意类型要和配置的输入输出 dtype匹配,多种类型可以不使用vector
std::vector<std::vector<float>> input_data(4);
input_data[0].resize(1024 * 1024 * 64);
input_data[1].resize(512 * 512 * 128);
input_data[2].resize(256 * 256 * 256);
input_data[3].resize(128 * 128 * 512);
std::vector<std::vector<float>> outs;
// 配置输入和输出的ITensor
std::vector<TopsInference::TensorPtr_t> input_tensor_list;
std::vector<TopsInference::TensorPtr_t> output_tensor_list;
int32_t input_num = engine->getInputNum();
for (size_t i = 0; i < input_num; i++) {
TopsInference::TensorPtr_t sub_input = TopsInference::create_tensor();
sub_input->setOpaque(reinterpret_cast<void*>(input_data[i].data()));
sub_input->setDeviceType(TopsInference::DataDeviceType::HOST);
TopsInference::Dims input_shape = engine->getInputShape(i);
input_shape.dimension[0] = 1;
input_shape.dimension[1] = 16 * pow(2, 6 - i);
input_shape.dimension[2] = 16 * pow(2, 6 - i);
input_shape.dimension[3] = 16 * pow(2, i + 2);
sub_input->setDims(input_shape);
input_tensor_list.emplace_back((TopsInference::TensorPtr_t)sub_input);
}
int32_t output_num = engine->getOutputNum();
outs.resize(output_num);
for (size_t i = 0; i < output_num; ++i) {
TopsInference::TensorPtr_t sub_output = TopsInference::create_tensor();
TopsInference::Dims max_shape = engine->getMaxOutputShape(i);
int64_t out_element_size = 1;
// max_shape.dimension[0]是根据实际的batch_size配置
// 如果第一维不表示batch_size,可删除
max_shape.dimension[0] = 1;
for (size_t j = 0; j < max_shape.nbDims; ++j) {
out_element_size *= max_shape.dimension[j];
}
outs[i].resize(out_element_size);
sub_output->setOpaque(reinterpret_cast<void*>(outs[i].data()));
sub_output->setDims(max_shape);
sub_output->setDeviceType(TopsInference::DataDeviceType::HOST);
output_tensor_list.emplace_back((TopsInference::TensorPtr_t)sub_output);
}
TopsInference::topsInferStream_t stream;
TopsInference::create_stream(&stream);
// 执行推理
auto status = engine->runV2(input_tensor_list.data(),
output_tensor_list.data(), stream);
TopsInference::synchronize_stream(stream);
for (size_t i = 0; i < output_num; ++i) {
int64_t real_element_num = 1;
TopsInference::Dims ouput_shape = output_tensor_list[i]->getDims();
for (int32_t index = 0; index < ouput_shape.nbDims; ++index) {
real_element_num *= ouput_shape.dimension[index];
}
outs[i].resize(real_element_num);
}
// 释放资源
for (size_t i = 0; i < input_num; ++i) {
TopsInference::destroy_tensor(input_tensor_list[i]);
}
for (size_t i = 0; i < output_num; ++i) {
outs[i].clear();
TopsInference::destroy_tensor(output_tensor_list[i]);
}
outs.clear();
std::vector<std::vector<float>>().swap(outs);
input_tensor_list.clear();
output_tensor_list.clear();
TopsInference::destroy_stream(stream);
TopsInference::release_parser(parser);
TopsInference::release_network(network);
TopsInference::release_optimizer(optimizer);
TopsInference::release_engine(engine);
TopsInference::release_device(handler);
TopsInference::topsInference_finish();
}
在模型推理前设置输出ITensor时,要将形状设置为最大尺寸,推理完再将尺寸设置为真实的输出尺寸。
runV2调用静态形状模型推理示例(H2H异步调用)¶
Python接口如何使用runV2的实现静态形状模式,同时也是H2H的异步调用:
# 读取模型并生成engine
onnx_parser = TopsInference.create_parser(TopsInference.ONNX_MODEL)
network = onnx_parser.read("/path/to/your/model/")
optimizer = TopsInference.create_optimizer()
engine = optimizer.build(network)
# 调用runV2,异步执行推理
outputs = []
stream = TopsInference.create_stream()
future = engine.runV2([img], py_stream=stream)
outputs = future.get()
Python静态形状模式只要输入input_list,运行完从future中获取输出。
C++接口如何使用runV2的实现静态形状模式,同时也是H2H的异步调用:
// 读取模型并生成engine
TopsInference::IParser* parser = TopsInference::create_parser(TopsInference::TIF_ONNX);
parser->setInputNames("input");
std::string input_shape = std::to_string(BATCH_NUM) + ",224,224,3";
parser->setInputShapes(input_shape.c_str());
parser->setOutputNames("resnet_v1_50/predictions/Reshape_1");
TopsInference::INetwork* network = nullptr;
network = parser->readModel("/path/to/your/model/");
TopsInference::IOptimizer* optimizer = TopsInference::create_optimizer();
TopsInference::IEngine* engine = optimizer->build(network);
std::vector<std::vector<float>> outs;
std::vector<std::vector<float>> inputs_guad;
...
// 设置输入ITensor
float* inputs[] = {inputs_guad[t].data()};
std::vector<TopsInference::TensorPtr_t> input_tensor_list;
int32_t input_num = engine->getInputNum();
for (size_t i = 0; i < input_num; i++) {
TopsInference::TensorPtr_t sub_input = TopsInference::create_tensor();
// 需要设置输入ITensor的数据
sub_input->setOpaque(reinterpret_cast<void*>(inputs[i]));
// 需要设置输入ITensor的DeviceType
sub_input->setDeviceType(TopsInference::DataDeviceType::HOST);
TopsInference::Dims input_shape = engine->getInputShape(i);
input_shape.dimension[0] = batch_size;
// 需要使用设置输入ITensor的dims
sub_input->setDims(input_shape);
input_tensor_list.emplace_back((TopsInference::TensorPtr_t)sub_input);
}
// 设置输出ITensor
std::vector<TopsInference::TensorPtr_t> output_tensor_list;
int32_t output_num = engine->getOutputNum();
for (size_t i = 0; i < output_num; ++i) {
TopsInference::Dims max_shape = engine->getMaxOutputShape(i);
int64_t element_size = 1;
max_shape.dimension[0] = batch_size;
for (size_t j = 0; j < max_shape.nbDims; ++j) {
element_size *= max_shape.dimension[j];
}
std::vector<float> out(element_size);
outs[t] = out;
TopsInference::TensorPtr_t sub_output = TopsInference::create_tensor();
// 需要设置输出ITensor的数据
sub_output->setOpaque(reinterpret_cast<void*>(out.data()));
// 需要使用maxoutputshape设置输出ITensor的dims,设置为最大输出形状
sub_output->setDims(max_shape);
// 需要设置输出ITensor的DeviceType
sub_output->setDeviceType(TopsInference::DataDeviceType::HOST);
output_tensor_list.emplace_back((TopsInference::TensorPtr_t)sub_output);
}
// 调用runV2,异步执行推理
TopsInference::topsInferStream_t stream;
TopsInference::create_stream(&stream);
engine->runV2(input_tensor_list.data(), output_tensor_list.data(), stream);
TopsInference::synchronize_stream(stream);
// 获取输出outs
...
// 释放资源
for (size_t i = 0; i < input_num; ++i) {
TopsInference::destroy_tensor(input_tensor_list[i]);
}
for (size_t i = 0; i < output_num; ++i) {
TopsInference::destroy_tensor(output_tensor_list[i]);
}
output_tensor_list.clear();
input_tensor_list.clear();
inputs_guad.clear();
std::vector<std::vector<float>>().swap(inputs_guad);
outs.clear();
std::vector<std::vector<float>>().swap(outs);
C++静态形状模式要先配置好输入输出的ITensor,再调用runV2接口计算结果,最后要释放所有使用的资源。
混合精度模型推理示例¶
Python混合精度模型推理示例:
handler = TopsInference.set_device(0, 0)
# 读取模型
onnx_parser = TopsInference.create_parser(TopsInference.ONNX_MODEL)
onnx_parser.set_input_names(["input.1", "1"])
onnx_parser.set_input_shapes([[1, 1, 32, 1600], [1]])
network = onnx_parser.read("/path/to/your/model/")
# 生成engine
optimizer = TopsInference.create_optimizer()
# 配置成FP32和FP16混合精度
optimizer.set_build_flag(TopsInference.KFP16_MIX)
engine = optimizer.build(network)
# 执行推理
input_data0 = np.random.randn(1, 1, 32, 1600).astype(np.float16)
input_data1 = np.random.randn(1).astype(np.float16)
outputs = []
future = engine.runV2([input_data0, input_data1])
outputs = future.get()
TopsInference.release_device(handler)
C++混合精度模型推理示例:
// 初始化TopsInference
TopsInference::topsInference_init();
uint32_t cluster_id[] = {0};
auto handler = TopsInference::set_device(0, cluster_id);
// 读取模型
TopsInference::IParser* parser = TopsInference::create_parser(TopsInference::TIF_ONNX);
TopsInference::INetwork* network = nullptr;
network = parser->readModel("/path/to/your/model/");
// 逐层单层设置精度
int32_t layers_num = network->getLayerNum();
for (size_t index = 0; index < layers_num; index++) {
auto layer = network->getLayer(index);
auto layer_type = layer->getType();
if (TopsInference::LayerType::TIF_CONVOLUTION == layer_type) {
layer->setPrecision(TopsInference::DataType::TIF_FP16);
}
}
// 生成engine
TopsInference::IOptimizer* optimizer = TopsInference::create_optimizer();
TopsInference::IOptimizerConfig* optimizer_config = optimizer->getConfig();
optimizer_config->setBuildFlag(TopsInference::BuildFlag::TIF_KTYPE_MIX_FP16);
TopsInference::IEngine* engine = optimizer->build(network);
...
std::vector<std::vector<float>> outs;
std::vector<std::vector<float>> inputs_guad;
...
// 设置输入ITensor
float* inputs[] = {inputs_guad[t].data()};
std::vector<TopsInference::TensorPtr_t> input_tensor_list;
int32_t input_num = engine->getInputNum();
for (size_t i = 0; i < input_num; i++) {
TopsInference::TensorPtr_t sub_input = TopsInference::create_tensor();
// 需要设置输入ITensor的数据
sub_input->setOpaque(reinterpret_cast<void*>(inputs[i]));
// 需要设置输入ITensor的DeviceType
sub_input->setDeviceType(TopsInference::DataDeviceType::HOST);
TopsInference::Dims input_shape = engine->getInputShape(i);
input_shape.dimension[0] = batch_size;
// 需要使用设置输入ITensor的dims
sub_input->setDims(input_shape);
input_tensor_list.emplace_back((TopsInference::TensorPtr_t)sub_input);
}
// 设置输出ITensor
std::vector<TopsInference::TensorPtr_t> output_tensor_list;
int32_t output_num = engine->getOutputNum();
for (size_t i = 0; i < output_num; ++i) {
TopsInference::Dims max_shape = engine->getMaxOutputShape(i);
int64_t element_size = 1;
max_shape.dimension[0] = batch_size;
for (size_t j = 0; j < max_shape.nbDims; ++j) {
element_size *= max_shape.dimension[j];
}
std::vector<float> out(element_size);
outs[t] = out;
TopsInference::TensorPtr_t sub_output = TopsInference::create_tensor();
// 需要设置输出ITensor的数据
sub_output->setOpaque(reinterpret_cast<void*>(out.data()));
// 需要使用maxoutputshape设置输出ITensor的dims,设置为最大输出形状
sub_output->setDims(max_shape);
// 需要设置输出ITensor的DeviceType
sub_output->setDeviceType(TopsInference::DataDeviceType::HOST);
output_tensor_list.emplace_back((TopsInference::TensorPtr_t)sub_output);
}
// 调用runV2,执行推理
engine->runV2(input_tensor_list.data(), output_tensor_list.data(), stream);
...
TopsInference::release_parser(parser);
TopsInference::release_network(network);
TopsInference::release_optimizer(optimizer);
TopsInference::release_engine(engine);
TopsInference::release_device(handler);
TopsInference::topsInference_finish();
D2D动态形状模型推理示例¶
Python模型推理示例:
with TopsInference.device(0, 0):
# 读取模型
onnx_parser = TopsInference.create_parser(TopsInference.ONNX_MODEL)
onnx_parser.set_input_names(["133", "360", "587", "814"])
onnx_parser.set_input_shapes([[1, -1, -1, 64], [1, -1, -1, 128],
[1, -1, -1, 256], [1, -1, -1, 512]])
onnx_parser.set_input_dtypes([
TopsInference.TIF_FLOAT32, TopsInference.TIF_FLOAT32,
TopsInference.TIF_FLOAT32, TopsInference.TIF_FLOAT32
])
network = onnx_parser.read("/path/to/your/model/")
# 生成engine
optimizer = TopsInference.create_optimizer()
# 配置动态形状模型输入的最大和最小形状
max_shape_dim_setting = []
max_shape_dim = {}
max_shape["main"] = [[1,512,512,64], [1,512,512,128],
[1,256,256,256], [1,128,128,512]]
max_shape_dim_setting.append(max_shape_dim)
optimizer.set_max_shape_range(max_shape_dim_setting)
min_shape_dim_setting = []
min_shape_dim = {}
min_shape_dim["main"] = [[1,16,16,64], [1,8,8,128],
[1,4,4,256], [1,2,2,512]]
min_shape_dim_setting.append(min_shape_dim)
optimizer.set_min_shape_range(min_shape_dim_setting)
engine = optimizer.build(network)
np.random.seed(0)
input0 = np.random.randint(0, 100, (1, 512, 512, 64)).astype(np.float32)
input1 = np.random.randint(0, 100, (1, 512, 512, 128)).astype(np.float32)
input2 = np.random.randint(0, 100, (1, 256, 256, 256)).astype(np.float32)
input3 = np.random.randint(0, 100, (1, 128, 128, 512)).astype(np.float32)
stream = TopsInference.create_stream()
# 设置输入
input_num = engine.get_input_num()
inputs = [input0, input1, input2, input3]
p_inputs = []
for ind in range(input_num):
input_size = np.size(np.array(inputs[ind])) * 4
p_inputs.append(TopsInference.mem_alloc(input_size))
p_inputs[ind].set_shape(np.array(inputs[ind]).shape)
TopsInference.mem_h2d_copy_async(inputs[ind], p_inputs[ind], input_size,stream)
# 设置输出
p_outputs = []
output_num = engine.get_output_num()
for ind in range(output_num):
output_size = 4
sub_output_shape = engine.get_max_output_shape(ind)
for d in sub_output_shape:
output_size *= d
p_outputs.append(TopsInference.mem_alloc(output_size))
p_outputs[ind].set_shape(sub_output_shape)
# 执行推理
future = engine.runV2(p_inputs, output_list=p_outputs, py_stream=stream)
outputs = future.get()
host_outputs = []
for ind in range(output_num):
real_buf_size = outputs[ind].get_real_size()
out_shape = outputs[ind].get_real_shape()
# out_shape[0] = 1是根据实际的batch_size配置
# 如果第一维不表示batch_size,可删除
out_shape[0] = 1
host_outputs.append(np.zeros(out_shape, dtype=np.float32))
TopsInference.mem_d2h_copy_async(p_outputs[ind], host_outputs[ind],
real_buf_size, stream)
stream.synchronize()
C++多线程推理¶
本示例展示C++多线程多Cluster并行推理:
#include <stdio.h>
#include <cstring>
#include <iostream>
#include <vector>
#include <tops/tops_runtime.h>
#include "TopsInference/TopsInferRuntime.h"
#include <json/json.h>
#include <thread>
#include <chrono>
#include <unistd.h>
/**
* createInputTensors 创建输入信息.
*
* @param engine 引擎指针 .
* @param input_data 输入数据地址空间 .
* @param input_tensor_list TopsInference runV2 输入集合.
* @param input_data_1 att_cache的动态参数 T_CACHE
* @param input_T chunk动态参数 T
*
*/
void createInputTensors(
TopsInference::IEngine* engine,
std::vector<std::vector<float>>& input_data,
std::vector<TopsInference::TensorPtr_t>& input_tensor_list,
int64_t* input_data_1, int64_t input_T) {
input_data[0].resize(1 * (input_T) * 80);
input_data[1].resize(1);
input_data[2].resize(12 * 8 * (*input_data_1) * 128);
input_data[3].resize(12 * 1 * 512 * 14);
int32_t input_num = engine->getInputNum();
for (int32_t i = 0; i < input_num; i++) {
TopsInference::TensorPtr_t sub_input = TopsInference::create_tensor();
float* inputs[] = {input_data[i].data()};
// 需要设置输入ITensor的数据
if(i==1) sub_input->setOpaque(reinterpret_cast<void*>(input_data_1));
else sub_input->setOpaque(reinterpret_cast<void*>(inputs[0]));
// 需要使用设置输入ITensor的DeviceType
sub_input->setDeviceType(TopsInference::DataDeviceType::HOST);
TopsInference::Dims input_shape = engine->getInputShape(i);
if(i==0) {
input_shape.dimension[0] = 1;
input_shape.dimension[1] = input_T;
input_shape.dimension[2] = 80;
}
else if (i==1) {
input_shape.dimension[0] = 1;
} else if (i==2) {
input_shape.dimension[0] = 12;
input_shape.dimension[1] = 8;
input_shape.dimension[2] = *input_data_1;
input_shape.dimension[3] = 128;
} else if (i==3) {
input_shape.dimension[0] = 12;
input_shape.dimension[1] = 1;
input_shape.dimension[2] = 512;
input_shape.dimension[3] = 14;
}
// 需要使用设置输入ITensor的dims
sub_input->setDims(input_shape);
input_tensor_list.emplace_back(sub_input);
}
}
/**
* setInferenceOutput 配置输出信息.
*
* @param engine 引擎指针 .
* @param outs 输出数据地址空间 .
* @param output_tensor_list TopsInference runV2 输出集合.
*
*/
void setInferenceOutput(
TopsInference::IEngine* engine,
std::vector<std::vector<float>>& outs,
std::vector<TopsInference::TensorPtr_t>& output_tensor_list
) {
int32_t output_num = engine->getOutputNum();
outs.resize(output_num);
for (int32_t i = 0; i < output_num; ++i) {
TopsInference::TensorPtr_t sub_output = TopsInference::create_tensor();
TopsInference::Dims max_shape = engine->getMaxOutputShape(i);
int64_t out_element_size = 1;
for (int32_t j = 0; j < max_shape.nbDims; ++j) {
out_element_size *= max_shape.dimension[j];
}
outs[i].resize(out_element_size);
// 需要设置输出ITensor的数据
sub_output->setOpaque(reinterpret_cast<void*>(outs[i].data()));
// 需要使用maxoutputshape设置输出ITensor的dims,设置为最大输出形状
sub_output->setDims(max_shape);
// 需要设置输出ITensor的DeviceType
sub_output->setDeviceType(TopsInference::DataDeviceType::HOST);
output_tensor_list.emplace_back((TopsInference::TensorPtr_t)sub_output);
}
}
/**
* getOutput 输出处理.
*
* @param engine 引擎指针 .
* @param outs 输出数据地址空间 .
* @param output_tensor_list TopsInference runV2 输出集合.
*
*/
void getOutput(
TopsInference::IEngine* engine,
std::vector<std::vector<float>>& outs,
std::vector<TopsInference::TensorPtr_t>& output_tensor_list){
int32_t output_num = engine->getOutputNum();
for (int32_t i = 0; i < output_num; ++i) {
int64_t real_element_num = 1;
// 需要使用真实的输出形状来获取结果数据
TopsInference::Dims ouput_shape = output_tensor_list[i]->getDims();
for (int32_t index = 0; index < ouput_shape.nbDims; ++index) {
real_element_num *= ouput_shape.dimension[index];
}
outs[i].resize(real_element_num);
}
}
/**
* freeResource 释放资源.
*
* @param engine 引擎指针 .
* @param input_tensor_list TopsInference runV2 输入集合 .
* @param output_tensor_list TopsInference runV2 输出集合.
* @param outs 输出数据地址空间 .
*
*/
void freeResource(TopsInference::IEngine* engine,
std::vector<TopsInference::TensorPtr_t>& input_tensor_list,
std::vector<TopsInference::TensorPtr_t>& output_tensor_list,
std::vector<std::vector<float>>& outs){
// 释放资源
int32_t input_num = engine->getInputNum();
int32_t output_num = engine->getOutputNum();
for (int32_t i = 0; i < input_num; ++i) {
TopsInference::destroy_tensor(input_tensor_list[i]);
}
for (int32_t i = 0; i < output_num; ++i) {
outs[i].clear();
TopsInference::destroy_tensor(output_tensor_list[i]);
}
input_tensor_list.clear();
output_tensor_list.clear();
outs.clear();
std::vector<std::vector<float>>().swap(outs);
}
/**
* run 功能汇总入口函数.
*
*/
void run(int itr_id,
std::vector<std::vector<float>>& input_data,
std::vector<TopsInference::TensorPtr_t>& input_tensor_list,
int64_t* input_data_1,
int64_t input_T,
std::vector<std::vector<float>>& outs,
std::vector<TopsInference::TensorPtr_t>& output_tensor_list) {
uint32_t cluster_id[] = {(uint32_t)itr_id};
auto handler = TopsInference::set_device(0, cluster_id);
TopsInference::IEngine* engine = TopsInference::create_engine();
// 加载模型引擎
engine->loadExecutable("/path/to/your/bin");
createInputTensors(engine, input_data, input_tensor_list, input_data_1, input_T);
setInferenceOutput(engine,outs,output_tensor_list);
// 测试使用,跑多次求平均时间,前几次为warmup
auto start_time = std::chrono::high_resolution_clock::now();
int runtimes = 1000;
for(int i =0 ;i<runtimes;i++) {
// 调用runV2 同步方式
engine->runV2(input_tensor_list.data(), output_tensor_list.data());
if(i==runtimes/2-1) {start_time = std::chrono::high_resolution_clock::now();}
if(i == runtimes-1) {
auto end_time = std::chrono::high_resolution_clock::now();
auto duration = std::chrono::duration_cast<std::chrono::milliseconds>(end_time - start_time);
std::cout << " ********** in run thread " << itr_id << " ********** " <<std::endl;
auto latency = duration.count() / ((runtimes/2) * 1.0);
std::cout << " latency: " << latency << " ms" << std::endl;
std::cout << " fps: " << 1000.0 / latency << " " << std::endl;
std::cout << " ********** ***** ********** " <<std::endl;
}
}
getOutput(engine,outs,output_tensor_list);
freeResource(engine,input_tensor_list,output_tensor_list,outs);
TopsInference::release_engine(engine);
TopsInference::release_device(handler);
delete[] execpath;
}
void TEST() {
// 初始化TopsInference
TopsInference::topsInference_init();
// 设置输入输出
std::vector<std::vector<std::vector<float>>> input_datas(6, std::vector<std::vector<float>>(4));
std::vector<std::vector<TopsInference::TensorPtr_t>> input_tensor_lists(6,std::vector<TopsInference::TensorPtr_t>());
std::vector<std::vector<std::vector<float>>> outs(6,std::vector<std::vector<float>>());
std::vector<std::vector<TopsInference::TensorPtr_t>> output_tensor_lists(6,std::vector<TopsInference::TensorPtr_t>());
// 6个大计算单元
int core_num = 6;
int64_t input_T = 67;
// 设置offset输入值
std::vector<int64_t*> input_vectors(6);
for (int i = 0; i < core_num; ++i) {
input_vectors[i] = new int64_t(32);
}
std::vector<std::thread> threads(core_num);
for (int i = 0; i < core_num; ++i) {
threads[i] = std::thread(&run,i,std::ref(input_datas[i]),std::ref(input_tensor_lists[i]),std::ref(input_vectors[i]),std::ref(input_T),\
std::ref(outs[i]),std::ref(output_tensor_lists[i]));
}
for (int i = 0; i < core_num; ++i) {
threads[i].join();
}
for (int i = 0; i < core_num; ++i) {
delete input_vectors[i];
}
TopsInference::topsInference_finish();
}
int main() {
TEST();
return 0;
}
多进程推理¶
Cluster可以被视为一个单独的推理设备,这也就意味着我们可以针对一些复杂的场景(如多模型推理任务,或多模型同步推理)的开发。
以下是利用Python multiprocessing模块进行多进程推理的例子。
import TopsInference
import time
import numpy as np
from multiprocessing import Process, Queue
# 定义推理函数
def inference_multiprocess(cluster_id, input_queue, output_queue):
with TopsInference.device(0, cluster_id):
engine = TopsInference.load("/path/to/engine")
output = []
while True:
try:
data = input_queue.get(timeout=10)
future = engine.runV2([data])
output = future.get()
output_queue.put(output)
except:
break
def run_multi():
# 假设我们要处理100个数据,则每个Cluster单独负责处理25个
cluster_num = 4
data_num = 25
# 创建queue list
input_queue_list = [Queue(data_num) for i in range(cluster_num)]
output_queue_list = [Queue(data_num) for i in range(cluster_num)]
# 创建推理进程列表
process_list = [Process(target=inference_multiprocess,
args=(i, input_queue_list[i], output_queue_list[i])) for i in range(cluster_num)]
# 启动四个推理进程
for process in process_list:
process.start()
start = time.perf_counter()
for _ in range(data_num):
for i in range(cluster_num):
dummpy_data = np.random.randn(d0, d1, d2, d3).astype(np.float32, order='C')
# 把输入数据放入input queue中
input_queue_list[i].put(dummpy_data)
# 由于推理服务已经开启,因此可以在此获取推理结果
infer_result = output_queue_list[i].get()
end = time.perf_counter()
if __name__ == "__main__":
run_multi()
多任务推理¶
由于每个Cluster可以视为一个单独的计算设备,因此我们可以从 多进程推理 的例子中引申出一些更加有趣也具实用性的应用案例。
以表情检测为例,表情检测在推理上分为两个步骤:
1.检测出图像中所有的人脸。
2.为检测出的人脸进行表情分类。
自然而然地,我们会想到把这两个推理任务放在两个Cluster上分别进行,在不使用多进程推理时这两个推理任务的执行顺序如下:

图 4.12.1 不使用多进程推理时两个推理任务的执行顺序¶
可以发现,在执行其中一个Cluster执行推理任务时,另一个Cluster则需要等待其结束之后才能开始计算。如果我们想要进一步提高任务处理的性能,则可以将这两个任务并行起来,并行之后的执行顺序如下:

图 4.12.2 并行的执行顺序¶
进一步地,因为每个人脸检测和表情分类都有着自己对应的图像预处理和后处理方法,我们可以将任务进一步拆解,将更多的操作并行起来,这样可以最大程度地使所有Cluster都保持在工作状态,从而最大化推理性能。
用户可以参考以下代码进行多模型推理任务的开发:
import TopsInference
import time
import numpy as np
import cv2
import os
from multiprocessing import Process, Queue
# 定义推理函数
def inference(engine_path, cluster_id, input_queue, output_queue):
with TopsInference.device(0, cluster_id):
engine = TopsInference.load("engine_path")
output = []
while True:
try:
data = input_queue.get(timeout=10)
future = engine.runV2([data])
output = future.get()
output_queue.put(output)
except:
break
# 定义人脸检测预处理函数
def preFace(data_path, queue_out):
imgs = os.listdir(data_path)
for img in imgs:
data = cv2.imread(data_path+img)
'''do something
'''
queue_out.put(preface_data)
# 定义人脸检测后处理函数
def postFace(queue_in, queue_out):
inference_result = queue_in.get()
'''do something
'''
queue_out.put(postface_data)
# 定义表情分类预处理函数
def preEmotion(queue_in, queue_out):
postface_data = queue_in.get()
'''do something
'''
queue_out.put(preemo_data)
# 定义表情分类后处理函数
def posEmotion(queue_in):
inference_result = queue_in.get()
'''do something
'''
cv2.imwrite("filename.jpg", postemo_data)
def main():
data_path = "/path/to/your/file"
face_engine = "/path/to/face/engine/"
emotion_engine = "/path/to/emotion/engine/"
queue_list = [Queue(maxsize=10) for i in range(4)]
process_preface = Process(target=preFace, args=(data_path, queue_list[0]))
process_infface = Process(target=inference, args=(face_engine, 0, queue_list[0], queue_list[1]))
process_postface = Process(target=postFace, args=(queue_list[1], queue_list[2]))
process_preemotion = Process(target=preEmotion, args=(queue_list[2], queue_list[3]))
process_infemotion = Process(target=inference, args=(emotion_engine, 1, queue_list[2],
queue_list[3]))
process_postemotion = Process(target=posEmotion, args=(queue_list[3],))
process_list = [process_preface, process_infface, process_postface,
process_preemotion, process_infemotion, process_postemotion]
for process in process_list:
process.start()
for process in process_list:
process.join()
if __name__ == "__main__":
main()
用户自定义JIT算子¶
dtu_sparse_fields_concat_v2_op.cc 代码如下:
#include "dtu_sparse_fields_concat_v2_op.h"
#include "/hlir_op_registry.h"
#include "/shape_config.h"
#include "gcu/op_define/op_define.h"
#include "gcu/op_define/to_factor.h"
namespace factor {
namespace MySparseFieldsConcatV2 {
class GcuSparseFieldsConcatV2Op : public hlir::HlirOpKernel {
public:
using hlir::HlirOpKernel::HlirOpKernel;
int getPriority(const hlir::HlirOpKernelDesc& kernel_description) const override {
if (!hlir::isHlirCustomOp(kernel_description.op)) {
return -1;
}
auto op_and_param =
hlir::getOpDefAndParam(kernel_description.op);
if (!op_and_param.first.isOpType("my_sparse_fields_concat_v2")) {
return -1;
}
auto in_out_shapes =
hlir::getHlirOpInOutShapes(kernel_description.op);
auto input_shapes = in_out_shapes.first;
auto output_shapes = in_out_shapes.second;
auto feature_weight_shape = input_shapes[0].Dimensions();
auto feature_field_shape = input_shapes[1].Dimensions();
auto feature_index_shape = input_shapes[2].Dimensions();
auto output1_shape = output_shapes[0].Dimensions();
auto output2_shape = output_shapes[1].Dimensions();
if (feature_weight_shape.size() != 3 || feature_field_shape.size() != 2 ||
feature_index_shape.size() != 1) {
return -1;
}
auto feature_weight_dtype = input_shapes[0].GetElementType();
auto feature_field_dtype = input_shapes[1].GetElementType();
auto feature_index_dtype = input_shapes[2].GetElementType();
auto output1_dtype = output_shapes[0].GetElementType();
auto output2_dtype = output_shapes[1].GetElementType();
if (feature_weight_dtype != hlir::HlirDataType::F32) {
return -1;
}
if (feature_field_dtype != hlir::HlirDataType::S32) {
return -1;
}
if (feature_index_dtype != hlir::HlirDataType::S32) {
return -1;
}
if (output1_dtype != feature_weight_dtype ||
output2_dtype != feature_weight_dtype) {
return -1;
}
return true;
}
void compile(const hlir::HlirOpKernelContext& context) const override {
DoradoSparseFieldsConcatV2Impl(context);
}
}; // class ctc op end
void myShapeInfer(const std::vector<hlir::TensorShape> inputs, const hlir::HlirOpParam& params,
std::vector<hlir::TensorShape>& outputs) {
auto input_shape = inputs[0];
auto field_shape = inputs[1];
auto index_shape = inputs[2];
::hlir::HlirDataType output_dtype = input_shape.GetElementType();
auto part1_fields = params["part1_fields"].as<std::vector<int64_t>>();
auto part2_fields = params["part2_fields"].as<std::vector<int64_t>>();
int32_t part1_len = part1_fields.size();
int32_t part2_len = part2_fields.size();
assert(input_shape.ndim()==3);
std::vector<int64_t> input_dimensions = input_shape.Dimensions();
hlir::TensorShape::Range input_range = input_shape.GetDimensionsRange();
std::vector<int64_t> output1_dimensions(2);
hlir::TensorShape::Range output1_range;
output1_dimensions.at(0) = hlir::kUnknownDim;
output1_range.first.emplace_back(1);
output1_range.second.emplace_back(2048);
std::vector<int64_t> output2_dimensions(2);
hlir::TensorShape::Range output2_range;
output2_dimensions.at(0) = hlir::kUnknownDim;
output2_range.first.emplace_back(1);
output2_range.second.emplace_back(2048);
if (input_dimensions.at(1) != hlir::kUnknownDim &&
input_dimensions.at(2) != hlir::kUnknownDim) {
int32_t embedding_size = input_dimensions.at(1) * input_dimensions.at(2);
output1_dimensions.at(1) = part1_len * embedding_size;
output1_range.first.emplace_back(output1_dimensions.at(1));
output1_range.second.emplace_back(output1_dimensions.at(1));
output2_dimensions.at(1) = part2_len * embedding_size;
output2_range.first.emplace_back(output2_dimensions.at(1));
output2_range.second.emplace_back(output2_dimensions.at(1));
} else {
output1_dimensions.at(1) = hlir::kUnknownDim;
output1_range.first.emplace_back(1);
output1_range.second.emplace_back(hlir::kMaxDim);
output2_dimensions.at(1) = hlir::kUnknownDim;
output2_range.first.emplace_back(1);
output2_range.second.emplace_back(hlir::kMaxDim);
}
auto out1_shape = hlir::TensorShape(output_dtype, output1_dimensions,
output1_range.second, output1_range.first);
outputs.at(0) = out1_shape;
auto out2_shape = hlir::TensorShape(output_dtype, output2_dimensions,
output2_range.second, output2_range.first);
outputs.at(1) = out2_shape;
}
int64_t field_num = 3;
std::vector<int64_t> part1{};
std::vector<int64_t> part2{1, 2, 3};
REGISTER_HLIR_OP(my_sparse_fields_concat_v2)
.addInputs(3)
.addOutputs(2)
.addParam("fw_field_num", hlir::HlirOpParamVal(field_num))
.addParam("part1_fields", hlir::HlirOpParamVal(part1))
.addParam("part2_fields", hlir::HlirOpParamVal(part2))
.setShapeInfer(myShapeInfer);
REGISTER_HLIR_KERNEL(hlir::Name("custom_call")
.Unique("my_sparse_fields_concat_v2")
.Device(hlir::GCU_DEVICE)
.TypeConstraint({hlir::HlirDataType::F32,
hlir::HlirDataType::S32,
hlir::HlirDataType::F16}),
GcuSparseFieldsConcatV2Op);
} // namespace MySparseFieldsConcatV2
} // namespace factor
dtu_sparse_fields_concat_v2_op_impl.cc 文件代码如下:
#include <string>
#include "/hlir_op_registry.h"
#include "gcu/factor/factor.h"
#include "gcu/factor/func_experimental.h"
#include "gcu/factor/program_experimental.h"
#include "gcu/op_define/op_define.h"
#include "gcu/op_define/to_factor.h"
#include "dtu_sparse_fields_concat_v2_op.h"
#define MAX_BATCH (2048)
#define MAX_FEATURE (65536)
#define CACHE_BATCH (16)
#define tile_batch (128)
#define BATCH_LEN (MAX_BATCH + 2)
#define BATCH_BOUND_LEN (AlignUp(2 * BATCH_LEN, tile_batch))
#define BATCH_COUNT_LEN (AlignUp(BATCH_LEN, 4 * tile_batch))
template <typename Int_Type, typename Int_Type2 = Int_Type>
inline Int_Type CeilDiv(Int_Type x, Int_Type2 y) {
return (x + y - 1) / y;
}
template <typename Int_Type, typename Int_Type2 = Int_Type>
inline Int_Type AlignUp(Int_Type x, Int_Type2 y) {
return CeilDiv(x, y) * y;
}
namespace factor {
namespace MySparseFieldsConcatV2 {
void SparseFieldsConcatV2L3Wrap(std::string func_name,
const hlir::HlirOpKernelContext& context) {
std::string c_func_name_0 = "sparse_fields_concat_v2_cal_aux";
std::string c_func_name_1 = "sparse_fields_concat_v2_merge_batch";
std::string c_func_name_2 = "sparse_fields_concat_v2_merge_field";
std::string c_func_name_2_0 =
"sparse_fields_concat_v2_merge_field_simplified";
std::string c_func_name_3 = "sparse_fields_concat_v2_div_count";
std::string c_func_name_4 = "sparse_fields_concat_v2_inverse";
auto in_out_shapes =
hlir::getHlirOpInOutShapes(context.kernel_desc.op);
auto input_shapes = in_out_shapes.first;
auto output_shapes = in_out_shapes.second;
auto op_and_param =
hlir::getOpDefAndParam(context.kernel_desc.op);
auto runtime_param = op_and_param.second;
auto op = op_and_param.first;
auto fw_field_num =
Cast64To32Bit(runtime_param["fw_field_num"].as<int64_t>());
auto part1_fields = CastVec64ToVec32Bit(
runtime_param["part1_fields"].as<std::vector<int64_t>>());
int32_t part1_field_num = part1_fields.size();
auto part2_fields = CastVec64ToVec32Bit(
runtime_param["part2_fields"].as<std::vector<int64_t>>());
int32_t part2_field_num = part2_fields.size();
std::string part1_merge_field_c_func_name = c_func_name_2;
if (part1_field_num == 1) {
part1_merge_field_c_func_name = c_func_name_2_0;
}
std::string part2_merge_field_c_func_name = c_func_name_2;
if (part2_field_num == 1) {
part2_merge_field_c_func_name = c_func_name_2_0;
}
auto weight_in_shape = input_shapes[0].Dimensions();
auto field_in_shape = input_shapes[1].Dimensions();
auto index_in_shape = input_shapes[2].Dimensions();
auto out1_shape = output_shapes[0].Dimensions();
auto out2_shape = output_shapes[1].Dimensions();
auto ori_weight_in_shape = weight_in_shape;
auto ori_field_in_shape = field_in_shape;
auto ori_index_in_shape = index_in_shape;
auto ori_out1_shape = out1_shape;
auto ori_out2_shape = out2_shape;
auto data_type = input_shapes[0].GetElementType();
auto int32_dtype = IntType(32);
auto float_dtype =
data_type == hlir::HlirDataType::F16 ? FloatType(16) : FloatType(32);
int32_t C_SIP_ALIGN = 32;
int32_t tile_feature = 512;
int32_t tile_main_feature = 64;
int32_t tile_sip_embedding = 32;
int32_t tile_csb_feature = 1024;
assert(tile_sip_embedding = 32);
auto sip_cnt = 4;
assert(sip_cnt == 4);
auto weight_in_hbm_t = DRAMType(float_dtype, ori_weight_in_shape);
auto field_in_hbm_t = DRAMType(int32_dtype, ori_field_in_shape);
auto index_in_hbm_t = DRAMType(int32_dtype, ori_index_in_shape);
auto part1_field2idx_hbm_t = DRAMType(int32_dtype, {fw_field_num});
auto part2_field2idx_hbm_t = DRAMType(int32_dtype, {fw_field_num});
auto part1_fields_hbm_t = DRAMType(int32_dtype, {part1_field_num});
auto part2_fields_hbm_t = DRAMType(int32_dtype, {part2_field_num});
auto out1_hbm_t = DRAMType(float_dtype, ori_out1_shape);
auto out2_hbm_t = DRAMType(float_dtype, ori_out2_shape);
auto index_in_csb_t = SRAMType(int32_dtype, {tile_feature + 1});
auto index_in_sip_t = L1Type(int32_dtype, {tile_feature + 1});
auto batch_bound_sip_t = L1Type(int32_dtype, {BATCH_BOUND_LEN});
auto all_batch_bound_sip_t = L1Type(int32_dtype, {sip_cnt, BATCH_BOUND_LEN});
auto batch_bound_csb_t = SRAMType(int32_dtype, {sip_cnt, BATCH_BOUND_LEN});
auto field_count_sip_t = L1Type(int32_dtype, {BATCH_COUNT_LEN, fw_field_num});
auto field_count_csb_t =
SRAMType(int32_dtype, {sip_cnt, BATCH_COUNT_LEN, fw_field_num});
auto cached_field_in_csb_t = SRAMType(int32_dtype, {MAX_FEATURE, 2});
auto cached_index_in_csb_t = SRAMType(int32_dtype, {MAX_FEATURE});
auto field_in_csb_t = SRAMType(int32_dtype, {tile_feature, 2});
auto field_in_sip_t = L1Type(int32_dtype, {tile_feature, 2});
auto field_in_main_csb_t = SRAMType(int32_dtype, {tile_main_feature, 2});
auto field_in_main_sip_t = L1Type(int32_dtype, {tile_main_feature, 2});
auto weight_in_csb_t =
SRAMType(float_dtype, {tile_main_feature, tile_sip_embedding});
auto cached_weight_in_csb_t =
SRAMType(float_dtype, {tile_csb_feature, tile_sip_embedding});
auto weight_in_sip_t =
L1Type(float_dtype, {tile_main_feature, tile_sip_embedding});
auto part1_field2idx_sip_t = L1Type(int32_dtype, {fw_field_num});
auto part2_field2idx_sip_t = L1Type(int32_dtype, {fw_field_num});
auto part1_fields_sip_t = L1Type(int32_dtype, {part1_field_num});
auto part2_fields_sip_t = L1Type(int32_dtype, {part2_field_num});
auto out1_sip_t =
L1Type(float_dtype, {1, part1_field_num, tile_sip_embedding});
auto out1_csb_t =
SRAMType(float_dtype, {1, part1_field_num, tile_sip_embedding});
auto out2_sip_t =
L1Type(float_dtype, {CACHE_BATCH, part2_field_num, tile_sip_embedding});
auto out2_csb_t =
SRAMType(float_dtype, {CACHE_BATCH, part2_field_num, tile_sip_embedding});
int32_t index_pad_value = BATCH_LEN - 1;
int32_t field_pad_value = 1;
D(func_)
(func_name.c_str(),
{weight_in_hbm_t, field_in_hbm_t, index_in_hbm_t, part1_field2idx_hbm_t,
part2_field2idx_hbm_t, part1_fields_hbm_t, part2_fields_hbm_t},
{out1_hbm_t, out2_hbm_t}, [&](auto args, auto results) {
auto ori_weight_in_hbm = args[0];
auto ori_field_in_hbm = args[1];
auto ori_index_in_hbm = args[2];
auto part1_field2idx_hbm = args[3];
auto part2_field2idx_hbm = args[4];
auto part1_fields_hbm = args[5];
auto part2_fields_hbm = args[6];
auto ori_out1_hbm = results[0];
auto ori_out2_hbm = results[1];
auto sip_idx = thread_id_();
auto index_in_cdma_0 = alloc_dma_(CDMAType());
auto index_in_sdma_0 = alloc_dma_(SDMAType());
auto field_in_cdma_0 = alloc_dma_(CDMAType());
auto field_in_sdma_0 = alloc_dma_(SDMAType());
auto memset_sdma = alloc_dma_(SDMAType());
auto memset_shared_cdma_0 = alloc_dma_(CDMAType()).shared_();
auto memset_shared_cdma_1 = alloc_dma_(CDMAType()).shared_();
auto memset_shared_cdma_2 = alloc_dma_(CDMAType()).shared_();
auto memset_shared_cdma_3 = alloc_dma_(CDMAType()).shared_();
auto memset_sync_sdma = alloc_dma_(SDMAType());
auto shared_cdma = alloc_dma_(CDMAType()).shared_();
auto index_in_csb_0 = alloc_(index_in_csb_t);
auto index_in_sip_0 = alloc_(index_in_sip_t);
auto field_in_csb_0 = alloc_(field_in_csb_t);
auto cached_field_in_csb = alloc_(cached_field_in_csb_t).shared_();
auto cached_index_in_csb = alloc_(cached_index_in_csb_t).shared_();
auto field_in_sip_0 = alloc_(field_in_sip_t);
auto batch_bound_sip = alloc_(batch_bound_sip_t);
auto batch_begin_sip =
memview_(batch_bound_sip, {0}, {BATCH_BOUND_LEN / 2});
auto batch_end_sip = memview_(batch_bound_sip, {BATCH_BOUND_LEN / 2},
{BATCH_BOUND_LEN / 2});
auto all_batch_bound_sip = alloc_(all_batch_bound_sip_t);
auto field_count_sip = alloc_(field_count_sip_t);
auto batch_bound_csb = alloc_(batch_bound_csb_t).shared_();
auto part2_batch_num_sip = alloc_(L1Type(int32_dtype, {1}));
auto field_count_csb = alloc_(field_count_csb_t).shared_();
auto run_feature_size = dim_(ori_weight_in_hbm, 0);
auto run_embedding_size =
dim_(ori_weight_in_hbm, 1) * dim_(ori_weight_in_hbm, 2);
auto weight_in_hbm =
bitcast_(DRAMType(float_dtype, {-1, -1}), ori_weight_in_hbm,
{run_feature_size, run_embedding_size});
auto field_in_hbm = bitcast_(DRAMType(int32_dtype, {-1, -1}),
ori_field_in_hbm, {run_feature_size, 2});
auto index_in_hbm = bitcast_(DRAMType(int32_dtype, {-1}), ori_index_in_hbm,
{run_feature_size + 1});
assert_(dim_(ori_field_in_hbm, 0) == run_feature_size,
"feature_field's shape should be [feature_size, 2]");
assert_(dim_(ori_field_in_hbm, 1) == 2,
"feature_field's shape should be [feature_size, 2]");
assert_(logical_or_(dim_(ori_index_in_hbm, 0) - 1 == 0,
dim_(ori_index_in_hbm, 0) - 1 == run_feature_size),
"feature_index's shape should be [feature_size]");
auto cached_index_in_csb_mv =
memview_(cached_index_in_csb, {0}, {run_feature_size + 1});
auto cached_index_in_csb_bc =
bitcast_(SRAMType(IntType(32), {-1}), cached_index_in_csb_mv,
{run_feature_size + 1});
load_(shared_cdma, index_in_hbm, cached_index_in_csb_bc, {0});
syncthreads_();
auto request_batch_size_sip = alloc_(L1Type(IntType(32), {1}));
load_(index_in_sdma_0, cached_index_in_csb, request_batch_size_sip,
{run_feature_size});
auto request_batch_size = request_batch_size_sip(0);
assert_(request_batch_size <= MAX_BATCH,
"no support batch size too large");
auto out1_hbm =
bitcast_(DRAMType(float_dtype, {-1, part1_field_num, -1}),
ori_out1_hbm, {request_batch_size, run_embedding_size});
auto out2_hbm =
bitcast_(DRAMType(float_dtype, {-1, part2_field_num, -1}),
ori_out2_hbm, {request_batch_size, run_embedding_size});
if (ori_out1_shape[0] == -1 && ori_out1_shape[1] == -1) {
update_shape_info_(
ori_out1_hbm,
{request_batch_size, Value(part1_field_num) * run_embedding_size});
} else {
update_shape_info_(ori_out1_hbm, {request_batch_size});
}
if (ori_out2_shape[0] == -1 && ori_out2_shape[1] == -1) {
update_shape_info_(
ori_out2_hbm,
{request_batch_size, Value(part2_field_num) * run_embedding_size});
} else {
update_shape_info_(ori_out2_hbm, {request_batch_size});
}
if_(
logical_and_(run_embedding_size > 0, run_feature_size > 0),
[&]() {
auto field_in_hbm_bc =
bitcast_(DRAMType(IntType(32), {-1}), field_in_hbm,
{run_feature_size * 2});
auto cached_field_in_csb_mv =
memview_(cached_field_in_csb, {0, 0}, {run_feature_size, 2});
auto cached_field_in_csb_bc =
bitcast_(SRAMType(IntType(32), {-1}), cached_field_in_csb_mv,
{run_feature_size * 2});
load_(shared_cdma, field_in_hbm_bc, cached_field_in_csb_bc, {0});
syncthreads_();
auto feature_per_sip =
(((run_feature_size + sip_cnt - 1) / sip_cnt) + tile_feature -
1) /
tile_feature * tile_feature;
auto feature_begin = feature_per_sip * sip_idx;
auto feature_end =
select_(feature_begin + feature_per_sip > run_feature_size,
run_feature_size, feature_begin + feature_per_sip);
async_memset_(memset_shared_cdma_0, batch_bound_csb, 0);
async_memset_(memset_shared_cdma_1, field_count_csb, 0);
if (part1_field_num > 0) {
async_memset_(memset_shared_cdma_2, out1_hbm, 0.0f);
}
if (part2_field_num > 0) {
async_memset_(memset_shared_cdma_3, out2_hbm, 0.0f);
}
memset_(memset_sdma, batch_bound_sip, 0);
memset_(memset_sync_sdma, field_count_sip, 0);
auto load_field_in = [&](const Value& field_in_sdma,
const Value& field_in_sip,
const Value& feature_idx,
const Value& cur_tile_feature,
const Value& feature_bound) {
auto pad_len =
select_(feature_idx + (cur_tile_feature)-feature_bound > 0,
feature_idx + (cur_tile_feature)-feature_bound, 0);
auto field_in_csb_mv =
memview_(cached_field_in_csb, {feature_idx, 0},
{Value(cur_tile_feature) - pad_len, 2});
async_load_(field_in_sdma, field_in_csb_mv, field_in_sip, {0, 0},
{0, 1}, {0, 0}, {pad_len, 0}, {0, 0}, field_pad_value);
};
auto load_index_in =
[&](const Value& index_in_sdma, const Value& index_in_sip,
const Value& feature_idx, const Value& cur_tile_feature,
const Value& feature_bound) {
// offset,layout,low_pad,mid_pad,high_pad,pad_value
auto pad_len = select_(
feature_idx + (cur_tile_feature + 1) - feature_bound > 0,
feature_idx + (cur_tile_feature + 1) - feature_bound, 0);
auto index_in_csb_mv =
memview_(cached_index_in_csb, {feature_idx},
{Value(cur_tile_feature + 1) - pad_len});
async_load_(index_in_sdma, index_in_csb_mv, index_in_sip, {0},
{0}, {0}, {pad_len}, {0}, index_pad_value);
};
batch_begin_sip[{0}] = 0;
var_ next_batch(IntType(32));
var_ cur_batch(IntType(32));
var_ cur_field(IntType(32));
auto sync_sdma = alloc_dma_(SDMAType());
auto sync_sdma_1 = alloc_dma_(SDMAType());
auto sync_sdma_2 = alloc_dma_(SDMAType());
auto sync_sdma_3 = alloc_dma_(SDMAType());
auto sync_sdma_4 = alloc_dma_(SDMAType());
auto sync_sdma_5 = alloc_dma_(SDMAType());
for_(feature_begin, feature_end, tile_feature,
[&](auto feature_idx) {
load_index_in(index_in_sdma_0, index_in_sip_0, feature_idx,
tile_feature, run_feature_size);
load_field_in(field_in_sdma_0, field_in_sip_0, feature_idx,
tile_feature, run_feature_size);
auto cur_index_in_sip = index_in_sip_0;
auto cur_index_in_sdma = index_in_sdma_0;
wait_dma_(index_in_sdma_0);
auto cur_field_in_sdma = field_in_sdma_0;
auto cur_field_in_sip = field_in_sip_0;
wait_dma_(field_in_sdma_0);
call_(c_func_name_0.c_str(),
cast_(IntType(32), cur_index_in_sip.addr_()),
cast_(IntType(32), cur_field_in_sip.addr_()),
cast_(IntType(32), batch_begin_sip.addr_()),
cast_(IntType(32), batch_end_sip.addr_()),
cast_(IntType(32), field_count_sip.addr_()),
tile_feature, feature_idx, fw_field_num);
});
wait_dma_(memset_shared_cdma_0);
wait_dma_(memset_shared_cdma_1);
if (part1_field_num > 0) {
wait_dma_(memset_shared_cdma_2);
}
if (part2_field_num > 0) {
wait_dma_(memset_shared_cdma_3);
}
// syncthreads_() to merge batch_bound_sip
// since workload is small, do not worth too much effort to optimize
syncthreads_();
auto batch_bound_sip_bc = bitcast_(
L1Type(int32_dtype, {1, BATCH_BOUND_LEN}), batch_bound_sip);
auto field_count_sip_bc =
bitcast_(L1Type(int32_dtype, {1, BATCH_COUNT_LEN, fw_field_num}),
field_count_sip);
// only valid sip
if_(feature_begin < feature_end, [&]() {
store_(sync_sdma_1, batch_bound_sip_bc, batch_bound_csb,
{sip_idx, 0});
store_(sync_sdma_3, field_count_sip_bc, field_count_csb,
{sip_idx, 0, 0});
});
syncthreads_();
load_(sync_sdma_4, batch_bound_csb, all_batch_bound_sip, {0, 0});
call_(c_func_name_1.c_str(), cast_(IntType(32), batch_bound_sip.addr_()),
cast_(IntType(32), all_batch_bound_sip.addr_()), sip_cnt,
BATCH_BOUND_LEN);
auto all_field_count_tile_sip =
alloc_(L1Type(int32_dtype, {sip_cnt, tile_batch, fw_field_num}));
for_(sip_idx * (BATCH_COUNT_LEN / 4),
sip_idx * (BATCH_COUNT_LEN / 4) + (BATCH_COUNT_LEN / 4),
tile_batch, [&](auto batch_i) {
load_(sync_sdma_5, field_count_csb, all_field_count_tile_sip,
{0, batch_i, 0});
auto field_count_sip_mv =
memview_(field_count_sip, {batch_i, 0},
{tile_batch, fw_field_num});
call_(c_func_name_1.c_str(),
cast_(IntType(32), field_count_sip_mv.addr_()),
cast_(IntType(32), all_field_count_tile_sip.addr_()),
sip_cnt, tile_batch * fw_field_num);
call_(c_func_name_4.c_str(),
cast_(IntType(32), field_count_sip_mv.addr_()),
cast_(IntType(32), field_count_sip_mv.addr_()),
tile_batch * fw_field_num);
});
syncthreads_();
auto field_count_sip_mv = memview_(
field_count_sip_bc, {0, sip_idx * (BATCH_COUNT_LEN / 4), 0},
{1, BATCH_COUNT_LEN / 4, fw_field_num});
store_(sync_sdma_5, field_count_sip_mv, field_count_csb,
{0, sip_idx * BATCH_COUNT_LEN / 4, 0});
syncthreads_();
load_(sync_sdma_5, field_count_csb, field_count_sip_bc, {0, 0, 0});
auto part1_batch_num =
select_(batch_end_sip(0) - batch_begin_sip(0) > 0, 1, 0);
load_(sync_sdma_2, cached_index_in_csb, part2_batch_num_sip,
{run_feature_size - 1});
auto part2_batch_num = part2_batch_num_sip(0) + 1;
if_(part2_batch_num > 0,
[&]() { batch_end_sip[{part2_batch_num}] = run_feature_size; });
auto common_feature_begin = batch_begin_sip(0);
auto common_feature_end = batch_end_sip(0);
auto weight_in_cdma = alloc_dma_(CDMAType());
auto weight_in_sdma = alloc_dma_(SDMAType());
auto out1_sdma = alloc_dma_(SDMAType());
auto out1_cdma = alloc_dma_(CDMAType());
auto weight_in_csb = alloc_(weight_in_csb_t);
auto cached_weight_in_csb = alloc_(cached_weight_in_csb_t);
auto weight_in_sip = alloc_(weight_in_sip_t);
auto out1_sip = alloc_(out1_sip_t);
auto out1_csb = alloc_(out1_csb_t);
auto part1_field2idx_sip = alloc_(part1_field2idx_sip_t);
load_(sync_sdma, part1_field2idx_hbm, part1_field2idx_sip, {0});
auto part2_field2idx_sip = alloc_(part2_field2idx_sip_t);
load_(sync_sdma, part2_field2idx_hbm, part2_field2idx_sip, {0});
auto part1_fields_sip = alloc_(part1_fields_sip_t);
auto part2_fields_sip = alloc_(part2_fields_sip_t);
auto field_in_main_csb = alloc_(field_in_main_csb_t);
auto field_in_main_sip = alloc_(field_in_main_sip_t);
if (part1_field_num > 0) {
load_(sync_sdma, part1_fields_hbm, part1_fields_sip, {0});
for_(
sip_idx * tile_sip_embedding, run_embedding_size,
sip_cnt * tile_sip_embedding, [&](auto embedding_idx) {
if_(common_feature_begin < common_feature_end,
[&]() { async_memset_(memset_sdma, out1_sip, 0.0f); });
for_(common_feature_begin, common_feature_end,
tile_main_feature, [&](auto feature_idx) {
load_field_in(field_in_sdma_0, field_in_main_sip,
feature_idx, tile_main_feature,
common_feature_end);
async_load_(weight_in_cdma, weight_in_hbm,
weight_in_csb,
{feature_idx, embedding_idx})
.notify_(weight_in_sdma);
auto act_feature_num =
select_(common_feature_end - feature_idx <
tile_main_feature,
common_feature_end - feature_idx,
tile_main_feature);
auto weight_in_csb_mv =
memview_(weight_in_csb, {0, 0},
{act_feature_num, tile_sip_embedding});
async_load_(weight_in_sdma, weight_in_csb_mv,
weight_in_sip, {0, 0})
.wait_on_(weight_in_cdma);
if_(feature_idx == common_feature_begin,
[&]() { wait_dma_(memset_sdma); });
wait_dma_(weight_in_sdma);
wait_dma_(field_in_sdma_0);
call_(part1_merge_field_c_func_name.c_str(),
cast_(IntType(32), weight_in_sip.addr_()),
cast_(IntType(32), field_in_main_sip.addr_()),
cast_(IntType(32), part1_field2idx_sip.addr_()),
cast_(IntType(32), out1_sip.addr_()),
tile_main_feature, tile_sip_embedding);
});
call_(c_func_name_3.c_str(),
cast_(IntType(32), field_count_sip.addr_()),
cast_(IntType(32), out1_sip.addr_()),
cast_(IntType(32), part1_fields_sip.addr_()),
part1_field_num, tile_sip_embedding);
store_(out1_sdma, out1_sip, out1_csb, {0, 0, 0});
store_(out1_cdma, out1_csb, out1_hbm, {0, 0, embedding_idx});
});
if_(logical_and_(request_batch_size > 1,
common_feature_end > common_feature_begin),
[&]() {
syncthreads_();
auto out1_hbm_bc =
bitcast_(DRAMType(float_dtype, {-1, -1}),
ori_out1_hbm,
{request_batch_size, Value(part1_field_num) *
run_embedding_size});
auto out1_hbm_single_batch = memview_(
out1_hbm_bc, {0, 0},
{1, Value(part1_field_num) * run_embedding_size});
auto out1_hbm_rest_batch =
memview_(out1_hbm_bc, {1, 0},
{request_batch_size - 1,
Value(part1_field_num) * run_embedding_size});
if_(sip_idx == 0, [&]() {
if_(
request_batch_size == 2,
[&]() {
store_(out1_cdma, out1_hbm_single_batch,
out1_hbm_rest_batch, {0, 0});
},
[&]() {
broadcast_(out1_hbm_single_batch,
out1_hbm_rest_batch);
});
});
});
}
// sample part
if (part2_field_num > 0) {
auto out2_sdma = alloc_dma_(SDMAType());
auto out2_cdma = alloc_dma_(CDMAType());
auto out2_sip = alloc_(out2_sip_t);
auto out2_csb = alloc_(out2_csb_t);
auto samples_feature_begin =
select_(part2_batch_num > 0, batch_end_sip(0), 0);
auto samples_feature_end = select_(
part2_batch_num > 0, batch_end_sip(part2_batch_num), 0);
var_ cur_batch_idx(IntType(32));
var_ cur_feature_idx(IntType(32));
auto weight_in_cdma_0 = alloc_dma_(CDMAType());
auto cached_weight_in_csb_0 = alloc_(cached_weight_in_csb_t);
auto weight_in_cdma_1 = alloc_dma_(CDMAType());
auto cached_weight_in_csb_1 = alloc_(cached_weight_in_csb_t);
var_ cur_pang(IntType(32));
var_ need_wait(IntType(32));
var_ cur_loop_idx(IntType(32));
cur_loop_idx = -1;
need_wait = 0;
load_(sync_sdma, part2_fields_hbm, part2_fields_sip, {0});
memset_(memset_sdma, out2_sip, 0.0f);
if_(logical_and_(sip_idx * tile_sip_embedding <
run_embedding_size,
samples_feature_begin <
samples_feature_end),
[&]() {
async_load_(
weight_in_cdma_0, weight_in_hbm, cached_weight_in_csb_0,
{samples_feature_begin, sip_idx * tile_sip_embedding});
});
for_(
sip_idx * tile_sip_embedding, run_embedding_size,
sip_cnt * tile_sip_embedding, [&](auto embedding_idx) {
cur_feature_idx = samples_feature_begin;
cur_batch_idx = 0;
for_(
samples_feature_begin, samples_feature_end,
tile_csb_feature, [&](auto csb_feature_idx) {
cur_loop_idx = cur_loop_idx + 1;
cur_pang = bitwise_and_(cur_loop_idx, 0x1);
// not logical_and_(csb_feature_idx + tile_csb_feature
// >=
// samples_feature_end,
// embedding_idx + sip_cnt *
// tile_sip_embedding >=
// run_embedding_size))
if_(logical_or_(
csb_feature_idx + tile_csb_feature <
samples_feature_end,
embedding_idx + sip_cnt * tile_sip_embedding <
run_embedding_size),
[&]() {
auto next_weight_in_cdma =
select_(cur_pang == 1, weight_in_cdma_0,
weight_in_cdma_1);
auto next_cached_weight_in_csb = select_(
cur_pang == 1, cached_weight_in_csb_0,
cached_weight_in_csb_1);
auto next_csb_feature_idx =
select_(csb_feature_idx + tile_csb_feature >=
samples_feature_end,
samples_feature_begin,
csb_feature_idx + tile_csb_feature);
auto next_embedding_idx = select_(
csb_feature_idx + tile_csb_feature >=
samples_feature_end,
embedding_idx +
sip_cnt * tile_sip_embedding,
embedding_idx);
async_load_(
next_weight_in_cdma, weight_in_hbm,
next_cached_weight_in_csb,
{next_csb_feature_idx, next_embedding_idx});
});
auto cur_weight_in_cdma = select_(
cur_pang == 0, weight_in_cdma_0, weight_in_cdma_1);
auto cur_cached_weight_in_csb =
select_(cur_pang == 0, cached_weight_in_csb_0,
cached_weight_in_csb_1);
wait_dma_(cur_weight_in_cdma);
while_(
[&]() {
return cur_feature_idx <
min_(csb_feature_idx + tile_csb_feature,
samples_feature_end);
},
[&]() {
auto sample_feature_end =
batch_end_sip(cur_batch_idx + 1);
auto out2_sip_mv = memview_(
out2_sip,
{cur_batch_idx % CACHE_BATCH, 0, 0},
{1, part2_field_num, tile_sip_embedding});
for_(
cur_feature_idx,
min_(csb_feature_idx + tile_csb_feature,
sample_feature_end),
tile_main_feature, [&](auto feature_idx) {
load_field_in(
field_in_sdma_0, field_in_main_sip,
feature_idx, tile_main_feature,
min_(
csb_feature_idx +
tile_csb_feature,
sample_feature_end));
auto act_feature_num = select_(
min_(
csb_feature_idx +
tile_csb_feature,
sample_feature_end) -
feature_idx <
tile_main_feature,
min_(
csb_feature_idx +
tile_csb_feature,
sample_feature_end) -
feature_idx,
tile_main_feature);
auto cached_weight_in_csb_mv = memview_(
cur_cached_weight_in_csb,
{feature_idx - csb_feature_idx, 0},
{act_feature_num, tile_sip_embedding});
async_load_(weight_in_sdma,
cached_weight_in_csb_mv,
weight_in_sip, {0, 0});
if_(need_wait == 1, [&]() {
wait_dma_(memset_sdma);
need_wait = 0;
});
wait_dma_(field_in_sdma_0);
wait_dma_(weight_in_sdma);
call_(part2_merge_field_c_func_name.c_str(),
cast_(IntType(32),
weight_in_sip.addr_()),
cast_(IntType(32),
field_in_main_sip.addr_()),
cast_(IntType(32),
part2_field2idx_sip.addr_()),
cast_(IntType(32),
out2_sip_mv.addr_()),
tile_main_feature,
tile_sip_embedding);
});
if_(cur_feature_idx >=
min_(csb_feature_idx + tile_csb_feature,
sample_feature_end),
[&]() {
if_(need_wait == 1, [&]() {
wait_dma_(memset_sdma);
need_wait = 0;
});
});
if_(sample_feature_end <=
csb_feature_idx + tile_csb_feature,
[&]() {
auto field_count_sip_mv =
memview_(field_count_sip,
{cur_batch_idx + 1, 0},
{1, fw_field_num});
call_(c_func_name_3.c_str(),
cast_(IntType(32),
field_count_sip_mv.addr_()),
cast_(IntType(32),
out2_sip_mv.addr_()),
cast_(IntType(32),
part2_fields_sip.addr_()),
part2_field_num, tile_sip_embedding);
if_(cur_batch_idx % CACHE_BATCH ==
CACHE_BATCH - 1,
[&]() {
async_store_(out2_sdma, out2_sip,
out2_csb, {0, 0, 0})
.notify_(out2_cdma);
async_store_(out2_cdma, out2_csb,
out2_hbm,
{cur_batch_idx -
(CACHE_BATCH - 1),
0, embedding_idx})
.wait_on_(out2_sdma)
.notify_(memset_sdma);
async_memset_(memset_sdma, out2_sip,
0.0f)
.wait_on_(out2_cdma);
need_wait = 1;
});
cur_batch_idx = cur_batch_idx + 1;
});
// only update feature_idx if batch has valid
// data
if_(cur_feature_idx <
min_(csb_feature_idx + tile_csb_feature,
sample_feature_end),
[&] {
cur_feature_idx = min_(
csb_feature_idx + tile_csb_feature,
sample_feature_end);
});
});
});
if_(cur_batch_idx % CACHE_BATCH != 0, [&]() {
async_store_(out2_sdma, out2_sip, out2_csb, {0, 0, 0})
.notify_(out2_cdma);
async_store_(out2_cdma, out2_csb, out2_hbm,
{cur_batch_idx - cur_batch_idx % CACHE_BATCH,
0, embedding_idx})
.wait_on_(out2_sdma)
.notify_(memset_sdma);
async_memset_(memset_sdma, out2_sip, 0.0f)
.wait_on_(out2_cdma);
need_wait = 1;
});
});
if_(need_wait == 1, [&]() {
wait_dma_(memset_sdma);
need_wait = 0;
});
}
},
[&] {
if (part1_field_num > 0) {
memset_(memset_shared_cdma_2, out1_hbm, 0.0f);
}
if (part2_field_num > 0) {
memset_(memset_shared_cdma_3, out2_hbm, 0.0f);
}
}); // end of embedding_size > 0 && run_feature_size > 0 guard
});
c_func_(
c_func_name_1.c_str(), {IntType(32), IntType(32), IntType(32), IntType(32)}, {},
#include "../../build/merge_batch.inc"
); // NOLINT
c_func_(c_func_name_0.c_str(),
{IntType(32), IntType(32), IntType(32), IntType(32), IntType(32),
IntType(32), IntType(32), IntType(32)},
{},
#include "../../build/cal_aux.inc"
); // NOLINT
c_func_(c_func_name_2.c_str(),
{IntType(32), IntType(32), IntType(32), IntType(32), IntType(32),
IntType(32)},
{},
#include "../../build/merge_field.inc"
); // NOLINT
c_func_(c_func_name_2_0.c_str(),
{IntType(32), IntType(32), IntType(32), IntType(32), IntType(32),
IntType(32)},
{},
#include "../../build/merge_field_simplified.inc"
); // NOLINT
c_func_(c_func_name_3.c_str(),
{IntType(32), IntType(32), IntType(32), IntType(32), IntType(32)}, {},
#include "../../build/div_count.inc"
); // NOLINT
c_func_(c_func_name_4.c_str(), {IntType(32), IntType(32), IntType(32)}, {},
#include "../../build/inverse.inc"
); // NOLINT
}
void DoradoSparseFieldsConcatV2Impl(
const hlir::HlirOpKernelContext& context) {
auto op = context.kernel_desc.op;
std::string func_name = std::string(context.identify) + "sparse_fileds_concat_v2";
auto sip_cnt = 4;
std::vector<std::vector<::factor::Value>> inputs;
std::vector<std::vector<::factor::Value>> outputs;
for (hlir::HlirValue value : context.inputs) {
inputs.push_back(hlir::toFactorValue(value));
}
for (hlir::HlirValue value : context.outputs) {
outputs.push_back(hlir::toFactorValue(value));
}
auto weight_in = inputs[0][0];
auto field_in = inputs[1][0];
auto index_in = inputs[2][0];
auto out1 = outputs[0][0];
auto out2 = outputs[1][0];
auto op_and_param =
hlir::getOpDefAndParam(context.kernel_desc.op);
auto runtime_param = op_and_param.second;
auto fw_field_num =
Cast64To32Bit(runtime_param["fw_field_num"].as<int64_t>());
auto part1_fields = CastVec64ToVec32Bit(
runtime_param["part1_fields"].as<std::vector<int64_t>>());
int32_t part1_field_num = part1_fields.size();
auto part2_fields = CastVec64ToVec32Bit(
runtime_param["part2_fields"].as<std::vector<int64_t>>());
int32_t part2_field_num = part2_fields.size();
auto int32_dtype = IntType(32);
auto gen_field2idx_uint8_data =
[](std::vector<int32_t> field_data,
int32_t fw_field_num) -> std::vector<uint8_t> {
int32_t field_num = field_data.size();
std::vector<int32_t> field2idx_data(fw_field_num);
std::vector<uint8_t> field2idx_uint8_data(fw_field_num * 4);
int32_t idx = 0;
for (size_t i = 0; i < field_num; ++i) {
field2idx_data.at(field_data.at(i) - 1) = i;
}
for (size_t i = 0; i < fw_field_num * 4; ++i) {
field2idx_uint8_data.at(i) =
((reinterpret_cast<uint8_t*>(field2idx_data.data()))[i]);
}
return field2idx_uint8_data;
};
auto gen_fields_uint8_data =
[](std::vector<int32_t> fields) -> std::vector<uint8_t> {
int32_t size = fields.size();
std::vector<uint8_t> uint8_fields(size * 4);
for (size_t i = 0; i < size * 4; ++i) {
uint8_fields.at(i) = ((reinterpret_cast<uint8_t*>(fields.data()))[i]);
}
return uint8_fields;
};
auto part1_field2idx_hbm_t = DRAMType(int32_dtype, {fw_field_num});
auto part2_field2idx_hbm_t = DRAMType(int32_dtype, {fw_field_num});
auto part1_field2idx_hbm = alloc_(part1_field2idx_hbm_t);
auto part2_field2idx_hbm = alloc_(part2_field2idx_hbm_t);
auto part1_fields_hbm_t = DRAMType(int32_dtype, {part1_field_num});
auto part2_fields_hbm_t = DRAMType(int32_dtype, {part2_field_num});
auto part1_fields_hbm = alloc_(part1_fields_hbm_t);
auto part2_fields_hbm = alloc_(part2_fields_hbm_t);
std::vector<int32_t> part1_fields_vec, part2_fields_vec;
for (auto field : part1_fields) {
part1_fields_vec.push_back(field);
}
for (auto field : part2_fields) {
part2_fields_vec.push_back(field);
}
const_(gen_field2idx_uint8_data(part1_fields_vec, fw_field_num),
part1_field2idx_hbm);
const_(gen_field2idx_uint8_data(part2_fields_vec, fw_field_num),
part2_field2idx_hbm);
const_(gen_fields_uint8_data(part1_fields_vec), part1_fields_hbm);
const_(gen_fields_uint8_data(part2_fields_vec), part2_fields_hbm);
SparseFieldsConcatV2L3Wrap(func_name, context);
auto task = cg_(
[&]() {
for (int32_t i = 0; i < sip_cnt; ++i) {
launch_(func_name.c_str(),
{weight_in, field_in, index_in, part1_field2idx_hbm,
part2_field2idx_hbm, part1_fields_hbm, part2_fields_hbm},
{out1, out2}, OnEngine::SIP, 0, i);
}
},
false, 0);
wait_cg_(task);
}
} // namespace MySparseFieldsConcatV2
} // namespace factor
JIT的Python测试代码:
import os
import TopsInference
import pytest
from math import pi
import numpy as np
from TopsInference import jit_op_compile_tools # type: ignore
import onnx
import shutil
import onnxruntime
def build_and_clean(test_func):
def wrapper():
cur_dir_path = os.path.dirname(os.path.abspath(__file__))
build_path = jit_op_compile_tools._get_build_dir(os.path.join("custom_jit_op", "build"))
if os.path.exists(build_path):
shutil.rmtree(build_path, ignore_errors=True)
extra_include_paths = [os.path.join(cur_dir_path)]
extra_ld_flags = []
ld_path = os.environ.get("LD_LIBRARY_PATH")
if ld_path != None:
ld_dirs = ld_path.split(":")
for ld_dir in ld_dirs:
if os.path.exists(ld_dir) and os.path.isdir(ld_dir):
for lib in os.listdir(ld_dir):
if "dtu_sdk" in lib:
extra_ld_flags += [
"-L{} -Wl,-rpath,{}".format(ld_dir, ld_dir)
]
break
custom_opsrc = [
os.path.join(cur_dir_path,
"dtu_sparse_fields_concat_v2_op.cc"),
os.path.join(cur_dir_path,
"dtu_sparse_fields_concat_v2_op_impl.cc")
]
custom_opkernel_src = [
os.path.join(cur_dir_path,
"sparse_fields_concat_v2_legacy", "cal_aux.cc"),
os.path.join(cur_dir_path,
"sparse_fields_concat_v2_legacy", "div_count.cc"),
os.path.join(cur_dir_path,
"sparse_fields_concat_v2_legacy", "inverse.cc"),
os.path.join(cur_dir_path,
"sparse_fields_concat_v2_legacy", "merge_batch.cc"),
os.path.join(cur_dir_path,
"sparse_fields_concat_v2_legacy", "merge_field.cc"),
os.path.join(cur_dir_path,
"sparse_fields_concat_v2_legacy", "merge_field_simplified.cc")
]
try:
_ = jit_op_compile_tools.build_and_load(
"my_sparse_fields_concat_v2",
custom_opsrc,
custom_opkernel_src,
extra_include_paths=extra_include_paths,
extra_ldflags=extra_ld_flags,
build_dir=build_path,
verbose=True,
)
test_func()
finally:
pass
return wrapper
np.set_printoptions(threshold=np.inf)
GEN_ONNX ="./tmp.onnx"
BIN_FILE_NAME = "./tmp.bin"
def cosine_similarity(eva, ref):
eva = eva.flatten()
ref = ref.flatten()
return eva.dot(ref) / (np.linalg.norm(eva) * np.linalg.norm(ref))
def compare_results(eva,ref, precision=1e-5,cos_dist=0.99999):
eva = eva.astype(np.float64)
ref = ref.astype(np.float64)
allclose_passed = np.allclose(
eva,
ref,
rtol=precision,
atol=precision,
equal_nan=False)
print("allclose?", allclose_passed)
actual_cos_sim = cosine_similarity(eva, ref)
print("act_cos_sim:{}, threshold:{}".format(actual_cos_sim, cos_dist))
cos_sim_passed = actual_cos_sim > cos_dist
assert(allclose_passed)
return allclose_passed or cos_sim_passed
def get_golden_outputs(input_datas, fw_field_num, part1_field_num, part2_field_num, part1_fields, part2_fields):
weight_in = input_datas[0]
field_in = input_datas[1]
index_in = input_datas[2]
request_batch = index_in[-1]
feature_size = weight_in.shape[0]
embedding_size = weight_in.shape[1] * weight_in.shape[2]
weight_in = np.reshape(weight_in, [feature_size,embedding_size])
common_out = np.zeros([1, part1_field_num, embedding_size]).astype(np.float32)
samples_out = np.zeros([request_batch, part2_field_num, embedding_size]).astype(np.float32)
total_field_count = np.zeros([request_batch + 1, fw_field_num])
for feature_i in range(feature_size):
cur_batch = index_in[feature_i]
cur_field = field_in[feature_i, 1]
if cur_batch >= -1 and cur_batch < request_batch:
total_field_count[cur_batch + 1, cur_field - 1] = total_field_count[cur_batch + 1, cur_field -1] + 1
for feature_i in range(feature_size):
cur_batch = index_in[feature_i]
cur_field = field_in[feature_i, 1]
is_common = True if cur_batch == -1 else False
field_count = total_field_count[cur_batch + 1, cur_field -1]
scale = 1.0 / field_count
cur_weight = weight_in[feature_i, :]
if is_common:
field_idx = (np.where(part1_fields == cur_field))[0]
common_out[0, field_idx, :] = common_out[0, field_idx, :] + scale * cur_weight
else:
field_idx = (np.where(part2_fields == cur_field))[0]
samples_out[cur_batch, field_idx, :] = samples_out[cur_batch, field_idx, :] + scale * cur_weight
common_out = np.reshape(common_out, [1, part1_field_num * embedding_size])
common_out = np.repeat(common_out, request_batch, axis=0)
samples_out = np.reshape(samples_out, [request_batch, part2_field_num * embedding_size])
print("r_batch", request_batch)
return [common_out, samples_out]
def gen_onnx(feature_size, embedding_size, field_num, part1_fields, part2_fields):
graph_nodes = []
feature_weight = onnx.helper.make_tensor_value_info(
"feature_weight", onnx.TensorProto.FLOAT, [feature_size, 2, int(embedding_size/2)])
feature_field = onnx.helper.make_tensor_value_info(
"feature_field", onnx.TensorProto.INT32, [feature_size, 2])
feature_index = onnx.helper.make_tensor_value_info(
"feature_index", onnx.TensorProto.INT32, [feature_size])
sparse_fields_concat_op_node = onnx.helper.make_node(
"my_sparse_fields_concat_v2", ["feature_weight", "feature_field", "feature_index"],
["output1", "output2"], name="sparse_fields_concat")
output1 = onnx.helper.make_tensor_value_info(
"output1", onnx.TensorProto.FLOAT, [-1, -1])
output2 = onnx.helper.make_tensor_value_info(
"output2", onnx.TensorProto.FLOAT, [-1, -1])
attr1 = onnx.helper.make_attribute("fw_field_num", field_num)
attr2 = onnx.helper.make_attribute("part1_fields", part1_fields)
attr3 = onnx.helper.make_attribute("part2_fields", part2_fields)
sparse_fields_concat_op_node.attribute.append(attr1)
sparse_fields_concat_op_node.attribute.append(attr2)
sparse_fields_concat_op_node.attribute.append(attr3)
graph_nodes.append(sparse_fields_concat_op_node)
graph_inputs = [feature_weight, feature_field, feature_index]
graph_inits = []
graph_outputs = [output1, output2]
graph = onnx.helper.make_graph(graph_nodes, "sparse_fields_concat",
graph_inputs, graph_outputs, graph_inits)
onnx_model = onnx.helper.make_model(graph)
onnx_model.opset_import[0].version = 13
onnx.save(onnx_model, GEN_ONNX)
def onnxrt_compute(input_datas):
cpu_outputs = []
sess_options = onnxruntime.SessionOptions()
sess_options.intra_op_num_threads = 1
sess_options.inter_op_num_threads = 1
sess_options.graph_optimization_level = onnxruntime.GraphOptimizationLevel.ORT_DISABLE_ALL
sess = onnxruntime.InferenceSession(GEN_ONNX, sess_options)
cpu_inputs = {}
for i in range(len(input_datas)):
cpu_inputs[sess.get_inputs()[i].name] = input_datas[i]
cpu_outputs = sess.run(None, cpu_inputs)
return cpu_outputs
def gcu_compile(max_feature_size, max_batch_size,
part1_field_num, part2_field_num, embedding_size):
with TopsInference.device(0, 0):
model_parser = TopsInference.create_parser(TopsInference.ONNX_MODEL)
last_dim_size = int(embedding_size/2)
model_parser.set_input_shapes([[-1,2,last_dim_size],[-1, 2],[-1]])
#model_parser.set_input_shapes([[-1,2,-1],[-1, 2],[-1]])
model_parser.set_input_names(["feature_weight", "feature_field", "feature_index"])
model_parser.set_input_dtypes([TopsInference.DT_FLOAT32, TopsInference.DT_INT32, TopsInference.DT_INT32])
model_parser.set_output_names(["output1", "output2"])
model_parser.set_output_dtypes([TopsInference.DT_FLOAT32, TopsInference.DT_FLOAT32])
optimizer = TopsInference.create_optimizer()
max_shape_dict={}
min_shape_dict={}
max_shape_setting=[]
min_shape_setting=[]
max_shape_dict["main"] = [[max_feature_size, 2, int(embedding_size / 2)], [max_feature_size, 2], [max_feature_size]]
min_shape_dict["main"] = [[0, 2, int(embedding_size / 2)], [0,2], [1]]
max_shape_setting.append(max_shape_dict)
min_shape_setting.append(min_shape_dict)
optimizer.set_max_shape_range(max_shape_setting)
optimizer.set_min_shape_range(min_shape_setting)
network_cpu = model_parser.read(GEN_ONNX)
#optimizer.set_build_flag(TopsInference.KFP16_MIX)
engine = optimizer.build(network_cpu)
engine.save_executable(BIN_FILE_NAME)
def gcu_compute(input_datas):
gcu_outputs = []
TopsInference.create_error_manager()
with TopsInference.device(0, 0):
engine = TopsInference.load(BIN_FILE_NAME)
engine.runV2(input_datas, output_list=gcu_outputs)
return gcu_outputs
def single_test(weight_data, index_data, field_data, part1_fields_data, part2_fields_data, request_batch_size, field_num,\
out1_data, out2_data, weight_shape, field_shape, out1_shape, out2_shape):
weight = np.array(weight_data).astype(np.float32)
weight = np.reshape(weight, weight_shape)
field = np.array(field_data).astype(np.int32)
field = np.reshape(field, field_shape)
index = np.array(index_data).astype(np.int32)
part1_fields = np.array(part1_fields_data).astype(np.int32)
part2_fields = np.array(part2_fields_data).astype(np.int32)
out1 = np.array(out1_data).astype(np.float32)
out1 = np.reshape(out1, out1_shape)
out2 = np.array(out2_data).astype(np.float32)
out2 = np.reshape(out2, out2_shape)
feature_size = weight_shape[0]
max_feature_size = 65536
max_batch_size = 1024
embedding_size = weight_shape[1] * weight_shape[2]
gen_onnx(feature_size, embedding_size, field_num, part1_fields, part2_fields)
golden_inputs = [weight, field, index]
part1_field_num = part1_fields.size
part2_field_num = part2_fields.size
cpu_outputs = get_golden_outputs(golden_inputs, field_num, part1_field_num, part2_field_num, part1_fields, part2_fields)
gcu_compile(max_feature_size, max_batch_size, part1_field_num, part2_field_num, embedding_size)
gcu_outputs = gcu_compute(golden_inputs)
@build_and_clean
def run_test():
part1_fields = []
part2_fields = [2, 4, 5]
field_num = 5
weight_data = [10.0, 21.1, 32.2, 43.3, 54.4, 65.5, 76.6, 87.7,\
-6.1, -5.2, -4.3, -3.2, -2.1, -1.0, -0.9, -0.345,\
34.12, 45.67, 12.03, 23.78, 94.56, 0.6543, 0.1234, 5.28,\
0.1, 0.2, 0.3, 0.4, -4.1, -4.2, -4.3, -4.4,\
0.5, 0.6, 0.7, 0.8, 0.1, 0.2, 0.3, 0.4,\
-0.9, -1.0, -1.1, -1.2, 0.5, 0.6, 0.7, 0.8,\
1.3, 1.4, 1.5, 1.6, -0.9, -1.0, -1.1, -1.2,\
1.7, 1.8, 1.9, 2.0, 1.3, 1.4, 1.5, 1.6,\
2.1, 2.2, 2.3, 2.4, 1.7, 1.8, 1.9, 2.0,\
-2.5, -2.6, -2.7, -2.8, 2.1, 2.2, 2.3, 2.4,\
2.9, 3.0, 3.1, 3.2, -2.5, -2.6, -2.7, -2.8,\
-4.1, -4.2, -4.3, -4.4, 3.7, 3.8, 3.9, 4.0]
weight_shape = [12, 2, 4]
field_data = [1, 1, 1, 1, 1, 3,\
2, 2, 2, 2, 2, 4,\
2, 2, 2, 2, 2, 2, 2, 4, 2, 5,\
2, 5]
field_shape = [12, 2]
index_data = [-1, -1, -1,0, 0, 0,1, 1, 1, 1, 1,3,5]
batch_size = 5
out1_shape = [5,0]
out2_shape = [5,24]
out1_data = []
out2_data =[[0.3,0.4,0.5,0.6,-2,-1.99999988,-2,-2,-0.9,-1,
-1.1,-1.2,0.5,0.6,0.7,0.8,0,0,0,0,0,0,0,0],\
[1.7,1.8,1.9,2,0.7,0.73333329,0.766666651,0.800000072,
-2.5,-2.6,-2.7,-2.8,2.1,2.2,2.3,2.4,2.9,
3,3.1,3.2,-2.5,-2.6,-2.7,-2.8],\
[0,0,0,0,0,0,0,0,0,0,0,0,0,0,0,0,
0,0,0,0,0,0,0,0],\
[0,0,0,0,0,0,0,0,0,0,0,0,0,0,0,0,
-4.1,-4.2,-4.3,-4.4,3.7,3.8,3.9,4],\
[0,0,0,0,0,0,0,0,0,0,0,0,0,0,0,0,
0,0,0,0,0,0,0,0]]
single_test(weight_data, index_data, field_data, part1_fields, part2_fields, batch_size, field_num,\
out1_data, out2_data, weight_shape, field_shape, out1_shape, out2_shape)
if __name__ == '__main__':
run_test()
4.13. 性能评估¶
用来计算gcu kernel,stream callback,H2D/D2D/D2H的执行时间。
Tool¶
模型类型格式为onnx/engine file,可以使用topsexec 来测试模型级别性能。
示例:
topsexec --loadExecutable=resnet50.exec --iterations=1002 --fp16 --threads=1 --streams=12 --cluster=-1
Attention
topsexec 工具在你安装topsInference时,会默认安装上,关于更多topsexec的信息,可以使用 topsexec –help 查看
CPU Timing¶
通过使用c++11特性,通过统计cpu 前后计算runV3执行时间,缺点:时间不准确,这里面的时间包含了系统调度时间。
示例:
#include<chrono>
topsStream_t stream;
topsStreamCreate(&stream);
...
auto startTime = std::chrono::high_resolution_clock::now();
engine->runV3(input_tensor_list.data(), output_tensor_list.data(), stream);
topsStreamSynchronize(stream);
auto endTime = std::chrono::high_resolution_clock::now();
float totalTime = std::chrono::duration(endTime - startTime).count();
topsStreamDestroy(stream);
GCU Event¶
这种方案,比上一种方案,提供了更细微的粒度,通过前后event来获得Stream执行时间,可以获取到sip kernel的执行时间。
示例:
topsStream_t stream;
topsStreamCreate(&stream);
topsEvent_t start, end;
topsEventCreate(&start);
topsEventCreate(&end);
...
topsEventRecord(start, stream);
engine->runV3(input_tensor_list.data(), output_tensor_list.data(), stream);
topsEventRecord(end, stream);
topsStreamSynchronize(stream);
float totalTime;
topsEventElapsedTime(&totalTime, start, end);
topsStreamDestroy(stream);
topsEventDestroy(start);
topsEventDestroy(end);
GCU Profiling¶
更全面的profile,可以看到op(operator 算子)/DMA copy的执行时间,以及整个模型作为kernel的执行时间。通常推荐选用此方式。 想了解topsprof更多,可以参考topsprof使用说明 性能分析工具。
topsprof --force-overwrite --debug --print-app-log --enable-activities "/General/Task Pipeline|Api Trace//*" --export-visual-profiler ./topsprof-result python3 -m pytest -k xxx.py[xxx]
5. 调试方法及常见问题¶
TopsInference为用户提供了许多种调试策略,报错信息管理可以帮助用户快速定位问题,性能分析工具能够方便用户获取时间线视图,分析和识别热点。
5.1. 报错信息管理¶
TopsInference提供IErrorManager作为错误信息管理类,在环境配置或参数配置错误时会抛出TIFStatus异常,用户可以跟进错误提示对模型进行调整,典型异常TIFStatus如下:
TIF_INVALID_PARAM: 不合法的参数。
TIF_INVALID_VALUE: 值为nullptr或0。
TIF_MIS_MATCHED_DATA_TYPE: 数据类型不匹配。
TIF_PARSER_UNSUPPORTED_MODEL: 模型在现有解析器还未支持。
TIF_UN_SUPPORTED_DATA_TYPE: 不支持的数据类型。
TIF_COMPILE_PARSE_FAILED: 不支持的模型结构。
TIF_NOT_FOUND: 列表中未找到关键字或名称。
TIF_LOAD_NON_EXIST_FILE: 加载文件不存在。
TIF_SAVE_NON_EXIST_FILE: 报错文件不存在。
TIF_EXECUTABLE_DEVICE_VERIFY_FAILED: 执行设备验证失败。
TIF_UN_SUPPORTED_OPERATOR: 操作算子不支持。
TIF_MIS_MATCHED_SHAPE_OR_DIM: 形状尺寸不匹配。
TIFStatus所有异常状态详情可以参考《TopsInference C++ API手册》。
5.2. 性能分析工具¶
燧原科技提供了性能分析工具topsprof和可视化分析软件TopsVisualProfiler,topsprof分析工具能够从命令行收集和查看分析数据。topsprof支持在CPU和GCU上收集与TopsInference相关的活动的时间线,包括内核执行、内存传输、内存集和内核的事件或指标。分析选项通过命令行选项提供给topsprof。收集分析数据后,分析结果显示在控制台中,也可以保存以供topsprof或TopsVisualProfiler以后查看。
topsprof使用方法为“topsprof [options] [application] [application-arguments]”。
options需要以–开头,示例“–print-api-summary”。
topsprof统计模式是topsprof的默认模式。在此模式,topsprof对每一个算子或API输出一行结果。对每一行结果topsprof会输出最大值,最小值,平均值,调用信息等统计参数。
topsprof --enable-activities "*/general/operator" python3 ./r50.py
topsprof跟踪模式提供一个时间轴显示发生的活动和持续时间,每次调用会显示为一行。
topsprof --print-dtu-trace --enable-activities "*/general/operator" python3 ./r50.py
选项–export-profile可以用来生成结果文件。选项–export-visual-profiler可以用来供visual-profiler使用的结果文件。可以用visual-profiler打开,也可以使用–import-profile选项导入生成的vpd文件。
运行生成结果文件后可以使用TopsVisualProfiler打开查看可视化时间线视图和相关报告。
topsprof使用细节见《topsprof用户手册》,TopsVisualProfiler使用细节见《TopsVisualProfiler用户手册》。
5.3. 常见问题¶
运行过程中出现报错,如何处理¶
查看报错提示,跟据TIFStatus判断错误类型,并修改代码或模型参数。
比如动态形状时出现“error log: [0] TIF_INVALID_PARAM(7) engine input less than minshape”的错误,此时根据log信息检查实际运行的输入形状是否小于设置的最小形状。
TopsInference有哪些优化技术¶
TopsInference使用大量汇编级别的优化,将模型重构为高效指令,编译器和硬件深度结合,使用算子融合技术,多精度混合计算,支持多batch及动态形状模型推理。
如何查看GCU的使用情况¶
在shell中使用efsmi命令可以查看GCU卡当前的监控状态,输出结果如下所示:
-----------------------------------------------------------------------
----------------- Enflame System Management Interface -----------------
-------- Enflame Tech, All Rights Reserved. 2022 Copyright (C) --------
-----------------------------------------------------------------------
*Thu Feb 16 11:30:20 2023-------------------------------------------------*
*EFSMI V1.13.2 Driver Ver: 2.2.20230213-29-g404ed8888d9 *
*-------------------------------------------------------------------------*
*-------------------------------------------------------------------------*
* DEV NAME | FW VER | BUS-ID ECC *
* TEMP DPM Pwr(Usage/Cap)| Mem vGCU| DEV-Util UUID *
*-------------------------------------------------------------------------*
* 0 T10 | 1.47.1 | 00:01:0.0 Disable *
* 62C 16 45.0W 225W | 16G Disable| 0.0% U260031207081 *
*-------------------------------------------------------------------------*
动态形状的含义是什么¶
动态形状指的是模型中张量形状不是固定的,而是根据运行时的输入决定的。在TopsInference中包含输入形状或输出形状是不确定的,类似nms算子都属于动态形状范围内。
用户需要使用runV2接口进行动态形状的推理,run_with_batch接口是应用在多batch输入的静态形状场景下,不支持动态形状。